What is Selection Bias
Identify and address selection bias for accurate and reliable results
Act now to identify and mitigate selection bias
Demo tailored to your business needs
Preview features you're interested in
Smart solutions from our experts
Selection bias is a critical concept in research and data analysis that can significantly impact the validity of study results. This comprehensive guide will delve into what selection bias is, its types, and how to mitigate it, providing practical examples along the way.
What is Selection Bias?
Selection bias refers to the systematic error that occurs when the sample chosen for a study is not representative of the population intended to be analyzed. This bias can lead to inaccurate conclusions because the results are skewed by the unrepresentative sample. Essentially, selection bias occurs when certain groups are overrepresented or underrepresented in the study sample compared to the population.
Selection Bias Definition
The selection bias definition can be summarized as the distortion of statistical analysis results due to the method of collecting samples. When the sample is not randomly selected, or certain groups are excluded, the study’s findings do not accurately reflect the target population.
Define Selection Bias in Simple Terms
“To define selection bias in simple terms: it is a type of error that occurs when the participants or data points selected for a study are not representative of the intended population. This leads to skewed results that do not accurately reflect the true characteristics or behaviors of the entire population.”
Selection Bias Meaning in Research
The selection bias meaning in research is the distortion of statistical analysis results due to the method of collecting samples. This bias can significantly impact the validity of research findings, making it crucial for researchers to identify and address it in their study designs.
Types of Selection Bias
Understanding the different types of selection bias is essential for identifying and mitigating them in research. Here are some common types:
- Self-Selection Bias
- Sample-Selection Bias
- Exclusion Bias
- Survivorship Bias
- Nonresponse Bias
- Self-Selection Bias
Self-Selection Bias
Self-selection bias happens when individuals choose to participate in a group or study on their own, leading to a non-random sample. This type of bias is especially common in surveys and studies where participants volunteer to join. The main problem is that those who decide to take part may have specific characteristics or opinions that are significantly different from those who do not. This difference can seriously distort the results, making it challenging to draw accurate conclusions about the broader population.
In essence, self-selection bias skews the data because the sample is not representative of the entire population. This bias can occur in various forms, such as when participants opt into a survey, choose to leave a review, or decide to join a particular study. The individuals who volunteer are often more motivated, have stronger opinions, or have more at stake compared to those who don’t participate. This can lead to an overrepresentation of certain viewpoints or characteristics and an underrepresentation of others.
To combat selection bias in your surveys, SurveySparrow’s automated sampling and multi-channel distribution ensure a diverse and representative respondent pool. This helps in collecting unbiased and accurate data for your research. Try it for FREE today.
Take action now to identify and reduce selection bias
A personalized walkthrough by our experts. No strings attached!
Example of Self-Selection Bias
Let’s consider a survey on job satisfaction. Imagine an organization sending out an email invitation to all its employees, asking them to participate in a survey about their job satisfaction. Those who feel very strongly about their jobs—whether they are extremely satisfied or extremely dissatisfied—are more likely to take the time to respond. On the other hand, employees who feel neutral or moderately satisfied might ignore the survey, either because they don’t feel compelled to share their opinions or because they don’t see the survey as important.
As a result, the survey results will predominantly reflect the views of those with strong feelings, creating a skewed representation of overall job satisfaction within the organization. The data might suggest that employees either love or hate their jobs, with few responses indicating moderate satisfaction. This self-selection bias can lead management to make decisions based on incomplete or misleading information, believing there is a significant problem or a high level of satisfaction that doesn’t actually exist.
Sample-Selection Bias
Sample-selection bias occurs when the sample selection method leads to certain members of the population being less likely to be included than others. This bias can happen intentionally or unintentionally, significantly affecting the generalizability of the study results. When a sample does not represent the entire population, the study’s findings may be skewed, leading to incorrect conclusions and potentially flawed decision-making.
Sample selection bias can arise in various ways. It might occur if the sampling process inherently favors certain groups over others. This can be due to the method of data collection, the availability of participants, or the criteria used to include individuals in the study. The bias can be subtle and often goes unnoticed, but its impact on the validity of the research can be substantial.
Example of Sample-Selection Bias
Consider a study aiming to understand the health outcomes of a population. If the study collects data solely through online surveys, it might inadvertently exclude individuals without internet access. These individuals, who are not represented in the sample, could have different health outcomes compared to those who have internet access. This exclusion leads to sample-selection bias, as the sample does not accurately represent the entire population. The study results might then overestimate or underestimate certain health outcomes, giving a distorted view of the actual health status of the population.
Exclusion Bias
Exclusion bias occurs when certain groups are systematically left out of a study, leading to skewed results that do not accurately reflect the population as a whole. This type of bias can arise from the study design or during the data collection process. When specific groups are excluded, the findings may not be generalizable, which can compromise the validity of the research.
Exclusion bias is particularly problematic because it often goes unnoticed until after the data has been collected and analyzed. By then, it can be challenging to correct the bias without redesigning the study or collecting additional data. Understanding and addressing exclusion bias is crucial for producing accurate and reliable research outcomes.
Example of Exclusion Bias
Consider a medical research study investigating the effectiveness of a new medication. If the study only includes participants who do not have any pre-existing conditions, the results may not accurately reflect how the medication affects the general population, which includes individuals with various health conditions. This exclusion of a specific group can lead to biased results, as the medication might interact differently in people with pre-existing conditions.
Survivorship Bias
Survivorship bias occurs when a sample only includes subjects that have “survived” a particular process, ignoring those that did not. This bias can lead to overly optimistic conclusions because it overlooks the failures that are no longer visible. By focusing only on the successes, the results can be misleading, suggesting that certain strategies or practices are more effective than they actually are.
Survivorship bias is a common issue in many fields, including business, finance, and research. It can distort our understanding of what factors contribute to success, leading us to draw incorrect conclusions and potentially make poor decisions based on incomplete data.
Example of Survivorship Bias
Consider a study on business success that only analyzes companies currently in operation. This study would ignore businesses that have failed, leading to survivorship bias. The results might falsely suggest that certain strategies are highly effective without considering the failures of those same strategies.
Nonresponse Bias
Nonresponse bias occurs when a significant portion of the selected sample does not respond to a survey or study. If the non-responders differ in meaningful ways from those who do respond, the results can be skewed. This bias can significantly affect the accuracy and reliability of the study’s findings, leading to conclusions that do not accurately reflect the views or characteristics of the entire population.
Nonresponse bias is a common challenge in research, particularly in surveys where participation is voluntary. It is crucial to identify and address this bias to ensure that the data collected is as representative as possible.
Example of Nonresponse Bias
In a survey about political opinions, if individuals who are disinterested in politics are less likely to respond, the findings will be biased towards those who are more politically active. This nonresponse bias can lead to inaccurate conclusions about the general population’s political views.
Selection Bias vs. Sampling Bias
Criteria | Selection Bias | Sampling Bias |
Definition | Bias that occurs when the selection of participants leads to a non-representative sample | Bias that occurs when the sample itself is not representative of the population |
Cause | Arises from the way participants are chosen | Arises from the method used to select the sample |
Example | Including only volunteers for a survey, excluding others | Using a phone survey that only includes landline users |
Impact | Results cannot be generalized to the broader population | Results are skewed and do not accurately reflect the population |
Detection | Can be identified by examining the selection process | Can be identified by evaluating the sampling method |
Prevention | Ensure random and unbiased participant selection | Use random sampling techniques to ensure representativeness |
It is important to understand the distinction between selection bias vs. sampling bias. While they are related, they are not the same.
Selection bias refers to the process by which the sample is chosen in a way that certain members of the population are more or less likely to be included.
Sampling bias is a broader term that includes any bias in the sampling process, including selection bias. It encompasses all aspects that cause the sample to be unrepresentative of the population.
How Selection Bias Occurs
Selection bias occurs when the selection of participants or data points for a study does not properly represent the intended population. This can happen due to several factors, including:
- Non-random sampling: When participants are not randomly selected, certain groups may be overrepresented or underrepresented.
- Self-selection: When individuals volunteer to participate, those who choose to participate may differ significantly from those who do not.
- Exclusion of certain groups: Systematic exclusion of certain groups during the sampling process can lead to biased results.
SurveySparrow’s advanced analytics tools are designed to identify and adjust for biases in your survey data. By using SurveySparrow, you can enhance the reliability of your survey results, ensuring that your insights are both precise and actionable. Try our tool today.
Take action now to identify and reduce selection bias
A personalized walkthrough by our experts. No strings attached!
Examples of Selection Bias in Research
Understanding what is selection bias in research is crucial for designing robust studies. Here are some examples of selection bias:
Example 1: Health Studies
A health study aiming to understand the effects of a new diet might recruit participants through a fitness website. This method likely attracts health-conscious individuals who are already engaged in healthy behaviors, leading to selection bias. The study’s findings may not apply to the general population, which includes people with varying levels of health awareness and behaviors.
Example 2: Political Polls
Political polls often face selection bias if they rely solely on landline phone calls. Younger individuals and those from lower socioeconomic backgrounds are less likely to have landlines, leading to their underrepresentation in the poll results. This bias can skew the poll outcomes and fail to accurately predict election results.
Example 3: Workplace Studies
A study examining workplace satisfaction might send surveys to employees via email. If the survey has a low response rate, and only those with strong opinions (positive or negative) respond, the results will be biased. This nonresponse bias can lead to inaccurate conclusions about overall employee satisfaction.
How to Avoid Selection Bias in Research
Mitigating selection bias is essential for ensuring the accuracy and reliability of research findings. Here are some strategies to avoid selection bias:
Random Sampling
One of the most effective ways to avoid selection bias is through random sampling. By randomly selecting participants from the population, each member has an equal chance of being included, reducing the risk of bias.
Stratified Sampling
Stratified sampling involves dividing the population into subgroups (strata) based on specific characteristics and then randomly sampling from each stratum. This ensures that all relevant subgroups are represented in the sample, reducing the risk of selection bias.
Ensuring Inclusivity
To avoid exclusion bias, researchers should design studies that include a diverse range of participants. This might involve using multiple recruitment methods to reach different segments of the population and ensuring that eligibility criteria are broad enough to include various groups.
Related: The Best Diversity And Inclusion Survey Questions To Ask
Increasing Response Rates
To minimize nonresponse bias, researchers can take steps to increase response rates. This might include sending reminders, offering incentives for participation, and making the survey or study as accessible and convenient as possible.
Transparency in Methodology
Researchers should clearly document and report their sampling methods, including any potential sources of bias. Transparency in methodology allows for better scrutiny and helps other researchers replicate the study or account for the biases in their analyses.
For a detailed reading on survey bias with examples, this blog post will come in handy.
Conclusion
Understanding what is selection bias and its impact on research is crucial for conducting accurate and reliable studies. By recognizing the different types of selection bias and implementing strategies to mitigate them, researchers can enhance the validity of their findings. Whether it’s self-selection bias, sample-selection bias, or other forms of bias, addressing these issues is essential for producing meaningful and generalizable results. Regularly reviewing and updating research methodologies to account for potential biases ensures the integrity and credibility of research outcomes.
By focusing on reducing selection bias, researchers can improve the accuracy of their studies, leading to better-informed decisions and advancements in their respective fields.
Frequently Asked Questions (FAQs)
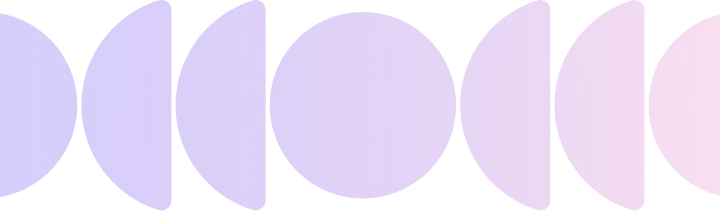