Customer Experience
Simple Random Sampling: A Complete Guide With Examples, Steps & Applications
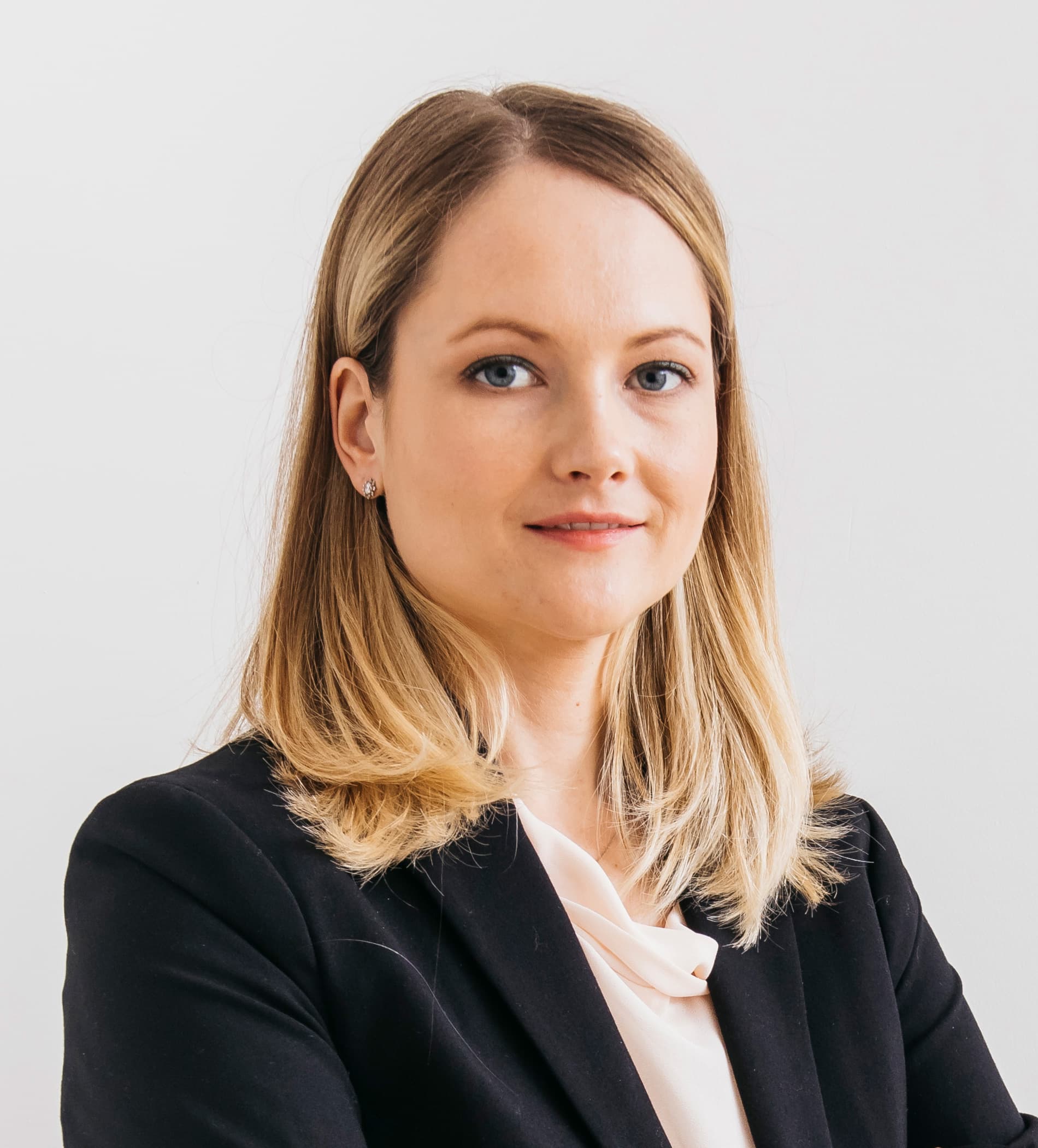
Article written by Kate Williams
Content Marketer at SurveySparrow
11 min read
22 April 2025
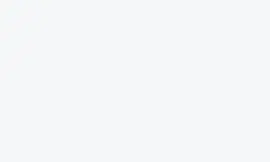
60-Second Summary:
Is Your Data Truly Random?
Want to be absolutely sure your survey data is unbiased? This guide to Simple Random Sampling (SRS) is your answer!
Know more about:
- The Core Concept: Understand exactly what SRS is and why it's the gold standard for unbiased data.
- When to Use Simple Random Sampling: Find the ideal situations to apply SRS for maximum accuracy.
- Step-by-Step Process: Get a clear walkthrough, from defining your population to selecting your sample, for seamless implementation.
- Pros & Cons: Weigh the advantages and disadvantages to make smart decisions about your survey methodology.
- How to Avoid Bias: Learn how to use SRS to eliminate bias and get data that truly represents your target audience.
- Beyond the Basics: Understand where SRS fits among other sampling methods for optimal results.
If you want to ensure the integrity of your survey data and make confident decisions, knowing Simple Random Sampling is a MUST. Read on!
What is Simple Random Sampling?
Definition: A probability sampling method where each member of the population has an equal and independent chance of being selected, with selections made randomly without any systematic pattern.
Simple random sampling stands as the foundation of probability sampling methods, where each member of a population has an equal chance of being selected. The numbers are interesting – properly executed random sampling can achieve 95% confidence levels with margins of error as low as ±3%.
This method is often used in research studies to ensure that the data collected is representative of the entire population. It is considered one of the most basic forms of sampling and the most efficient way to select a sample from a population.
For example, imagine a company with 500 employees wanting to survey 50 people about workplace satisfaction. With simple random sampling, each employee has exactly a 10% chance of being selected, regardless of their department, seniority, or any other factor.
Simple Random Sampling in the Real World
Understanding simple random sampling in theory is one thing, but actually applying in real-world contexts is what gives you the clarity to its value and implementation.Â
Let’s look at three practical applications that demonstrate this technique in action:
Market Research Case Study
A telecommunications company needed to assess customer satisfaction across their national customer base of 2.3 million subscribers. They use simple random sampling, they select 1,500 customers through computerized random number generation.
The result? Their survey revealed satisfaction patterns that held true when later verified against larger data sets, with a margin of error of just ±2.5%. This precision allowed them to confidently implement targeted service improvements that increased overall customer retention by 7%.
Political Polling Example
During election cycles, polling organizations regularly employ simple random sampling to predict outcomes. One notable example is the Iowa Presidential Caucus poll, where researchers randomly selected 1,247 likely voters from voter registration databases.
The random selection process ensured representation across demographic variables including age, income levels, and geographic location without explicitly stratifying for these factors. The poll predicted the winner within 2.1 percentage points of the actual result.
Academic Research Application
Researchers studying the effectiveness of a new teaching methodology randomly selected 12 schools from a district containing 78 schools. This approach ensured that schools of varying sizes, socioeconomic profiles, and previous performance levels all had equal probability of inclusion.
The findings from this randomly selected sample provided sufficient statistical power to implement the new methodology district-wide, ultimately improving standardized test scores by an average of 14%
4 Different Simple Random Sampling Techniques
There are several methods to implement simple random sampling effectively. Here are the main techniques.
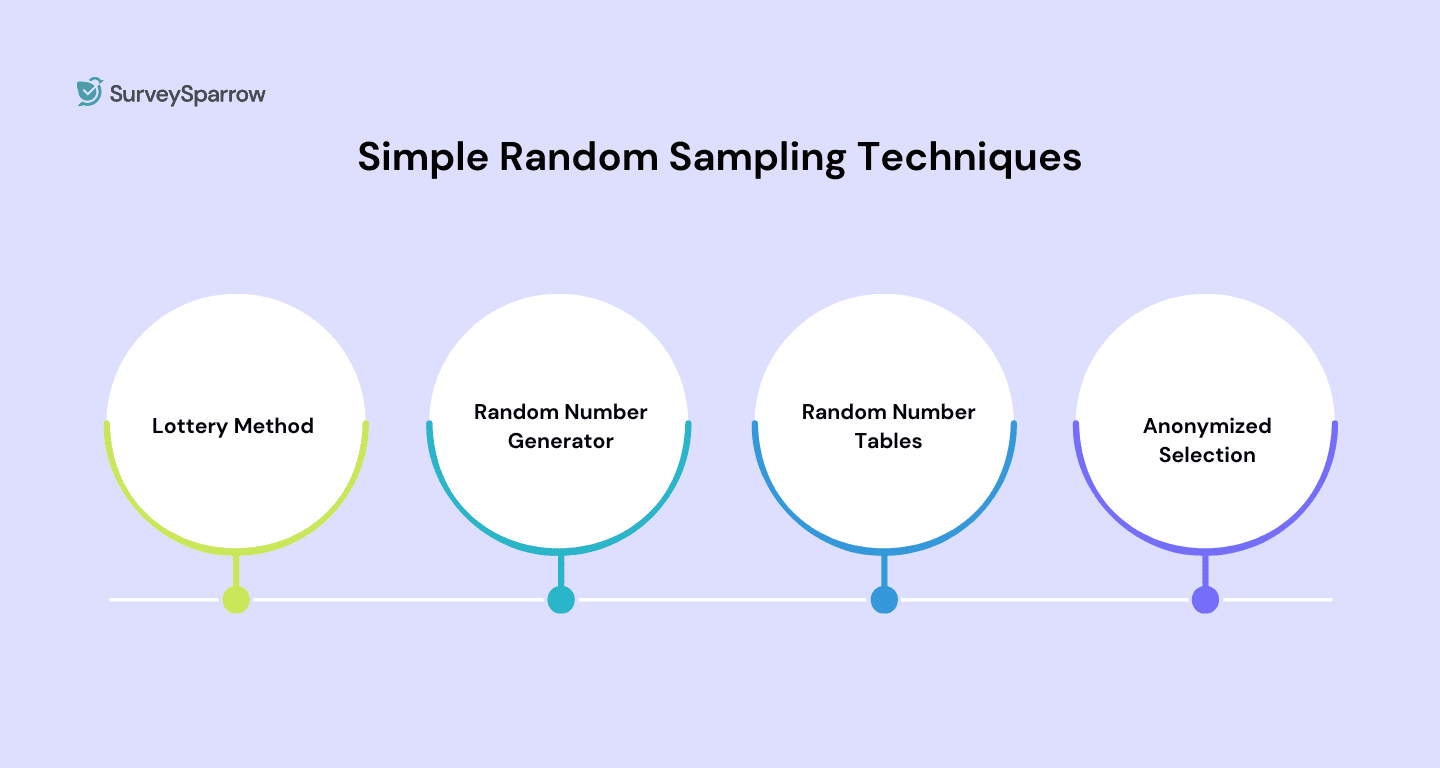
1. Lottery Method
This is a traditional sampling method. In this, each member of the population is assigned a unique number. All numbers are placed in a container (like a box or hat), and numbers are drawn randomly without looking.
For example, suppose you have 100 employees and want to select 10. Then, you would write numbers 1 to 100 on slips of paper, mix them, and draw 10 slips.
2. Random Number Generator
This simple random sampling technique uses software to generate numbers. These are the numbers that's assigned to the members of your population.
For example, suppose you want to select 50 students from a total of 500. The software will generate 50 unique numbers between 1 and 500. The students corresponding to those numbers would be your sample.
3. Random Number Tables
In this method, researchers can use pre-printed tables that contain random numbers. Each number corresponds to a member of the population.
For instance, if you have a list of participants numbered from 1 to N, you can look up random numbers in the table and select participants based on those numbers.
4. Anonymized Selection
This is similar to the lottery method. Same, Same, but different - if you know what we mean. In this technique, instead of using numbers, names are written on slips of paper and drawn randomly.
Consider a classroom setting, for example. You might write each student's name on a slip of paper and draw names to form a sample group.
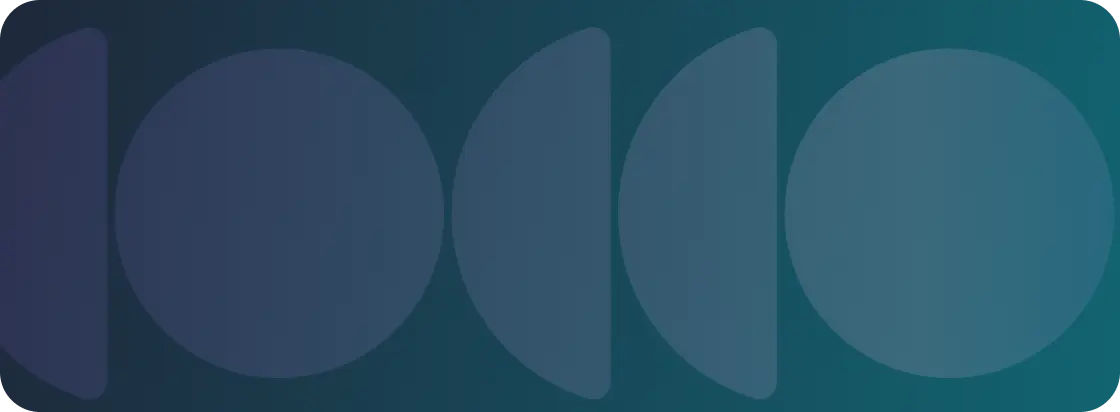
Simplify Your Sampling Efforts with an Intuitive AI Survey Tool!
A personalized walkthrough by our experts. No strings attached!
How to Do Simple Random Sampling | 8 Simple Steps
Here is a step-by-step guide on how you can conduct simple random sampling.Â
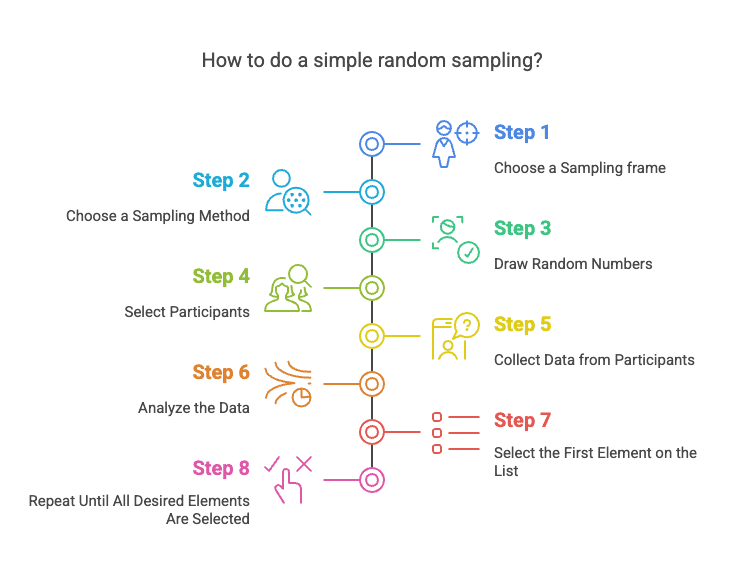
Step 1 - Choose a Sampling Frame
The sampling frame is the list of everyone in the population you want to study. You have to decide who or what you want to include in your study. For example, if you are studying students, your population might be all the students in a school.
Following up, you have to create a list of all members of this population. This could be a roster of students, a list of customers, or any other relevant group. Also, make sure the list is current and includes everyone you want to sample.
Step 2 - Choose a Sampling Method
Now, decide how you will randomly select people from your sampling frame.Â
The easiest way is to use a random number generator (RNG), which can be found online or in statistical software. For smaller groups, you can write names on slips of paper and draw them from a hat.
Step 3 - Draw Random Numbers
This step involves generating numbers that will help you select participants. If you have a printed table, pick numbers at random from it until you have enough for your sample size. If using software, enter the range of numbers that correspond to your sampling frame and let it generate random numbers for you.
Step 4 - Select Participants
Now it's time to choose your sample based on the random numbers generated. Look at your random numbers and find the corresponding names or items in your list.
Always make sure that each selected number corresponds to a different participant so that everyone has an equal chance of being chosen.
Step 5 - Collect Data from Participants
After selecting your participants, gather the information you need. There are many ways to collect data, but the fastest, easiest, and cheapest one is through surveys. While creating the surveys, make sure to curate the questions. Keep it short, to the point, and formal.
Nowadays, everything is AI, and so are surveys. SurveySparrow offers an AI-powered survey builder. Just add in your prompt and the tool will do the rest. Here's a sneak peek into how the feature will work.
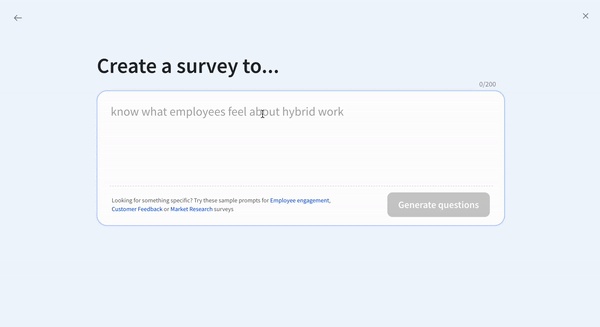
The feature is available in the free version of the tool. So, feel free to try it out.

Step 6 - Analyze the Data
Once you have collected all your data, it's time to look at what it tells you.
Depending on what you are studying, use basic statistics (like averages) or more complex methods if needed. Also, make sure your sample reflects the larger population by comparing key characteristics (like age, gender, etc.).
Step 7 - Select the First Element on the List
Identify which participant will be sampled first based on your random selection process. Go through your list and find out which participant corresponds to the first random number you generated.
Step 8 - Repeat Until All Desired Elements Are Selected
Continue selecting participants until you reach the number you need for your study.
Use your RNG or number table again for each selection to maintain randomness. And don't forget to document! Write down how you selected each participant for transparency and future reference.
Pros and Cons of Simple Random Sampling
The following are some major pros and cons of simple random sampling.
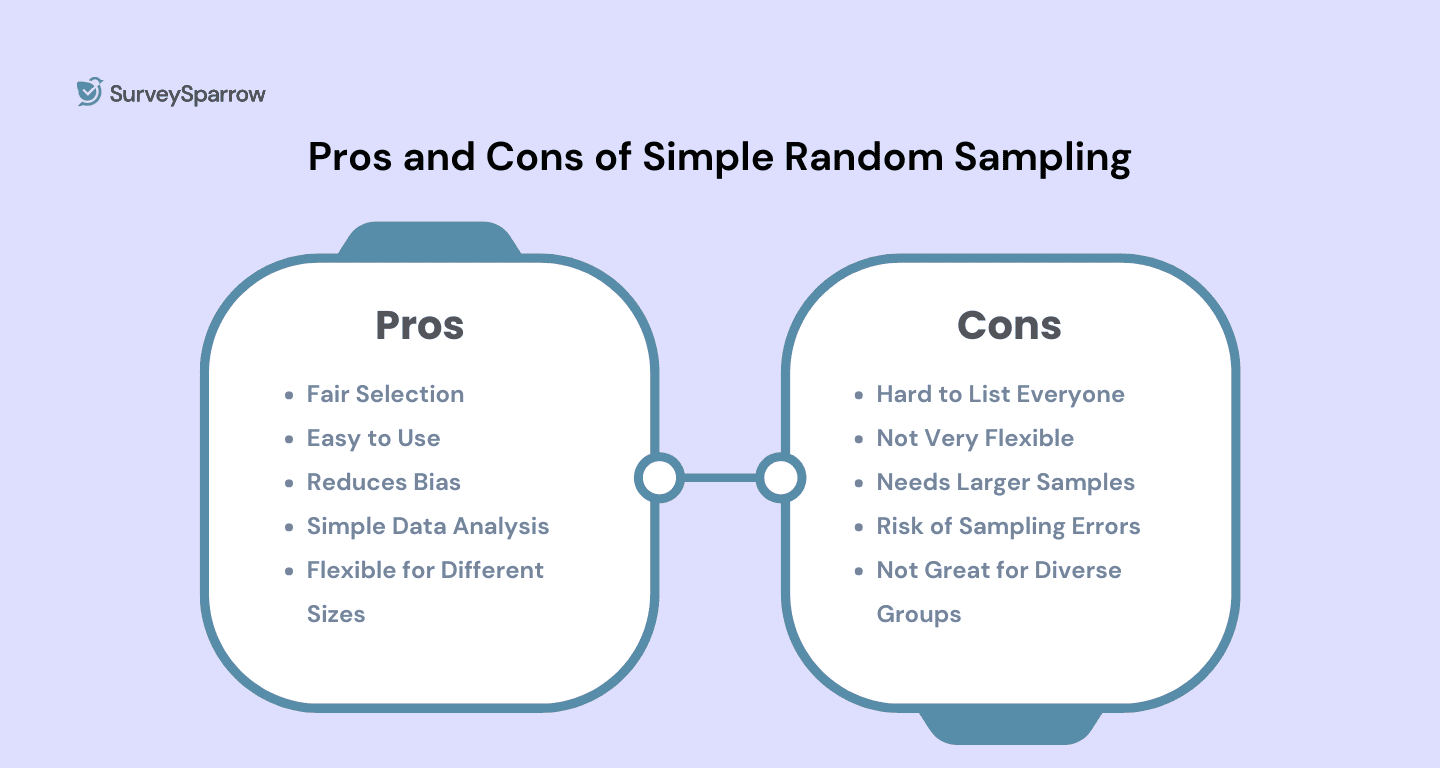
Pros of Simple Random Sampling
1. Fair Selection
Everyone in the population has an equal chance to be chosen. This helps to avoid bias and ensures that the sample represents the whole group.
2. Easy to Use
The sampling method is simple and straightforward. Anyone can understand and apply it without needing special skills or knowledge.
3. Reduces Bias
Because the selection is random, there’s less chance for sampling bias. Researchers can’t influence who gets picked, which leads to more trustworthy results.
4. Simple Data Analysis
Analyzing data from simple random samples is easier because the statistical methods used are not complicated.
5. Flexible for Different Sizes
This method works well for both small and large groups, making it a good choice for various types of research.
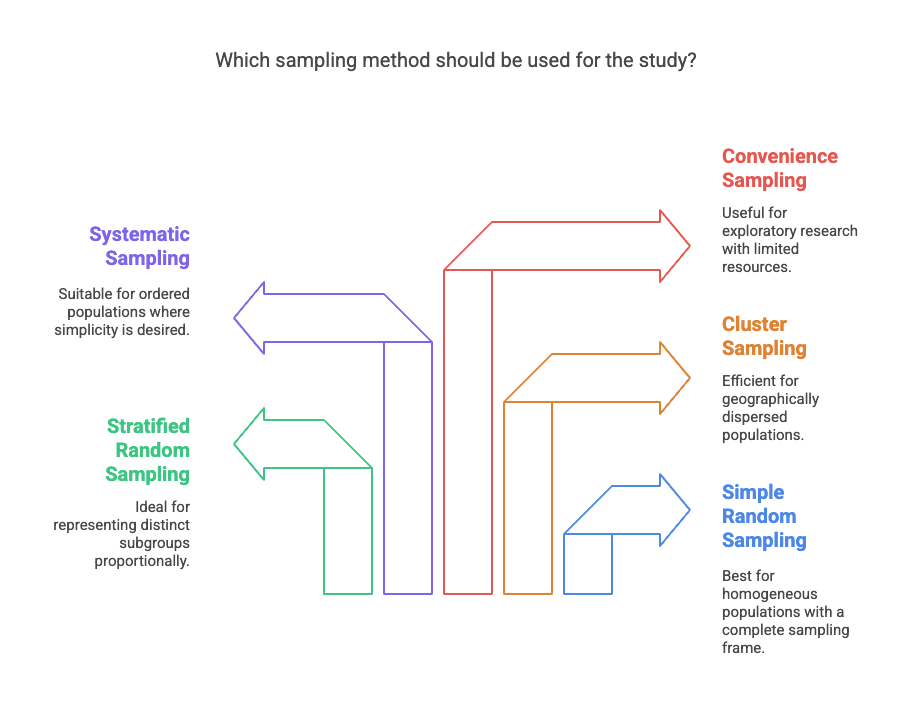
Cons of Simple Random Sampling
1. Hard to List Everyone
It can be tough to get a complete list of everyone in the population, especially if it’s large or spread out. Missing people can lead to an unbalanced sample.
2. Not Very Flexible
Once you start selecting your sample, it’s hard to change things if new information comes up about the population.
3. Needs Larger Samples
To get reliable results, you often need a larger sample size, which can take more time and money to manage.
4. Risk of Sampling Errors
Sometimes, by chance, the selected sample might not accurately represent the population. For example, you might accidentally pick mostly one type of person.
5. Not Great for Diverse Groups
If the population is very different (like having many different age groups or backgrounds), simple random sampling might miss important subgroups. In such cases, other methods like stratified sampling might work better.
Related Read: Types of sampling methods you can use.
When to Use Simple Random Sampling?
Simple random sampling is particularly valuable in specific research contexts. Consider this approach when:
Homogeneous Populations
When your population is relatively uniform regarding the variables you’re studying, simple random sampling excels. With lower variance across the population, even smaller random samples can accurately represent the whole.
Example: A company with employees predominantly in similar roles and demographics might effectively use simple random sampling for an employee satisfaction survey.
Need for Statistical Inference
Research requiring rigorous statistical analysis and inference benefits from the mathematical properties of simple random sampling.
Example: Pharmaceutical research often employs simple random sampling in clinical trials to ensure statistical validity when drawing conclusions about drug efficacy.
Preliminary Research Phases
When conducting exploratory research where population characteristics are still unclear, simple random sampling provides an unbiased first look.
Example: Market researchers exploring consumer interest in a novel product category might start with simple random sampling before implementing more targeted approaches.
Need for Procedural Simplicity
Projects with limited statistical expertise or resources may benefit from the straightforward implementation of simple random sampling.
Example: Small businesses conducting customer feedback surveys often use simple random sampling due to its operational simplicity and clear methodology.
External Credibility Requirements
When research findings will face scrutiny from external stakeholders, the objectivity of simple random sampling enhances credibility.
Example: Government agencies often employ simple random sampling for public opinion surveys to demonstrate impartiality in data collection.
Wrapping Up
Simple random sampling is best used when you want every individual in your population to have an equal chance of being selected. Be it for market research or any research, when executed properly, it offers unparalleled objectivity and forms the basis for legitimate statistical inference.
However, successful implementation requires careful consideration of population characteristics, sampling frame completeness, and resource constraints. You’ll need to understand both the strengths and limitations of this approach to make informed decisions on when to use simple random sampling and when alternative methods might better be a fit.
For organizations seeking to implement effective sampling strategies, SurveySparrow offers tools that simplify the process from sample selection through data collection and analysis.
Ready to conduct your own research using proper sampling techniques?
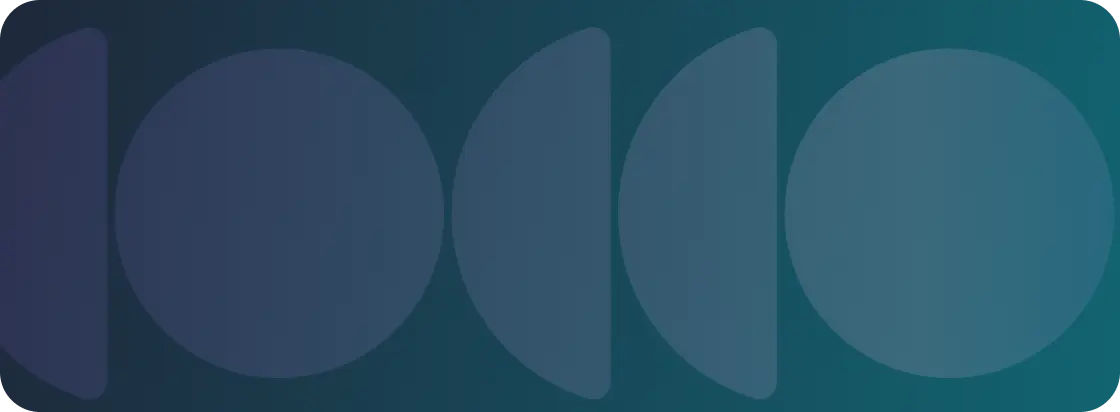
Simplify Your Sampling Efforts with an Intuitive AI Survey Tool!
A personalized walkthrough by our experts. No strings attached!
Start 14 Days free trial
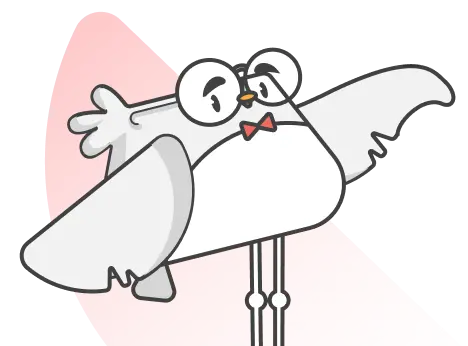
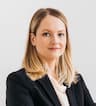
Kate Williams
Frequently Asked Questions (FAQs)
The appropriate sample size depends on three key factors: population size, desired confidence level, and acceptable margin of error. For most business and social research, a 95% confidence level with a ±5% margin of error is standard. For a population of 10,000, this typically requires a sample of approximately 370 participants. Smaller margins of error or higher confidence levels require larger samples. Online sample size calculators can provide specific numbers based on your parameters.
While both are probability sampling methods, simple random sampling selects participants completely at random, giving each population member an equal chance of selection at each draw. Systematic sampling, in contrast, selects every nth member after a random starting point. The key difference is that systematic sampling creates a pattern after the initial randomization, potentially introducing bias if the population has cyclical patterns that align with the sampling interval.
When the complete population is inaccessible, researchers must either:
- Use the best available sampling frame while documenting its limitations
- Consider alternative sampling methods like cluster sampling
- Implement a two-stage sampling approach, first sampling accessible subgroups, then sampling within those
- Clearly acknowledge the limitations in generalizability due to the incomplete sampling frame
The most frequent implementation errors include:
- Incomplete or outdated sampling frames that miss segments of the population
- Improper randomization procedures that introduce systematic patterns
- High non-response rates that may create selection bias
- Insufficient sample sizes for the desired statistical precision
- Failure to properly document the sampling process for transparency
While simple random sampling is primarily associated with quantitative research, it can be valuable in qualitative studies when seeking to avoid selection bias. However, qualitative researchers often need participants with specific experiences or characteristics, making purposive sampling more appropriate. When using simple random sampling in qualitative contexts, researchers should be prepared for potentially larger sample sizes to ensure they capture the diversity of experiences relevant to their research questions.
Related Articles
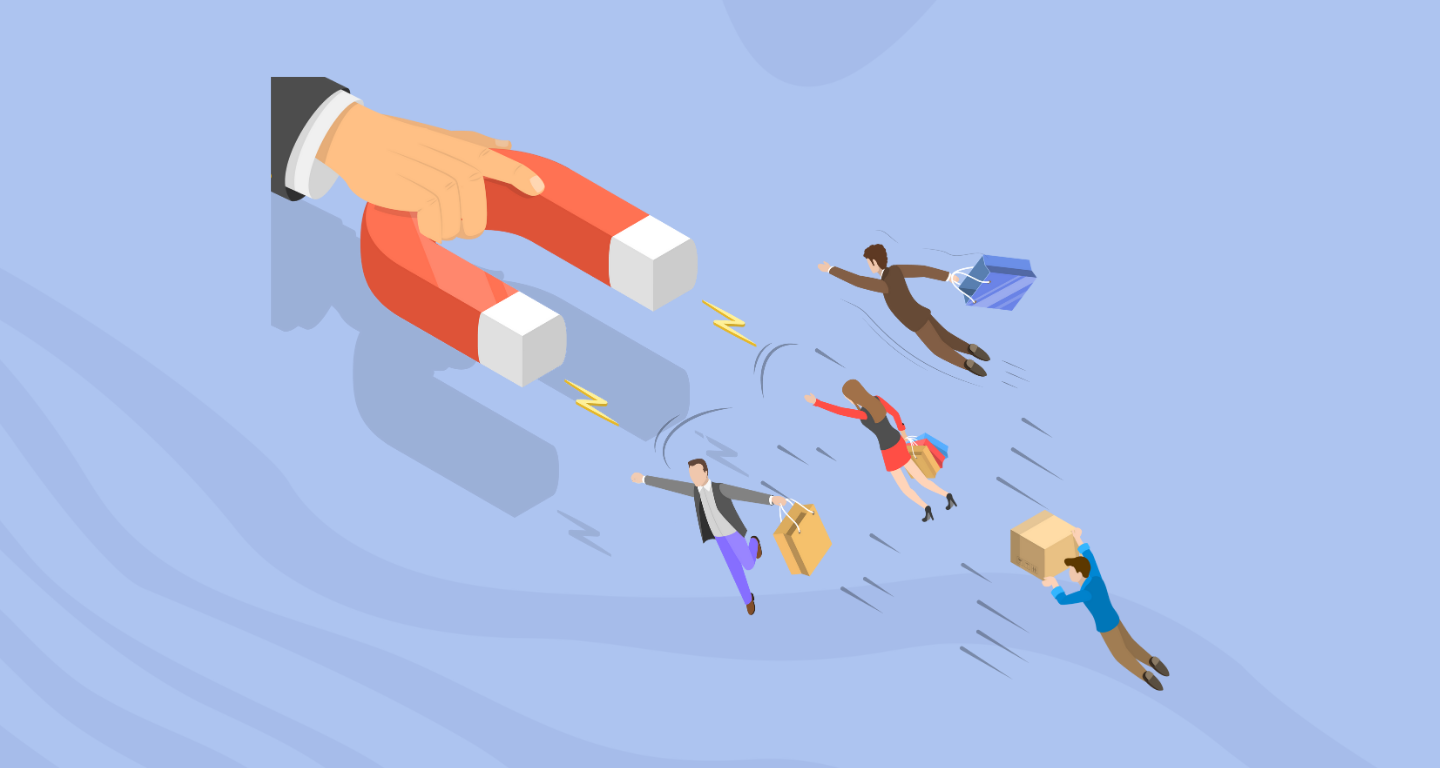
Customer Experience
Customer Retention Metrics: 5 Metrics, KPIs & How to Measure Them
12 MINUTES
2 July 2018
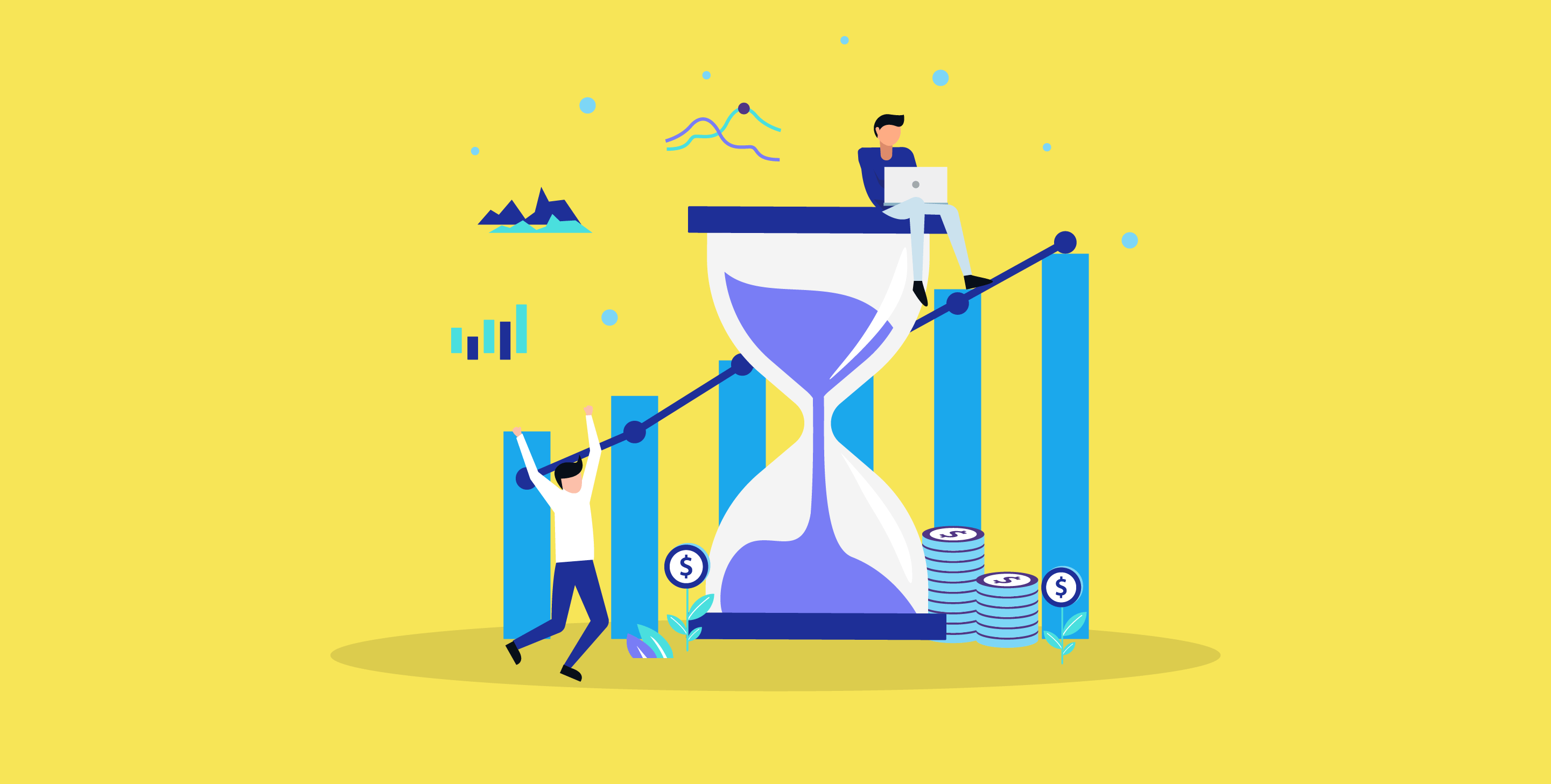
Best Of
17 Product Management KPIs and Metrics You Need to Know
22 MINUTES
10 February 2020
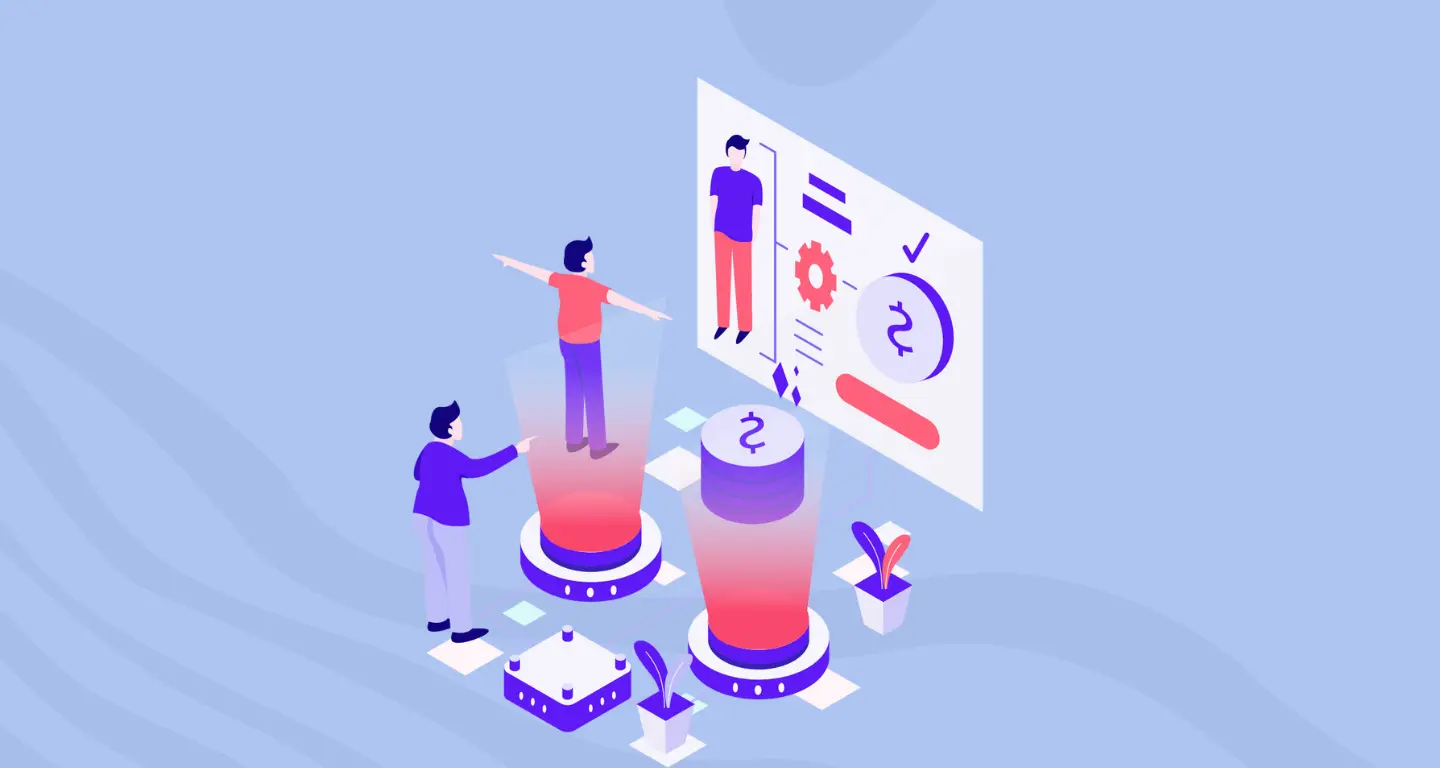
Customer Experience
Customer Experience Monitoring: The Path to Customer Delight
10 MINUTES
15 September 2023
![40 Probing Questions Examples for Customer Service: Complete Guide [2025] 40 Probing Questions Examples for Customer Service: Complete Guide [2025]](/_next/image/?url=https%3A%2F%2Fsurveysparrow.com%2Fwp-content%2Fuploads%2F2022%2F05%2Fprobing-questions-customer-service-1-2.png&w=3840&q=75)
Customer Experience
40 Probing Questions Examples for Customer Service: Complete Guide [2025]
9 MINUTES
20 May 2022