Ordinal Scale: Definition, Characteristics, and Benefits
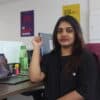
Vismaya Babu
Last Updated: 5 September 2024
11 min read
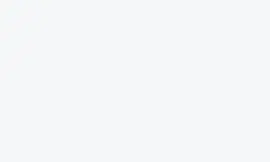
Let’s take a 100-meter sprint. After the race is done, you will have a ranking of all the athletes who participated in it. It will tell you who got the first, second, and so on and so forth. But it won’t tell us by how much distance the first athlete won, or the fourth horse lost the third place.
The ordinal scale is one of the ways in which you take qualitative data and associate them with a numerical value. It allows you to assign order or rank the responses in your survey. When it comes to the realm of survey and market research, data collection is the core of the system.
Associating responses with numerical value makes it incredibly easy to analyze, & deliver rich insights.
In this blog, we’ll learn about the ordinal scale, its scope, how to measure ordinal data, and discuss a few examples.
What is the Ordinal Scale?
Ordinal meaning ‘order’ is a classification system that ranks data in an order or sequence without actually establishing the degree of variation between them.
And when used in surveys, an ordinal scale enables you to study a respondent’s attitude toward a subject, such as satisfaction or preference, using a series of ordered replies.
Ordinal scales can be used to identify and describe the magnitude of variables by grouping, ranking, or ordering them. Let’s explore the details.
Using different types of survey scales in your surveys can bring value to the insights you gather. At the same time, having the backup of the right survey tool is crucial. Perhaps SurveySparrow can help! The drag-and-drop builder is so easy to use. Now, if you feel a bit unmotivated, hit a prompt and let the AI builder craft your survey in seconds.
Why don't you try it out?
Characteristics of Ordinal Scale
Determining value
Let’s take one of the most popular survey questions: “How satisfied are you with our product?”. Ideally, the respondent can choose their answer from "Very satisfied," "Satisfied," "Neutral," and "Dissatisfied."
Assess the distribution of responses on the ordinal scale to determine whether your overall customer satisfaction rates are high, somewhere in between, or low. The insights will help you make data-driven decisions to enhance customer experience.
Natural order
To explain this, let’s take the survey question, “How likely are you to recommend our product to a friend or colleague?”. The response options are: "Extremely Likely," "Very Likely," "Likely," "Neither Likely nor Unlikely," "Unlikely," and "Very Unlikely."
The ordinal scale, arranged from most likely to recommend to least likely to recommend in a natural order, makes it easier for the respondents to express their level of intent to recommend your product.
Ranking variables
To elaborate, let’s take a survey question as an example: “How often do you use our online platform?” The response options are "Daily,” "Weekly,” "Monthly,” "Rarely,” and "Never.”
By ranking the responses from highest to lowest usage, the ordinal scale will help you assess the overall engagement, identify opportunities for targeted marketing, or verify areas of improvement.
Measuring non-numerical variables
To elaborate, let's take a critical question from employee engagement surveys: How often do you feel stressed at work? The employee can choose "Very Frequently," "Sometimes", "Rarely", or "Never" to answer this question.
Using an ordinal scale, the survey question measures the non-numerical variable, "work stress". The scale allows you to quantify non-numerical characteristics such as happiness, pleasure, and more.
The analysis of the responses will help you understand the distribution of stress levels amongst your employees, identify the influencing variables and gain insights to prevent employee burnout.
Assessing agreement
To clarify, let's consider the survey question: Please rank the following in order of importance when selecting a new smartphone:
- Price
- Brand Reputation
- Features
- Battery Life
- Design
Here, the respondents are asked to rank selected factors from most important(1) to least important(5). The analysis of the response distribution would help you study the degree of agreement present amongst your respondents.
So, if the majority of respondents ranked "Price" as the most important factor, it suggests a strong agreement among your respondents on this variable's influence.
From understanding consumer behavior to product development and choosing the right marketing strategy, ordinal scales can help your business move in the right direction.
Relative comparison
Similar to a Likert scale, an ordinal scale helps you assess the relative rank of variables and quantify their values. From usage patterns to perceived product quality and satisfaction to experiences, the scale will help you make smarter decisions.
Advantages of Ordinal Scale
Compare variables
Easy to use & understand, an ordinal scale makes it simple to run relative comparisons of different variables in your survey. From attitudes and satisfaction to experiences and preferences, the scale will help you measure, analyze, and improve influential factors.
Grouping
The ordinal scale helps you learn the relative positions of different variables by ranking or ordering your survey data. For instance, let's recall the customer satisfaction survey question, "How satisfied are you with our product?"
The ordinal scale categorizes your customers based on their responses to help you identify the happy customers, passives, and those at risk of churn. The categorization makes it easier for you to deliver tailored engagement to the appropriate category.
Easy inferences
Be it the degree of agreement or describing the magnitude of a variable, the ordinal scale simplifies the analysis and categorization of variables. The scale makes it easy to compare variables and turn survey data into actionable insights for your business.
Richer insights
The ordinal scale insights are considered more informative than other levels of measurement, like a nominal scale, because it uses a linear rating system. Using the ranking system, this method can provide a better understanding of the magnitude of the variable being measured.
Versatility
The ordinal scales are flexible, and their adaptability deems the scale suitable for surveys, polls, and questionnaires of all kinds. Since the scale can measure even non-numeric characteristics, it can be used in both quantitative and qualitative research.
Ordinal Scale vs Other Levels of Measurement
In the realm of statistics, variables or numbers are identified, defined, and categorized using different types of measurement scales. The data measured is classified into quantitative and qualitative data.
There are four different scales of measurement. Like the ordinal scale, each level of measurement scale has specific properties that determine its use of statistical analysis.
Nominal scale vs. Ordinal scale
Also called the categorical variable scale, the nominal scale labels variables into classifications without assigning them a quantitative value or order.
The numbers associated with variables serve as tags to classify or identify the objects and deal with the non-numeric variables that do not have any value.
Whereas the ordinal scale, the analysis goes beyond naming the categories. The data is categorized in a natural order, though the difference between them may not be equal.
For example, let's take the survey question, "What is your favorite movie genre?"
For the researcher, only the genre names are significant and don't need any specific order for these genres.
Interval scale vs. Ordinal scale
Known as the numerical scale and considered the third level of measurement (the first & second levels of measurements being nominal scale and ordinal scale, respectively), the interval scale can be defined as a quantitative measurement scale, where the order of variables and the difference between these variables are known and meaningful.
Unlike the ordinal scale, the variables involved in the interval scale are measured exactly; the difference between them is equal, with no true zero point.
For example, let's take the survey question, "What is the temperature in your city?", to explain this further. Measured in Celsius or Fahrenheit temperature scales, the values are already defined, constant, and measurable.
It's established that 100 degrees is always higher than 60 degrees, and the difference between these two variables is the same as 90 degrees and 50 degrees. But, we cannot say that zero degrees is the true zero point in the temperature scale because negative temperature exists.
In simpler words, the interval scale lets you quantify the difference between two variables.
Ratio Scale vs. Ordinal Scale
The 4th level of measurement, the ratio scale, is defined as the variable measurement scale and allows the researchers to compare the differences or intervals between two variables.
The scale can be used in your surveys to collect data that can be ranked in order, has equal distances between identified categories, and also has a true zero point. This means that the scale will value the variable at zero if there is no measured quantity.
Because it can establish the absolute value of zero, the ratio scale is used in market research to measure market share, determine the number of customers, or decide the price of an upcoming product.
To explain further, let's consider a survey question about the respondent's income. Measured in currency, income can have a true zero value(zero income) and have an equal distance between categories (intervals between units in currency).
How to Analyze Ordinal Scale Data
The categories of data generated from an ordinal scale lack the scope to represent equal increments of the underlying attribute. It records the higher and lower values of different variables in a set.
You can analyze ordinal scale data with two methods: inferential statistics and descriptive statistics. Inferential statistics will let you form conclusions about a population based on a sample.
Descriptive statistics for ordinal scale
This method is used when you want to summarize your data set. The common descriptive statistics for ordinal data include:
- The frequency distribution is a table that explains your ordinal data in terms of numbers and percentiles. For a customer satisfaction survey, the frequency distribution would help you identify which response options, such as "Very satisfied" or "not satisfied," were chosen by the respondents. It could also help you assess how the values are spread across the categories as well.
- Visualize the data with a bar graph that provides a clearer picture of the frequency distribution found in the responses.
- Central tendency is where the majority of the values in a dataset are located. The ordinal scale will help you identify the mode, the most frequent category, and the median, the middle value when the data is ranked. It's important to note that since the ordinal scale lacks a number component, it is impossible to calculate the mean.
- Since ordinal data doesn't have true numerical values, the measures of variability are considered less precise than those used for interval or ratio scales. One common technique used to measure variability is determining the dataset's range, maximum, and minimum values.
- Likewise, you can also use the Interquartile Range (IQR), Coefficient of Variation (CV), or Gini Coefficient to assess data variability.
Suppose you have a survey question asking the customers to rate their satisfaction with your product on a scale of 1-5 (where 1 = Very Dissatisfied to 5 = Very Satisfied).
With descriptive statistics, you can calculate the frequency of each response category, the median satisfaction level, and the percentage of respondents who are satisfied or very satisfied. Or, with inferential statistics, you may compare the satisfaction levels of two different groups like, men and women.
It's also important to consider the limitations of ordinal scales while analyzing data, such as the unequal distance between categories. More than the parametric tests, it's better to rely on non-parametric tests as they make fewer assumptions about the distribution of the data.
The best part about having a SurveySparrow at your disposal is that you can rely on it from start to finish. With an executive dashboard and advanced analytics features such as sentiment analysis, individual response editing, report summaries, and an AI-powered text analytics tool, you get everything you need all in one place.
14-day free trial • Cancel Anytime • No Credit Card Required • No Strings Attached
Ordinal Scale: Do You Need It?
Unlike interval or ratio scale data, you cannot alter ordinal scale data by mathematical operators. Easy to create, & easier to interpret, micro surveys created with ordinal scale questions would be the best data collection tool you could try.
The ordinal scale will help you make better decisions, from measuring customer satisfaction to assessing employee engagement and improving the product experience you deliver.
PS: if you're more into creating your survey from scratch like me, do your research, define the variables you want to, & learn how to write your survey questions before you finalize the survey design.
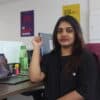
Vismaya Babu
Lead SEO specialist at SurveySparrow
Marketing whiz and tech lover, always exploring the digital world with curiosity and creativity!
Turn every feedback into a growth opportunity
14-day free trial • Cancel Anytime • No Credit Card Required • Need a Demo?