What is Data Analysis in Research?
A comprehensive guide to data analysis in research, covering its importance, steps, and tools.
Unlock game-changing insights before your competitors do. Start your SurveySparrow trial now!
14 days Free Trial
No Credit Card Required
Use SurveySparrow for FREE—Forever
What will happen if you don’t understand your customers? You will get fewer and fewer customers by the day, which will ultimately lead to the downfall of your business. In this day and age, you must understand customer behavior to provide a user-friendly experience. And for that, your decisions should be data-backed.
With rapid growth in various fields of business, the need for data analysis has increased. Developing a method of analysis has become an essential factor, and you can get this done by using various software and other tools.
What is Data Analysis?
Data analysis refers to collecting, cleaning, analyzing, and interpreting data to derive observations to support decision-making in a business. It involves breaking down large databases to observe influence and shifts in trends.
Data analysis is an important factor in research as well as business. Data analysis helps you understand the market trend research, which allows your business to apply the analysis to maximize its output/revenue. There are various methods of data analysis you can use; one such is predictive analysis, where historical and statistical techniques are used to forecast future outputs.
As an analyst, your role is to collect data from the market, separate the data from any errors, and understand what the data tells you. It’s like solving a puzzle box. Solving the puzzle gets you a beautiful picture. In the same way, after interpreting the data, decision-making becomes easier, which in turn helps the growth of the business.
Why is Data Analysis Important?
Data analysis helps you to make better decisions in your business, improve your operations, and stay ahead of the market trends. Data analysis reveals data that is sometimes lost in a larger database. Here’s how data analysis becomes an important factor in your business:
Smart Decision-making
With data analysis, your decisions are made on facts rather than on guesses. Whether choosing the right strategy or deciding which product to launch next, the decisions are more likely to lead to success. You can assess potential risks and rewards with the analysis and make a confident choice.
Better Understanding
Analyzing data helps you understand your customers more. You can get more connected to the changes in trends and understand how well your business is working. When you know these things, you can make choices that align with what you want to achieve.
Reducing Risks
Looking at past data helps you spot patterns that might point to possible dangers. This lets you take steps to prevent them. In fields like finance, examining data can help you find fraud by spotting odd transactions. This forward-thinking approach helps cut money losses, shield customers, and protect your company’s goodwill.
Stay Ahead of Competitors
Looking at data helps you see new chances and risks in the market. This lets you change your plans before other companies do. When you know about trends, how people buy things, and how well other businesses are doing, you can change direction fast and come up with new ideas. Being able to act based on what the data shows gives you a big advantage in a market that changes.
Efficient Use of Resources
Data analysis assists you in using the resources (money, people, material, etc.) efficiently. Hospitals, for example, can employ data to allocate staff according to the number of patients. This ensures you spend resources where they matter most by making improvements and giving customers or clients a better return on the funds.
Continual Improvement
Using data analysis, progress can be monitored, and you can use performance metrics to identify areas for improvement. Take the time to analyze, change, and re-analyse what is there to improve your products or services, implementing changes for a better product or service, leading to better overall performance.
RELATED: Ways to Improve Customer Experience: 10 Proven Techniques
Steps Included in Data Analysis
There are mainly six steps included in data analysis, and most of the time these steps are followed in the given order. The six processes through which data analysis is performed are:
Step 1: Understanding the problem
An issue is assigned to the data analyst in the first step of the procedure. The analyst must define the problem and understand what is causing the issue.
For example, if you notice a drop in sales, your goal is to identify what made the sales downward.
In this initial step of problem-solving, it’s crucial to collect relevant data to fully understand the issue. Tools like SurveySparrow can be valuable here, enabling you to gather actionable feedback from customers or teams through engaging surveys, helping identify key factors behind the problem and driving informed solutions.
Don’t be left behind.
Get exclusive access to SurveySparrow's advanced analysis tools. Limited spots available!"
14-Day-Free Trial • Cancel Anytime • No Credit Card Required • Need a Demo?
Step 2: Collecting the data
The second step is to collect the data. The analyst has to collect the data based on the problem that has arisen. The data has to be collected from internal or external sources.
The data that is collected by an individual themselves is called first-party data.
The data that is collected and marketed is called second-party data.
Data that is collected from external sources is called third-party data.
The common sources from which the data is collected are interviews, surveys, and questionnaires. The data collected can be stored in MS Excel, Google Sheets, or a SQL database.
Step 3: Data cleaning
The third step is to clean the data. Data cleaning means removing any misspellings, repetitions, and irrelevance from the data. Clean data largely depends on data integrity. The extra data is cleared and cleaned because it may be duplicated or not in the proper format.
Both SQL and Excel offer several functions for cleaning data.
One of the most crucial phases in data analysis is this one since well-formatted, clean data makes it easier to identify patterns and locate answers. Biassing should be avoided as it could impact the data analysis.
Step 4: Analyzing the data
Once the data is cleaned, it’s time for the analysis. The analysis of the data is the fourth stage. This involves applying various techniques to the data to discover patterns, relationships, or trends, depending on your goal.
All types of data fit into these four categories:
Descriptive Analysis
Descriptive analysis focuses on what occurred. It is the starting point for any research before deeper explorations. As the first step, breaking down the data and summarizing its key characteristics is involved.
Diagnostic Analysis
This analysis focuses on the question of why it’s happening. Just as a mechanic inspects the car to uncover the issue, you can use diagnostic analysis to reveal the underlying cause of the problem.
Predictive Analysis
This type of analysis allows you to forecast future trends based on historical data. It generally uses results from the above analysis, machine learning (ML), and artificial intelligence (AI) to identify future growth.
Prescriptive Analysis
Now that you know what to do, you must also understand how you’ll do it. The prescriptive analysis aims to determine your research’s best course of action.
Step 5: Data interpretation
The fifth step is data interpretation where the modified data has to be made into easily understandable material. This process involves the creation of visual representations, such as charts, graphs, and tables, to effectively communicate insights gained from the data analysis.
This helps in interpreting complex information more easily. A presentation is made based on the results of data findings. It helps in making more informed decisions and it leads to better outcomes.
Tools Used in Data Analysis
For a good analysis, you need to use various tools and techniques to gather and analyze data. Here are some of the renowned as well as effective ones:
- Excel: Widely used for visualization and basic data analysis. It is ideal for anyone performing data analysis.
- R: A programming language and environment for graphics and statistical computing. Has a simple programming language and many operators for calculations.
- Python: A versatile programming language with libraries like Pandas, Scrapy, and Seaborn for data analysis.
- SPSS: A user-friendly software package used for interactive or grouped statistical analysis.
- SAS: A software suite used for advanced analytics, information retrieval, and data management.
- Google Sheets: A remarkable and cost-effective tool for fundamental data analysis. It is known for its seamless collaboration capabilities.
- Google Analytics: This tool provides insights into website traffic, user behaviour, and performance to make data-driven decisions.
- RapidMiner: Ideal for data mining and model development. This platform offers remarkable predictive analytics capabilities.
- Tableau: One of the best commercial data analysis tools, best for its interactive dashboards and data exploration capabilities.
- Power BI: It has incredible data integration features and interactive reporting, which makes it ideal for enterprises.
- KNIME: Short for Konstanz Information Miner, is an outstanding tool for data mining. It has a user-friendly graphical interface and is a cost-effective choice.
Data Analysis in Research: How SurveySparrow fits into the Picture
While we’ve covered a range of powerful tools for data analysis, it’s worth highlighting how SurveySparrow can be a game-changer in your data collection and analysis process.
SurveySparrow shines when it comes to gathering fresh, relevant data directly from your target audience. Its user-friendly interface makes creating surveys a breeze, whether you’re looking to understand customer preferences, employee satisfaction, or market trends.
But SurveySparrow isn’t just about collecting data – it’s about making that data work for you. The platform offers built-in analytics tools that help you spot patterns and trends quickly. You can visualize your survey results with eye-catching charts and graphs, making it easier to present your findings to stakeholders or team members.
What sets SurveySparrow apart is how it bridges the gap between data collection and analysis. You’re not just getting numbers – you’re getting insights. The platform’s features allow you to segment your responses, compare different data sets, and even track changes over time. This means you can start drawing meaningful conclusions from your data right away, without having to export it to another tool first.
It’s a valuable addition to any data analyst’s toolkit, especially for those who want to keep their finger on the pulse of their audience or organization.
Don’t be left behind.
Get exclusive access to SurveySparrow's advanced analysis tools. Limited spots available!"
14-Day-Free Trial • Cancel Anytime • No Credit Card Required • Need a Demo?
Frequently Asked Questions (FAQs)
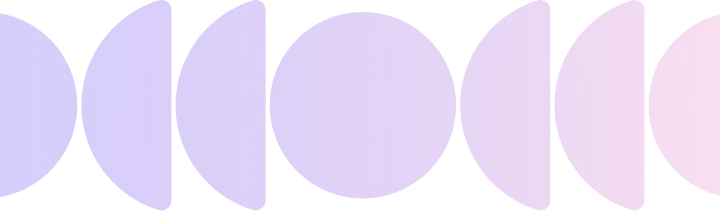