Knowledge
Statistical Significance: Definition, Calculation, Importance
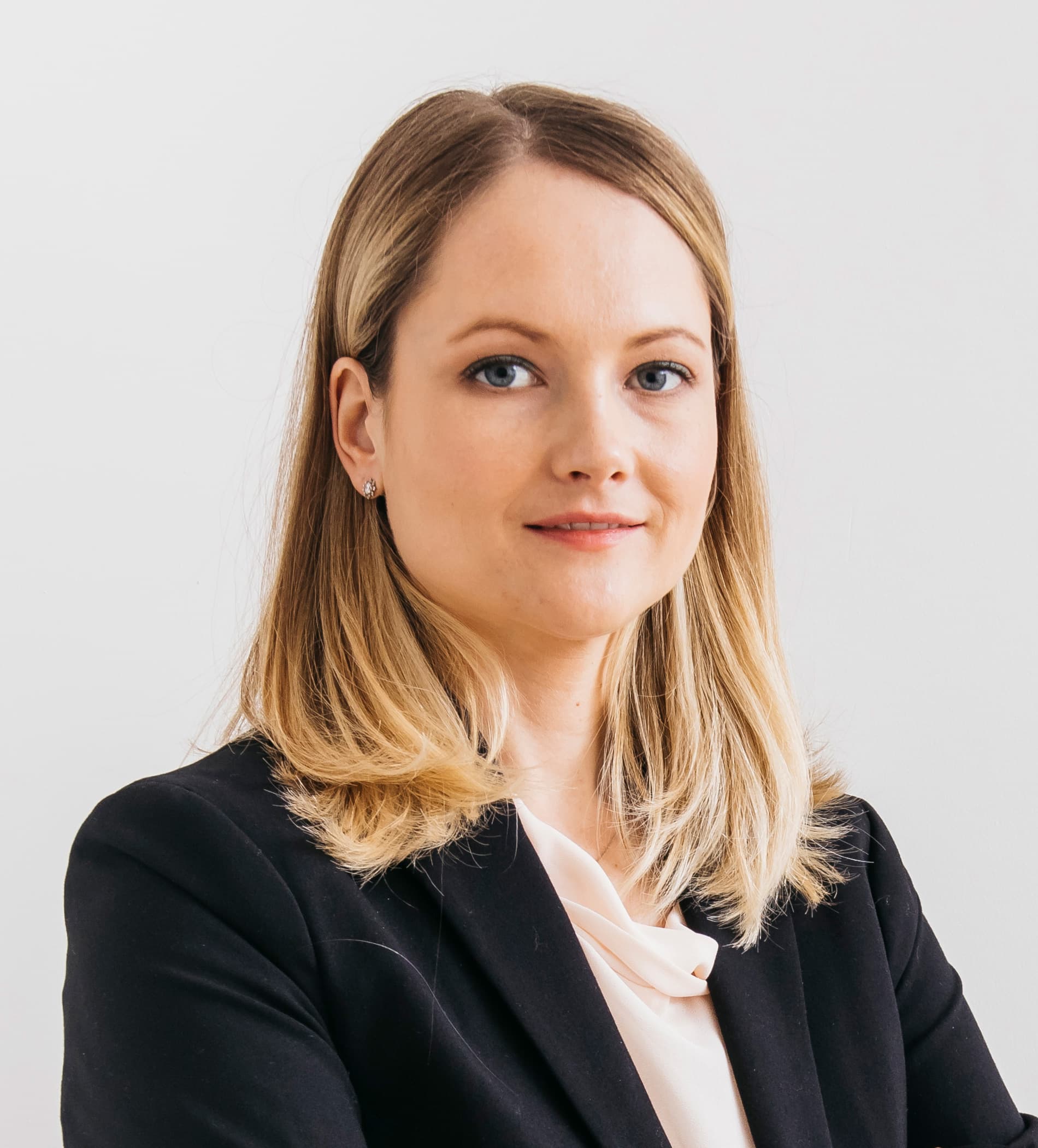
Article written by Kate Williams
Content Marketer at SurveySparrow
12 min read
14 November 2023
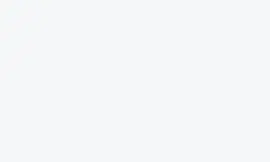
What separates a genuine discovery from mere chance? Statisticians agree that in data analysis, statistical significance holds a prime position. It is essential to know all about it if you wish to draw accurate conclusions. So, what does it mean, and how is it calculated?
As we know, it can get messy if you do not understand the terms associated with it. So, why not start with the basics? I have included the statistical jargon used in this blog.
Basic Terms and Definitions
Statistical Significance:
- It helps us know if our findings are authentic or just random chance.
- We calculate it using a p-value, usually less than or equal to 0.05 for significance.
P-Value:
- The p-value shows us if our results have a meaning.
- Also, a small p-value (below 0.05) means strong evidence supports our findings.
Null-Hypothesis:
- This hypothesis suggests there’s no difference between groups or conditions.
- Above all, researchers test it to find natural patterns and differences in the data.
Statistical Significance Calculator:
- It’s a user-friendly tool that simplifies complex calculations. The calculator gives researchers the p-value without needing advanced math.
- Researchers use it to quickly analyze their data, making the process efficient and accurate.
Effect Size:
- Effect size measures the real impact of our results.
- It helps us understand the practical significance of our findings beyond just statistical significance.
Confidence Interval:
- A confidence interval gives us a range indicating the uncertainty of our results.
- It shows the probable range within which our result lies, offering a clearer picture of the data.
Type I Error and Type II Error:
- Type I is a false positive, while Type II is a false negative.
- Striking a balance between these errors is essential for accurate conclusions in research.
Alpha Level (α)
- The alpha level (usually set at 0.05) is the threshold for significance in hypothesis testing.
- Choosing an appropriate alpha level is vital. It influences our confidence in research outcomes.
Critical Region:
- The critical region shows the area where results are considered significant.
- If our results fall inside this region, they are meaningful, guiding us toward valuable conclusions.
What is a Hypothesis?
A hypothesis is like an educated guess in science!
As mentioned above, it’s a clear and specific statement that predicts what might happen in an experiment or study. Scientists use hypotheses to guide their research and predict the outcomes they expect to see.
Components of a Hypothesis
Statement: A hypothesis is a concise statement that explains a relationship between variables. It’s usually based on prior knowledge, observations, or existing theories.
Testable: A reasonable hypothesis must be testable. We must be able to get to the “right” or “wrong” through experiments or observations. Scientists need to design experiments that can either support or refute the hypothesis.
Precise Prediction: A hypothesis includes a clear prediction about the experiment’s outcome. It specifies what the researcher expects to observe if the hypothesis is correct.
Example
Let’s say a scientist is curious about whether plants grow better with sunlight. Their hypothesis could be: “Plants exposed to sunlight will grow taller than plants kept in the dark.” This statement is specific, testable, and predicts that sunlight positively affects plant growth.
Importance of Hypotheses
- Guiding Research: Hypotheses provide a roadmap for scientific investigations. They help researchers focus their experiments, ensuring they collect relevant data to support or refute the hypothesis.
- Predicting Outcomes: By making predictions, hypotheses allow scientists to anticipate what they might find. This foresight helps in interpreting results and drawing meaningful conclusions.
- Advancing Knowledge: Testing hypotheses contributes to the accumulation of scientific knowledge. Whether a hypothesis is confirmed or rejected, the results provide valuable insights, leading to a deeper understanding of the natural world.
What is a Null Hypothesis?
A null hypothesis is the opposite of the main guess in a scientific experiment. It’s a statement suggesting that there is no significant difference or effect between groups or conditions.
In simpler terms, it represents the idea that any observed differences are just due to chance and not because of a real relationship or effect.
Components of a Null Hypothesis
Neutral Statement: The null hypothesis is a neutral and straightforward statement. It doesn’t predict a specific outcome but asserts that there is no difference.
Comparison Basis: It serves as a benchmark for comparison. Scientists test their experimental results against the null hypothesis to see if there is enough evidence to reject it in favor of their hypothesis.
Example
In the case of the plant growth experiment, the null hypothesis could be: “There is no significant difference in the height of plants grown in sunlight compared to plants grown in the dark.” This statement implies that any difference in plant height observed between the two groups is merely coincidental and not because of sunlight.
Importance of Null-Hypotheses:
- Critical Comparison: By comparing experimental results to the null hypothesis, scientists determine if their findings are statistically significant. If the results significantly differ from what the null hypothesis predicts, it suggests a meaningful relationship.
- Avoiding Bias: Having a null hypothesis prevents bias in interpreting results. Researchers remain open to the possibility of no effect, ensuring objective analysis.
- Scientific Rigor: Including a null hypothesis in experiments adds rigor to scientific investigations. It sets a standard that results must surpass to be considered genuinely significant.
Okay, now let’s get to business!
What is Statistical Significance?
Statistical significance helps scientists trust their findings!
By definition, being statistically significant means that the results of a study are probably actual, not random.
When the p-value is low (usually below 0.05), it shows the results are meaningful. It’s like a stamp of approval for research conclusions.
So, what does being statistically significant mean?
Picture this: You’ve conducted an experiment, and the results are astounding. But are they genuine or merely coincidental? To be statistically significant means your findings aren’t a random chance at play. It signifies a meaningful pattern in the data, lending credibility to your research.
Scientists use statistical significance to determine if results are meaningful and not just due to chance. This helps researchers understand if differences or patterns in their data are accurate. It answers the question: “Is what we found in our research genuine, or could it have happened randomly?
Still not clear? Let’s break it down a little further.
Example
Imagine a study comparing two groups: Group A received a new drug, and Group B received a placebo. After analyzing the data, researchers found that patients in Group A had significantly lower cholesterol levels than Group B, with a p-value of 0.02 (less than 0.05).
This indicates that the difference in cholesterol levels between the groups is statistically significant. This suggests that the new drug effectively reduces cholesterol.
Statistical Significance Calculator
Crunching numbers can be a little daunting, right? Well, it doesn’t have to be (given that you have the right guide.)
A statistical significance calculator is a handy tool for researchers. It does the complex math for them! Scientists feed in their data, and the calculator quickly tells them whether the results are significant. It’s user-friendly and saves time, ensuring accurate analysis without the headache of intricate calculations.
Furthermore, a high variance in the population shows a high chance of error and sampling bias.
The formula for statistical significance varies based on the statistical test being used. However, a general procedure for many tests involves calculating a test statistic (like t or z) and comparing it to a critical value from a statistical table or using the software.
Test Statistic = Observed Value−Expected Value/ Standard Error
In this formula, the test statistic represents how many standard deviations the observed value is from the expected value. The smaller the test statistic, the more likely the results are statistically significant. Researchers compare this statistic with critical importance to determine significance.
Importance of Statistical Significance
- Validates Research Findings: Statistical significance confirms that the observed results are not due to chance, adding credibility to the study’s outcomes.
- Informs Informed Decision-Making: It provides a basis for making informed decisions in various fields, guiding policies, strategies, and interventions.
- Supports Research Conclusions: When results are statistically significant, they keep the conclusions drawn from the study, making them more robust and reliable.
- Facilitates Accurate Comparisons: Statistical significance allows researchers to accurately compare different groups, treatments, or conditions, enabling meaningful comparisons.
- Optimizes Resource Utilization: By focusing efforts on statistically significant findings, resources such as time, money, and manpower are used efficiently, avoiding waste on less meaningful outcomes.
- Builds Trust in Research: Studies with statistically significant results are more trustworthy, gaining the confidence of peers, stakeholders, and the general public.
Regarding data analysis, advanced tools such as SurveySparrow simplify complex statistical processes. For instance, for data collection, it is the perfect tool that lets you create, share, and collate data. Further, you can analyze and act upon the insights gained. Yes, all on the same platform.
Give it a try!
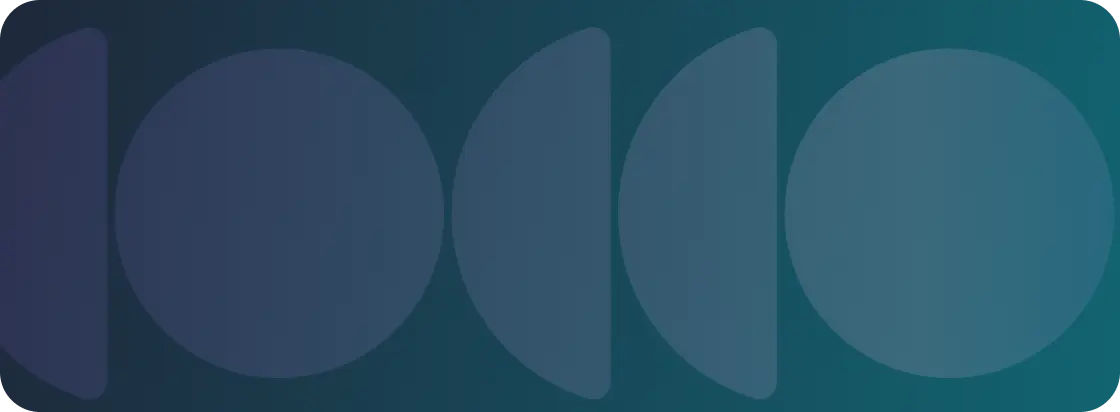
Create conversational surveys with SurveySparrow
A personalized walkthrough by our experts. No strings attached!
How to Determine Statistical Significance
Take a look at this 8-step guide:
1. Formulate Hypotheses
- Clearly state what you’re trying to prove or disprove.
Null Hypothesis (H0): No significant difference.
Alternative Hypothesis (Ha): Expected difference based on research.
2. Select a Significance Level (α)
- Next, choose a small number (often 0.05 or 5%) as a cutoff for significance.
- The results are significant if the p-value (calculated later) is lower than α.
3. Collect Data
- Gather data relevant to your study from experiments or observations.
- Also, ensure data is accurate and representative of the population.
4. Choose a Statistical Test
- Pick the proper test based on your research question and data type.
- For instance, t-test for comparing two groups’ means, chi-square for categorical data, etc.
5. Calculate Test Statistic
- Now, use the chosen test formula to calculate a test statistic (like t, z, F).
- The formula varies for each test. You must refer to statistical resources or software.
6. Find P-Value
- You must have understood by now that the P-value indicates the probability of getting your results by chance.
- Moreover, a lower p-value suggests results are less likely due to chance.
7. Compare P-Value and α
- The results are statistically significant if the p-value is less than α (your chosen significance level).
- It means observed differences are likely, not random.
8. Draw Conclusions
- All things considered, it is vital to check the practical importance of your findings.
- If the results are statistically significant, they support your research hypothesis.
- However, be cautious. Statistical significance doesn’t always mean practical importance.
Limitations of Statistical Significance
- Sensitivity to Sample Size: Big samples can sometimes overemphasize minor differences. This makes them seem more important than they really are.
- Influence of Outliers: Some results might seem more significant or less significant than they indeed are. Why? Because outlying extreme values can mess with our results.
- Cumulative Significance Testing: If we keep testing the exact same data for different things, we increase the risk of finding something significant just by chance.
- Contextual Disregard: Biases and misrepresentations are inevitable at times. These tests often consider the unique context of a situation.
The Role of Statistical Significance in Hypothesis Testing
Statistical significance acts as the verdict!
Accept or Reject Hypotheses: Statistical significance guides researchers to either accept their hypothesis (if the results are significant) or reject it (if not).
Informed Decisions: It ensures decisions are based on solid evidence. This reduces the risk of making conclusions without proper support.
Minimizes Errors: Researchers rely on significance levels to minimize the chance of Type I (false positive) and Type II (false negative) errors in their conclusions.
Scientific Validity: Significance ensures that scientific studies meet rigorous standards. The result? Credibility and reliability of research outcomes.
Guides Further Research: Positive significance motivates further exploration. You get deeper insights and expand the scope of knowledge.
Wrap Up!
As we’ve explored, statistical significance isn’t merely a checkbox to tick. It’s a nuanced understanding of probabilities and outcomes. It separates chance from genuine patterns, helping researchers discern the meaning from the coincidental. However, it’s vital to acknowledge its limitations and interpret results contextually.
So, the next time you encounter a research study or delve into statistical analyses, remember the significance of statistical significance. It’s not just numbers!
And while at it, why not take SurveySparrow for a spin? You can streamline your data collection process.
It’s free to try!
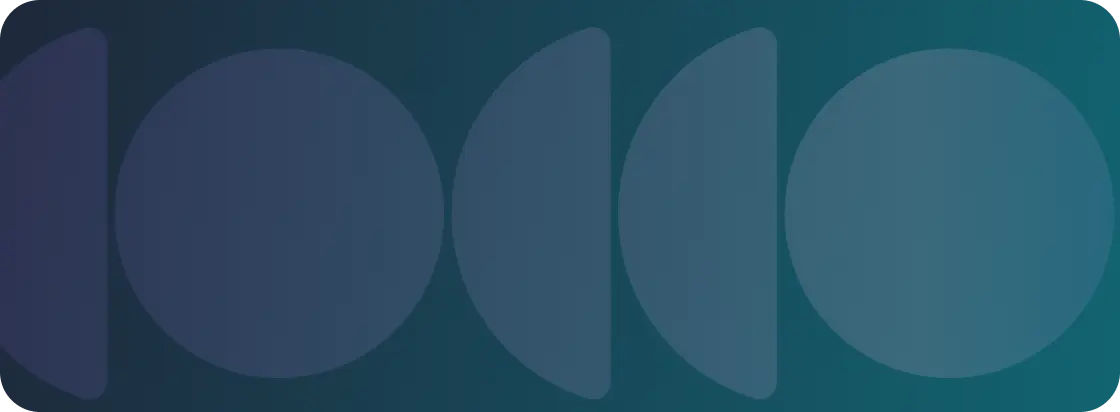
Create conversational surveys with SurveySparrow
A personalized walkthrough by our experts. No strings attached!
Start 14 Days free trial
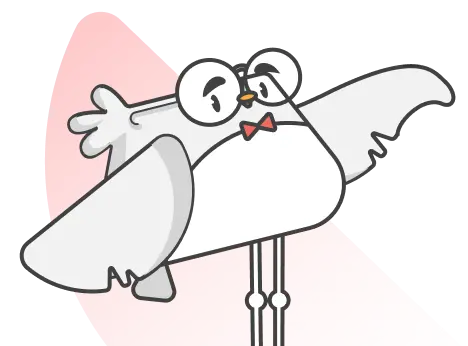
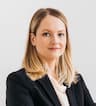
Kate Williams
Related Articles
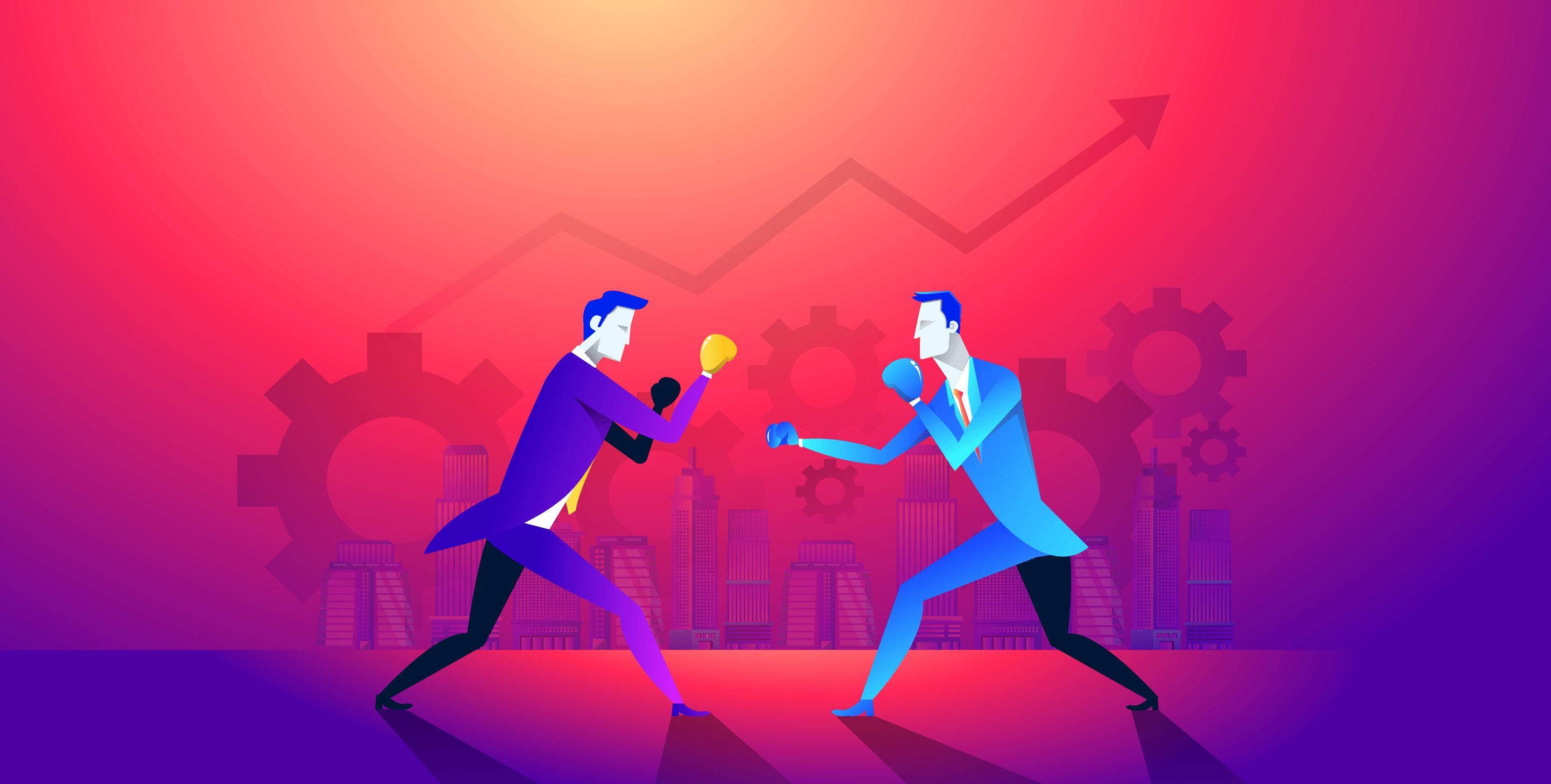
Best Of
Get Ahead of Your Business Competitors by Going Where They Won’t
6 MINUTES
10 April 2020
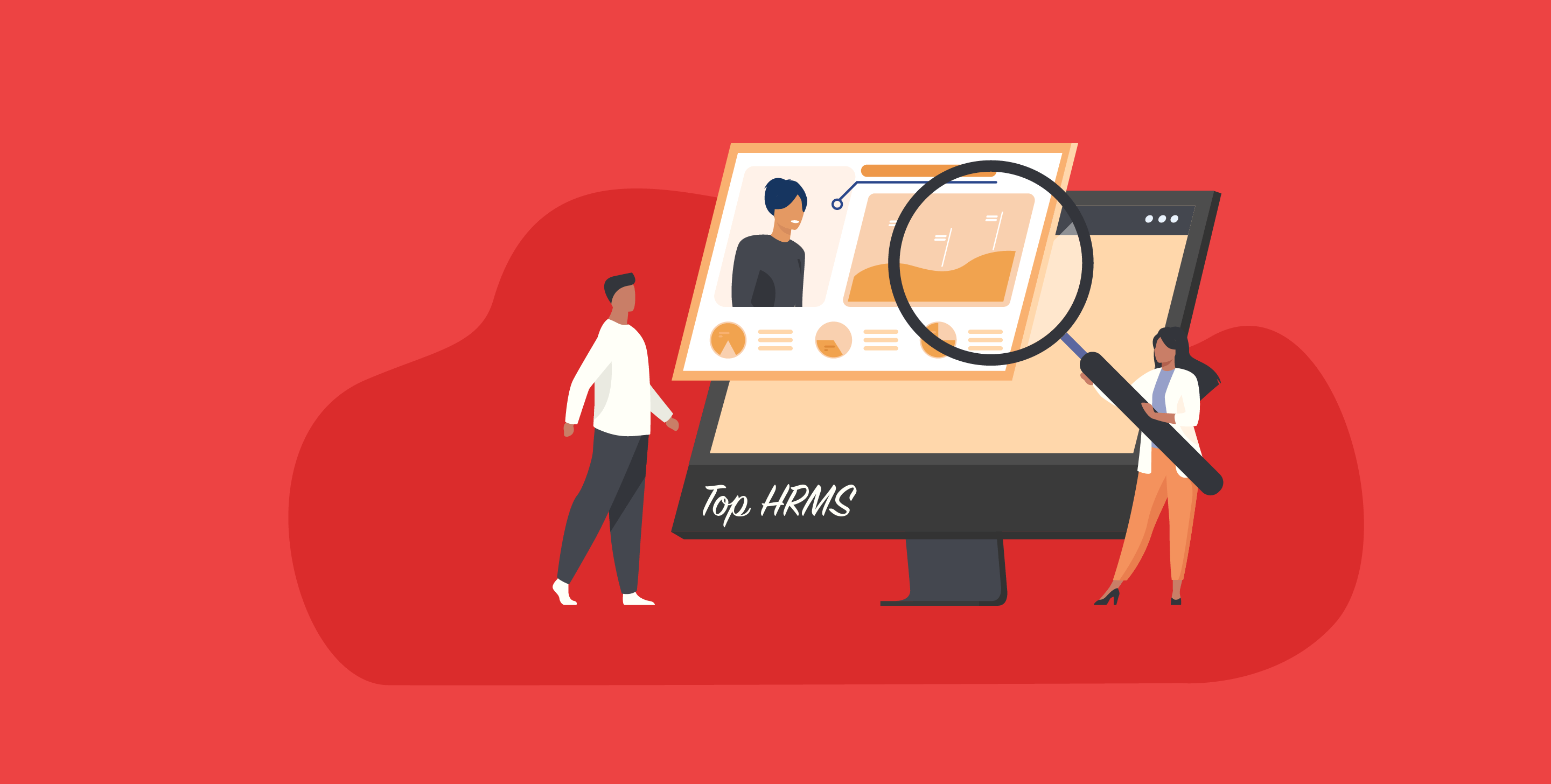
Compare
12 Top HRMS Software: The 2024 Edition
14 MINUTES
26 November 2021
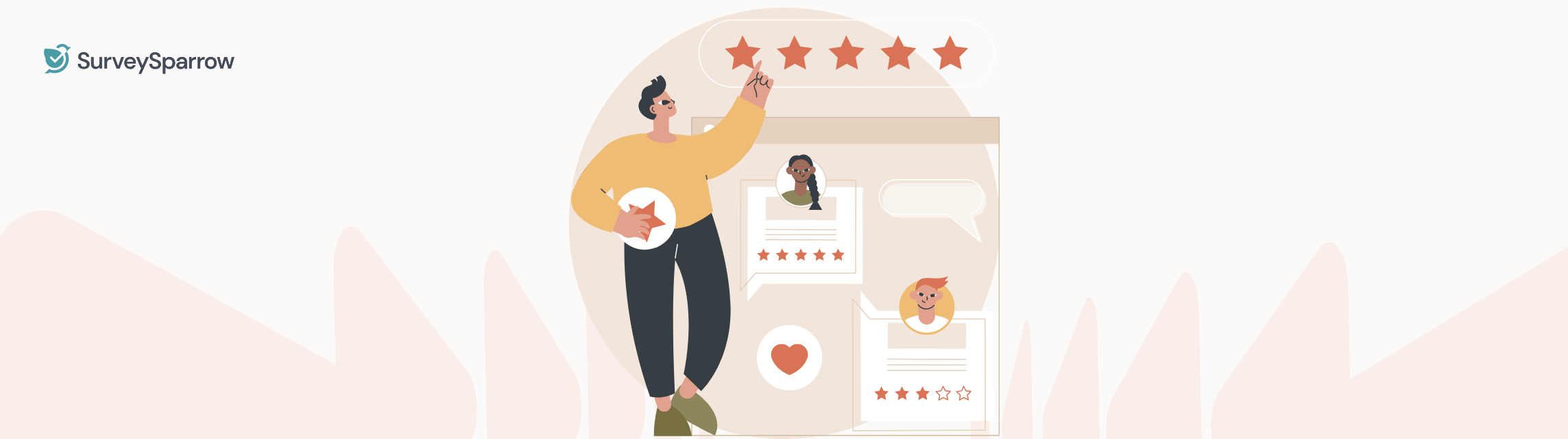
Knowledge
Feedback Button: Benefits,Types, Use Cases and Tools to Use
10 MINUTES
6 August 2024
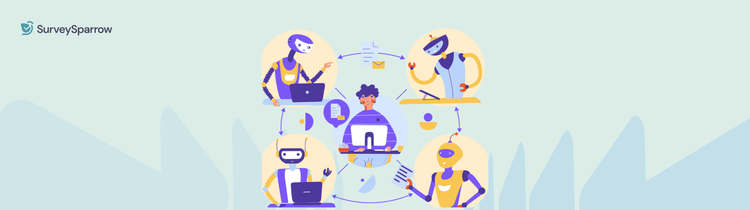
Sales
Top 9 Proposal Automation Tools for Sales Teams in 2025
11 MINUTES
6 February 2025