Compare
Generative AI vs Predictive AI: Exploring Key Distinctions and Applications
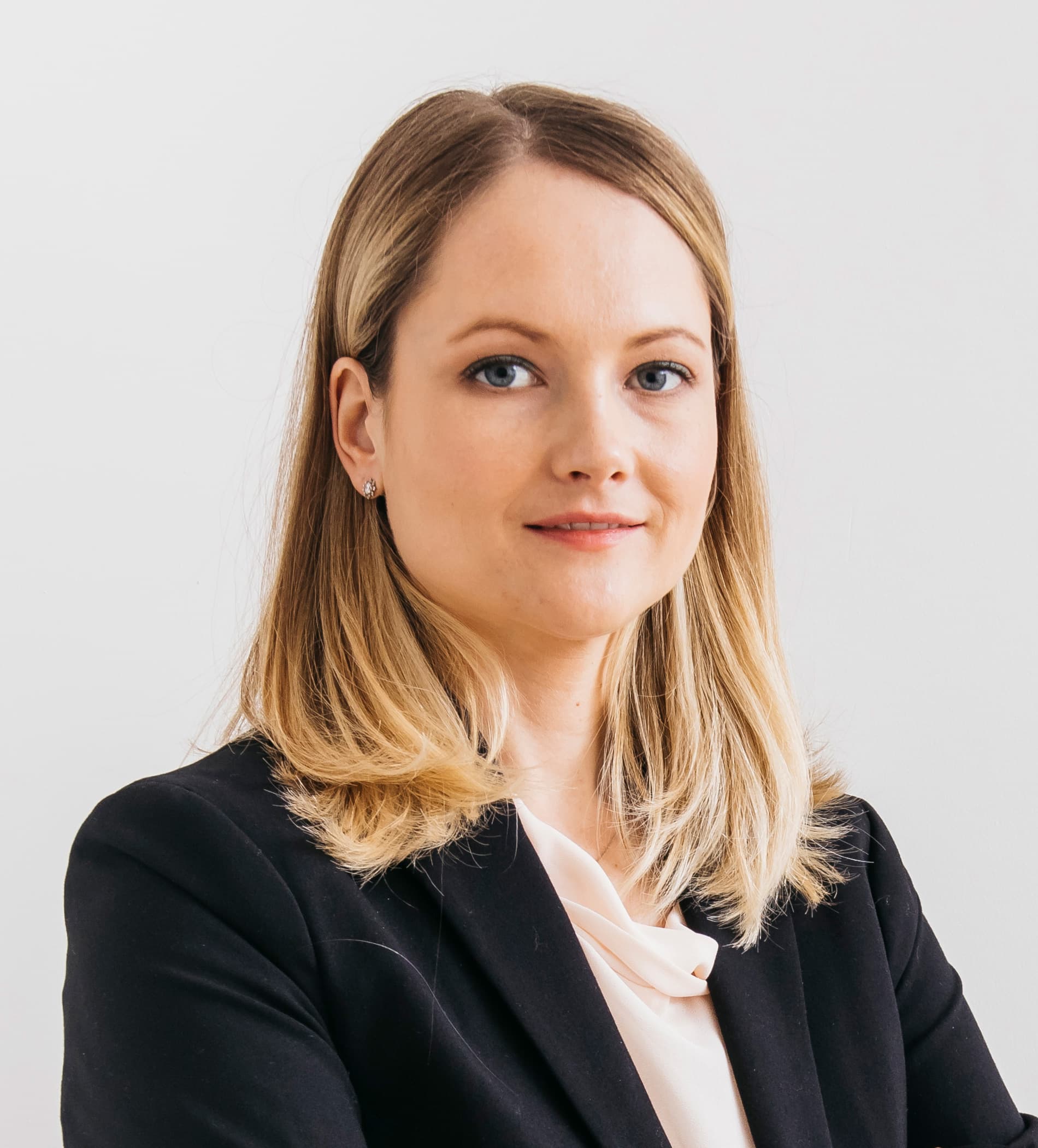
Article written by Kate William
Content Marketer at SurveySparrow
9 min read
14 May 2025
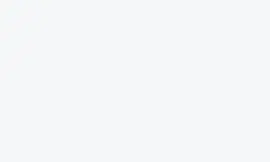
If you’re looking for information on generative AI vs predictive AI, you’ve come to the right place.
With its ever-growing leaps in technology, artificial intelligence will soon dominate the market. Generative AI and predictive AI are two of its greatest products, and both have their own individuality and approach.
These two tools have grabbed the attention of AI enthusiasts and businesses alike with their unique feature: the ability to create different content/predictions based on data. Both generative AI and predictive AI use machine learning to analyze the data provided by the user, and create the output according to their needs.
Due to their potential, they are quickly gaining popularity in the corporate world. In this blog on generative AI vs predictive AI, we will talk about what makes them special, and what sets them apart.
What is Generative AI?
The word generative itself means “able to produce or create”. So generative AI models focus on generating new content that is unique each time. Some data models are pre-loaded into the generative AI tool. This becomes the pattern, based on which the AI creates new data.
Note: Every data model has a pattern.
For example, imagine you're writing an essay on a historical personality. You will begin with their date and place of birth, and then as the essay progresses, their major achievements follow.
Similarly, generative AI learns patterns from the pre-loaded training models, and then creates its own content, which is unique. The contents are not copied from the training models. Moreover, the contents can come as diverse types, including texts, images, audio, and synthetic data.
Generative AI was introduced in the 1960s, when it was deployed in chatbots. But, with the introduction of GAN (Generative Adversarial Networks) – a complex machine learning algorithm - in 2014, generative AI could create real images, audios, and other complex content. That's when it really took off.
How Does Generative AI Work?
- The user enters a prompt. This can be anything the AI tool can process, like text, images, audios, etc.
- Next, the AI tool uses the prompt to give the user a response.
Initially, generative AI used API models to give the response, and developers used complex Python programming to get the response. Now, these tools use GANs, along with other auto-regressive algorithms and artificial neural networks (ANN), to process and generate the content. Characters, like letters and punctuation, are converted into sentences.
Moreover, any visual prompts are converted into images and are finally represented as vectors. In short, generative AI tools are now more user friendly. Users can interact with the tool to change the content style and tone based on their requirements.
What Are the Advantages of Using Generative AI?
1. Effortless content creation
Content creators can quickly create unique content by entering the right set of prompts. This is a boon for marketing teams in most industries and verticals, including engineering, marketing, and sales.
By using AI smartly, they can scale up content production across multiple areas, including blogs, emails, surveys, and more.
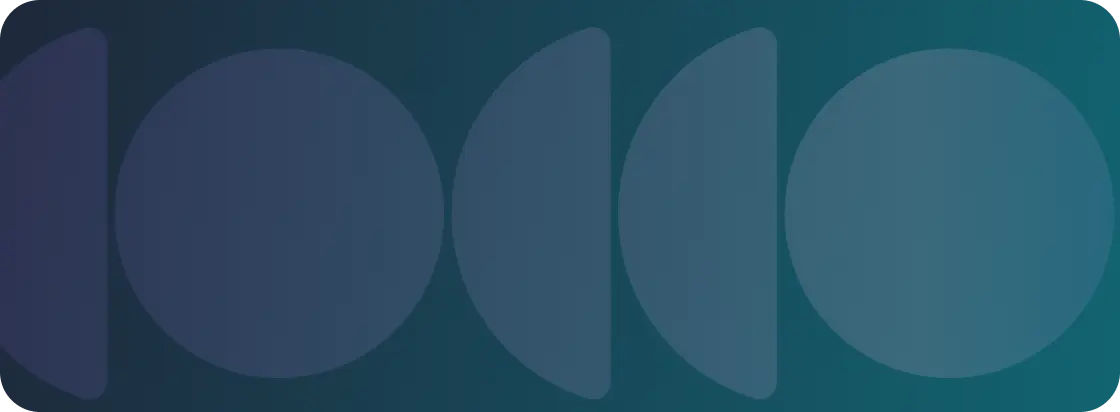
Use AI to Create Surveys
A personalized walkthrough by our experts. No strings attached!
2. Error-free communication
Not everyone can come up with good, error-free content. Either due to lack of time or writing skills, many people struggle with written communication. AI tools can help them create content that is both unique and professional.
3. Easier comprehension
Speed-reading has become second nature because one, we have too much content, and two, some content is just too hard to understand. But generative AI can help us understand the main points in a much easier way.
By prompting it to summarize a text or an article using an AI summarizer, it transforms complex content into a simple summary.
4. Error-proof email marketing
81% of companies use email as part of their core marketing strategy. If you're one of the 81%, you can use AI to craft high-quality, conversational email templates for key areas - like customer service, feedback emails, and more. Additionally, generative AI is equally helpful when it comes to personalization.
85% of marketers say AI boosts their content personalization.
What Are the Disadvantages of Generative AI?
1. Reduced critical thinking
This is one of the worst ill effects of generative AI, and it works in two ways:
One: AI is based on algorithms.
These algorithms create responses according to predefined patterns in their data model. As personal experience (and articles like these) show, this can lead to responses that are confusing or at the worst, misleading.
Two: AI tools are both quick and convenient.
This can tempt people to become more dependent on AI tools for an easy solution. As a result, they are losing creative skills like deep thinking and the ability to collaborate with others.
Solution: Use AI to handle repetitive tasks like research and code generation. Don't use it as a replacement for you. For instance, as a creator, you still want to fact-check the content, and add your own perspective.
2. Loss of job opportunities
Generative AI is becoming more capable of creating art and generating content. So less importance is given to writers and designers, and there is a risk they may be replaced. This is especially high for roles that are purely about execution. For example, designing blog banners or presentations.
Solution:
This is where retraining steps in. By learning how to work with AI, humans can use it in a way that enhances their own creativity.
3. AI Bias
Generative AI, at times, presents content that contains racial or other biases. This happens due to human biases that can skew the original data model. Researchers have found examples of AI bias in a wide range of use cases, from academic research to healthcare.
Solution:
Always fact-check the output. Also, depending on the use case, you can set conditions for the AI's output to be considered as acceptable. For example, you're using an AI tool to generate images about work culture. So you can decide, "Okay, I want the images to represent an equal mix of men and women, from diverse racial backgrounds."
Example of Generative AI: Chat GPT 4.0
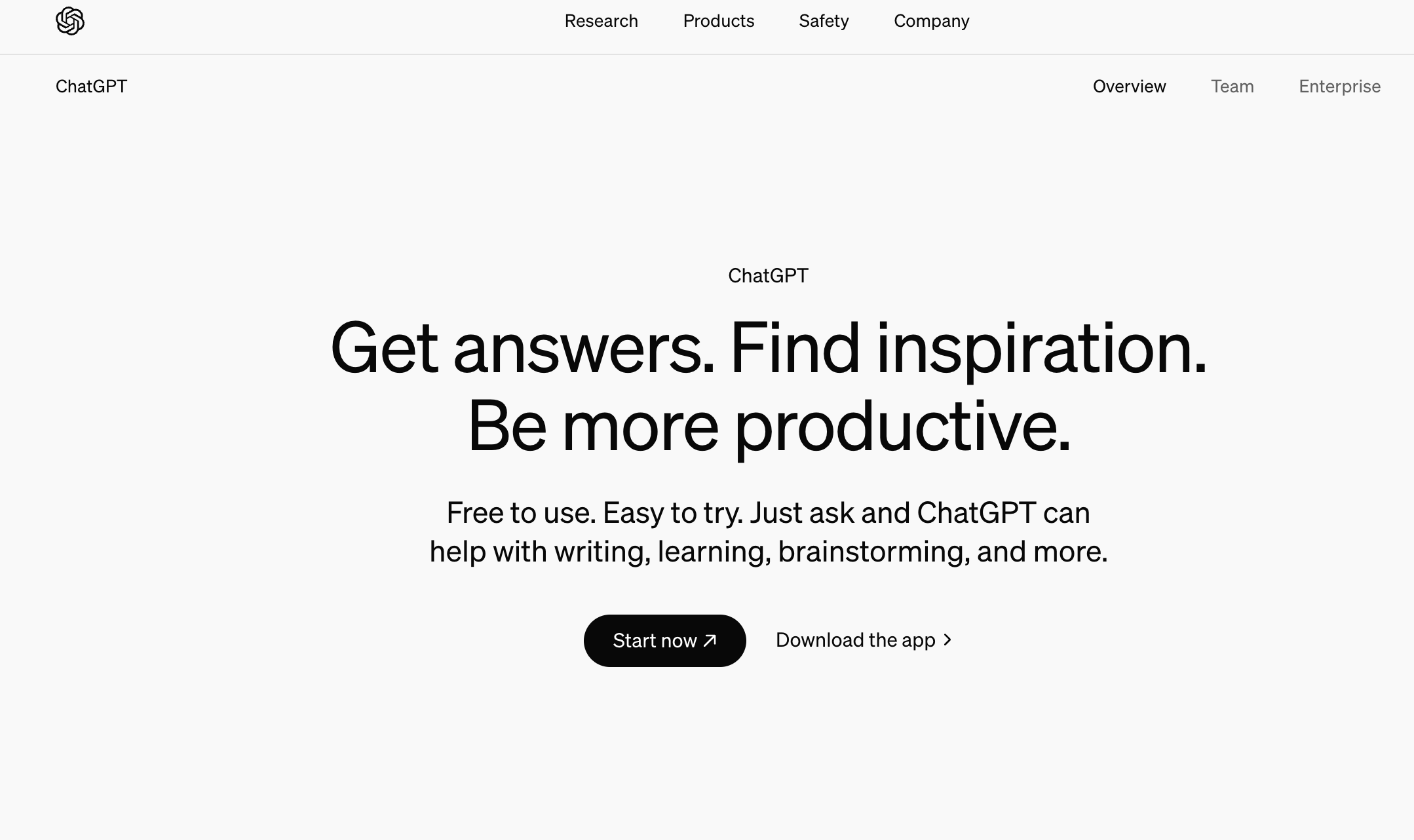
Developed by Open AI, ChatGPT 4 is a language model that generates output based on the input. Like most generative models, this generative AI focuses on creating new content from data training and machine learning.
You might ask "Is ChatGPT predictive AI?"
Well, yes and no. ChatGPT is primarily a generative AI model - it excels at content generation based on its data training. But it indirectly has some of the features of predictive AI tools. By using natural language processing (NLP) features, you can adapt ChatGPT for predictive forecasting by analyzing and interpreting data.
Here are some ways to use it:
- Data analysis
- Scenario generation
- Reporting and insights
- Sentiment and trend analysis
Now let's explore predictive AI.
What is Predictive AI?
Another valuable type of AI tool is predictive AI. Similar to generative AI, it also analyzes and learns from the patterns of the data, but it is mostly based on historical data pre-loaded into it. AI applications with predictive models are specifically used for predictive analytics and predictive AI forecasts .
Predictive AI technologies are especially useful for most of the data analysts, as they use it to make critical decisions for the future. This, in turn, helps the companies analyze the possible outcomes and prepare for them.
How Does Predictive AI Work?
For making any predictions, a huge amount of data is required for the AI's training. The training is done through advanced machine learning technologies, and the huge data is called ‘BIG DATA’. The tool learns from big data.
For example, the survey results of thousands of customers about the stock they are buying - which can provide predictions for users about the best stock available based on demand. Similarly, big data helps to provide prediction results that are almost accurate. The tool analyzes a lot of factors before publishing the results, which makes it more efficient.
What Are the Advantages of Predictive AI?
1. Getting insight into future trends
It helps in getting insights into future trends, which helps users know things in advance and plan and act accordingly.
2. Accuracy in forecasting
Predictive AI works with a large number of datasets. As a result, we can expect an excellent rate of accuracy.
What Are the Disadvantages of Predictive AI?
1. Data quality
The tool is equipped with a lot of data and keeps learning the patterns. But it cannot verify the quality of the data. As a result, this hampers the prediction accuracy to some extent. Since predictive AI utilizes the data it gets to create forecasts, the quality of the predictive AI analysis is directly proportional to the quality of the data it gets.
2. Complex algorithms
The machine learning algorithms used by the tool are complex, which increases the maintenance cost.
Example of Predictive AI: IBM Watson Predictive Analytics
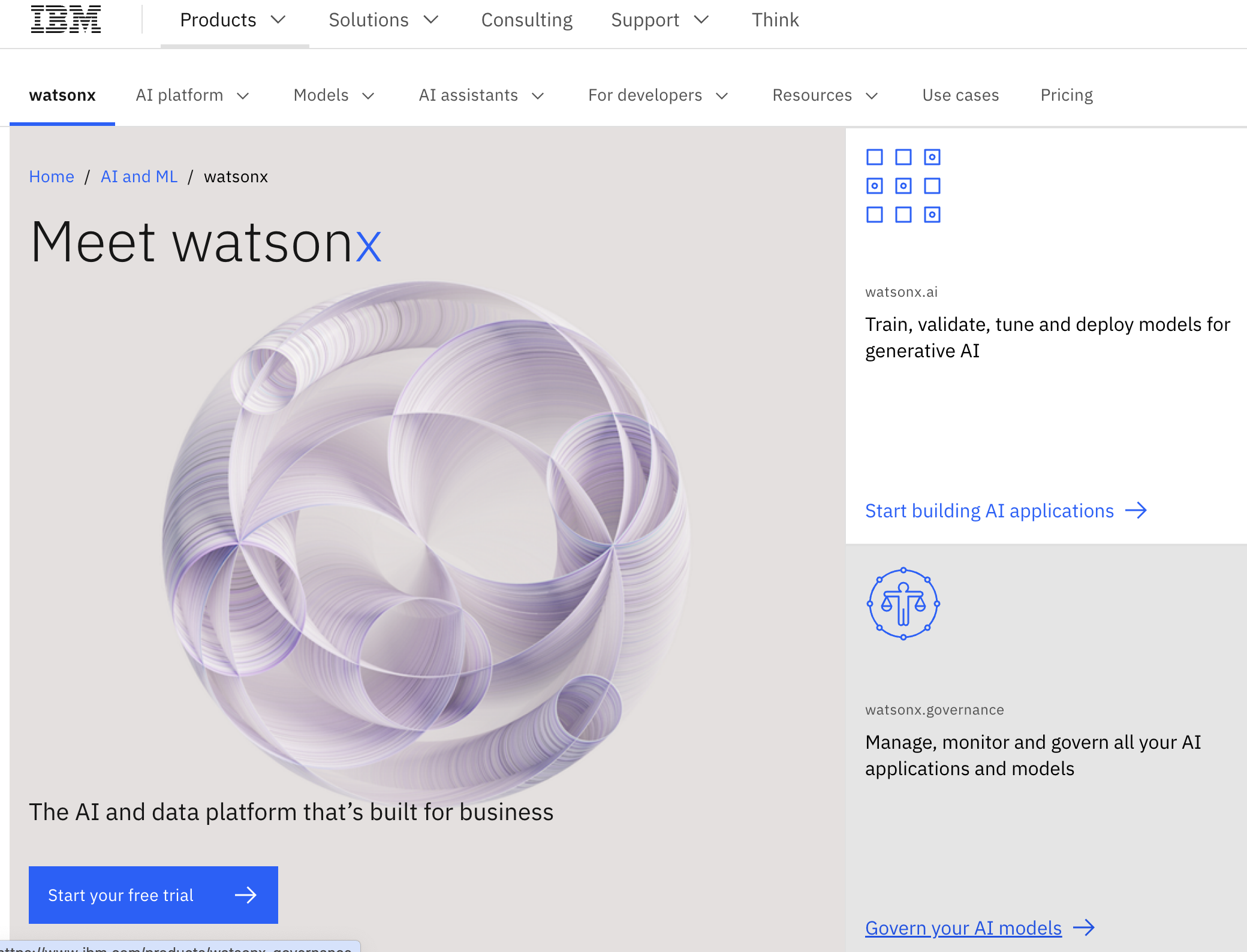
IBM's predictive analysis tool IBM Watson uses dynamic machine learning algorithms to predict future events using historical data. What is IBM Watson Analytics used for? It is used in retail, finance, and healthcare to predict future events, improve decision-making processes, and operations.
Both generative and predictive AI are immensely popular in their own way. While the tools are similar when it comes to the working process, there are some differences between generative AI vs predictive AI.
5 Differences Between Generative AI vs Predictive AI
1. Main use - For generative AI, it is to create unique content; be it image content, video content, or text content. On the other hand, predictive AI focuses on providing predictions for future events.
2. Technologies - Generative AI and predictive AI are different. Generative AI uses technologies like GANs and neural networks, while predictive AI uses complex machine learning algorithms and statistics to produce results.
3. User input - Generative AI starts producing results, i.e., high-quality content with just a prompt. Predictive AI relies on statistical data to analyze and predict future outcomes.
4. Results - The results of generative AI are long content, unique images, or videos. But the results of predictive AI are future predictions required for market trends.
5. Use cases - Generative AI is mostly used in e-commerce sites, digital marketing, and other corporate uses. Predictive AI finds its usage in healthcare industries, finance companies, and stock market brokers.
Generative AI vs Predictive AI: Wrapping Up
The development of AI models and tools, like generative and predictive AI, offers many use cases and benefits. Both types of AI are useful and unique, successfully capturing their target audience.
While these tools have some demerits, developers constantly strive to make them more efficient. We can expect future versions of these tools to be more feature-rich and user-friendly.
Start 14 Days free trial
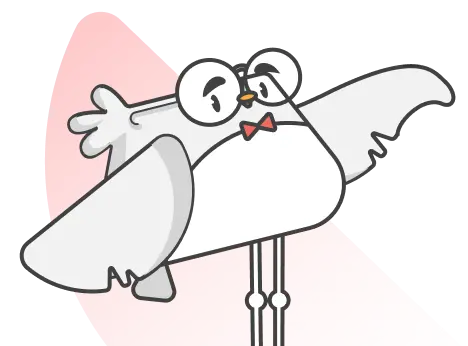
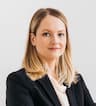
Kate William
Related Articles
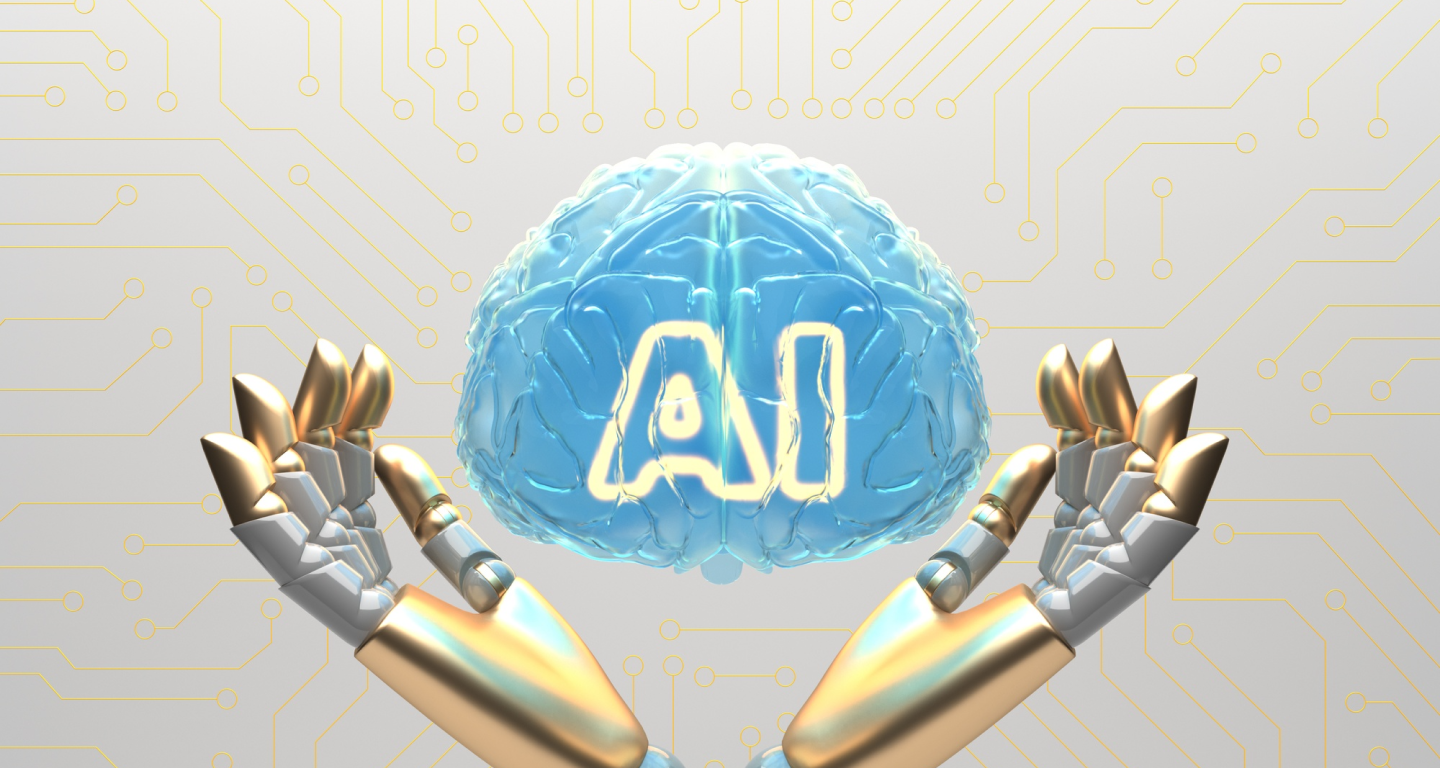
General
The Power of AI in Marketing: Applications, Pros, and Future Trends
9 MINUTES
18 July 2023
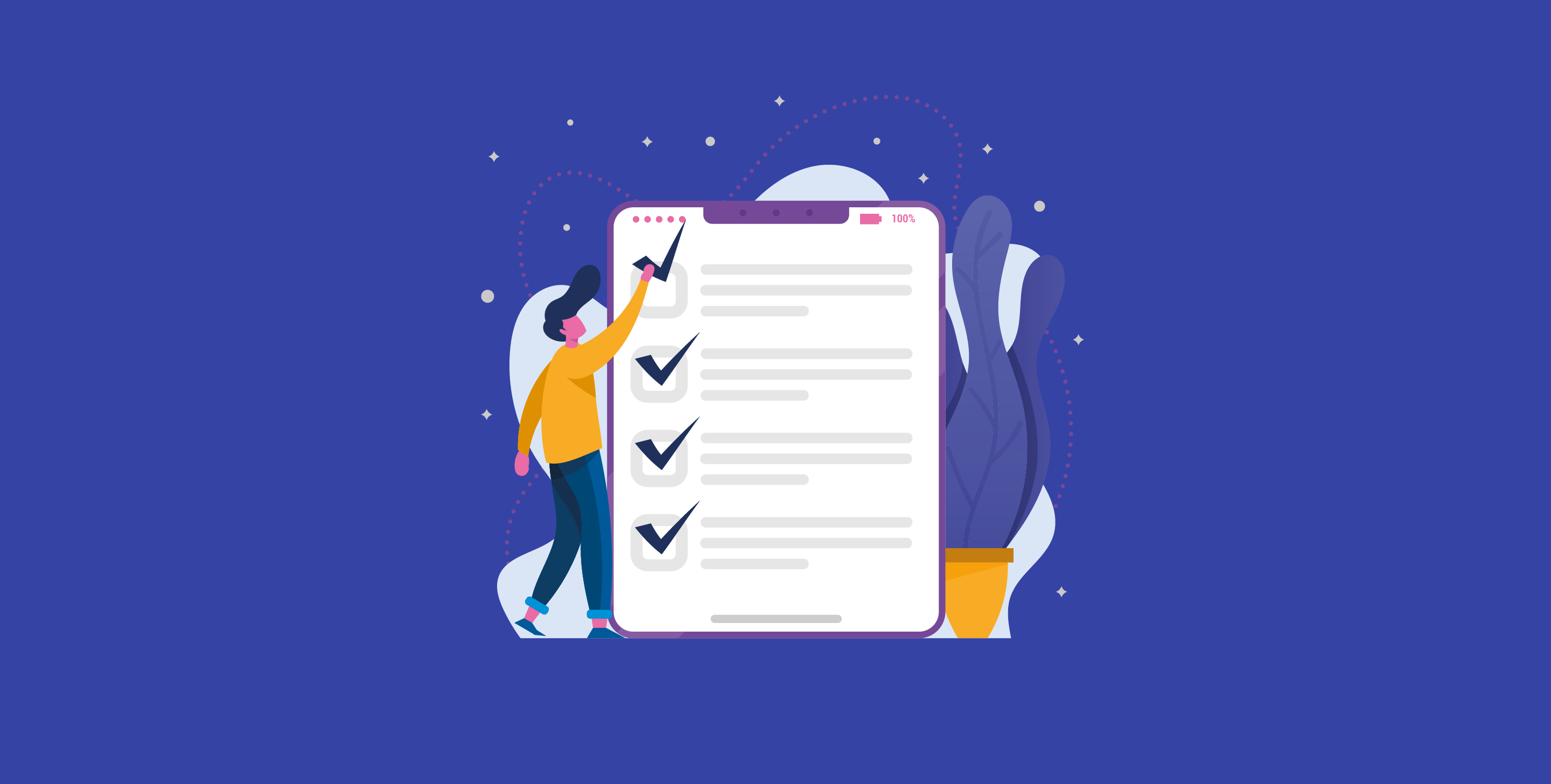
Best Of
How To Increase Sales Using Surveys: A Guide
16 MINUTES
25 September 2020
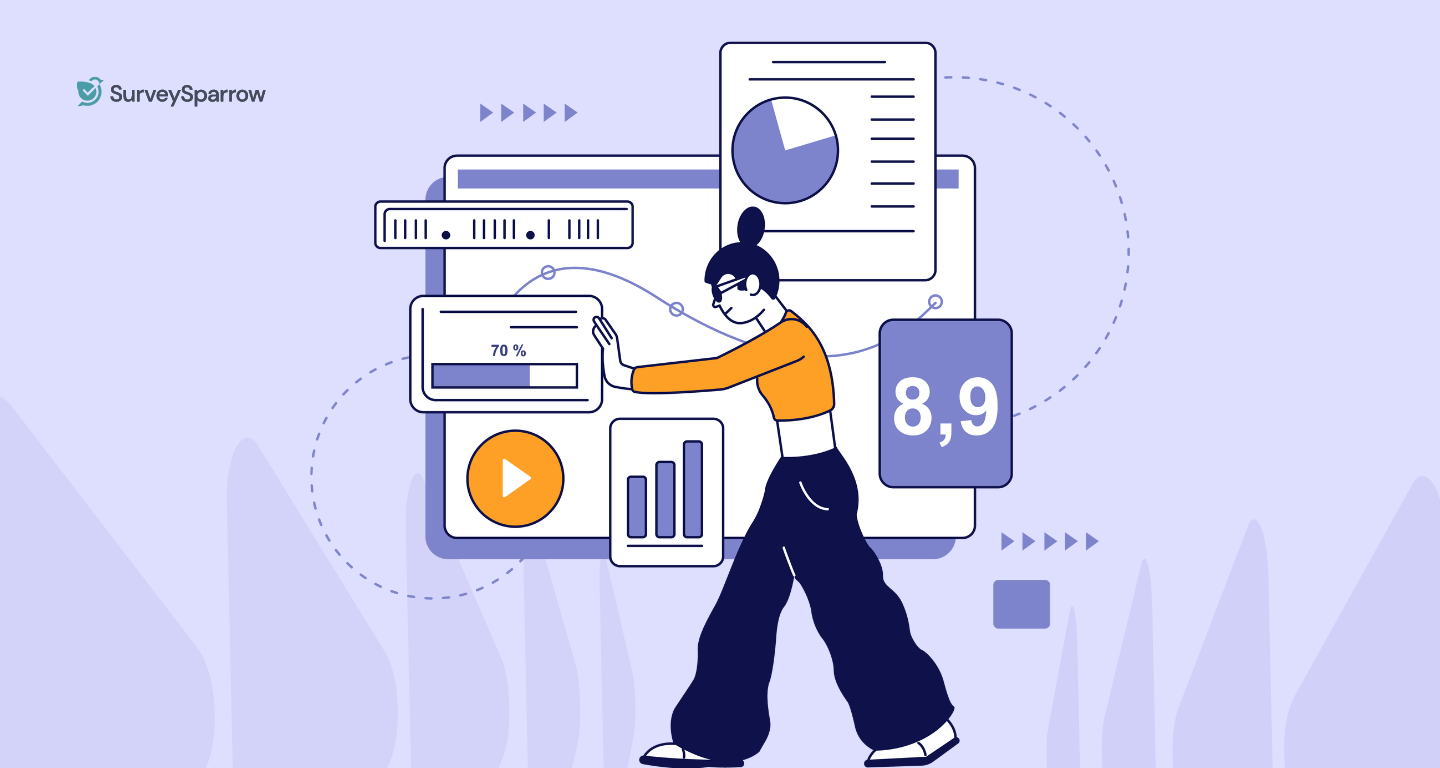
Knowledge
What is a Digital Experience Platform (DXP)? How do You Choose One?
8 MINUTES
6 July 2024
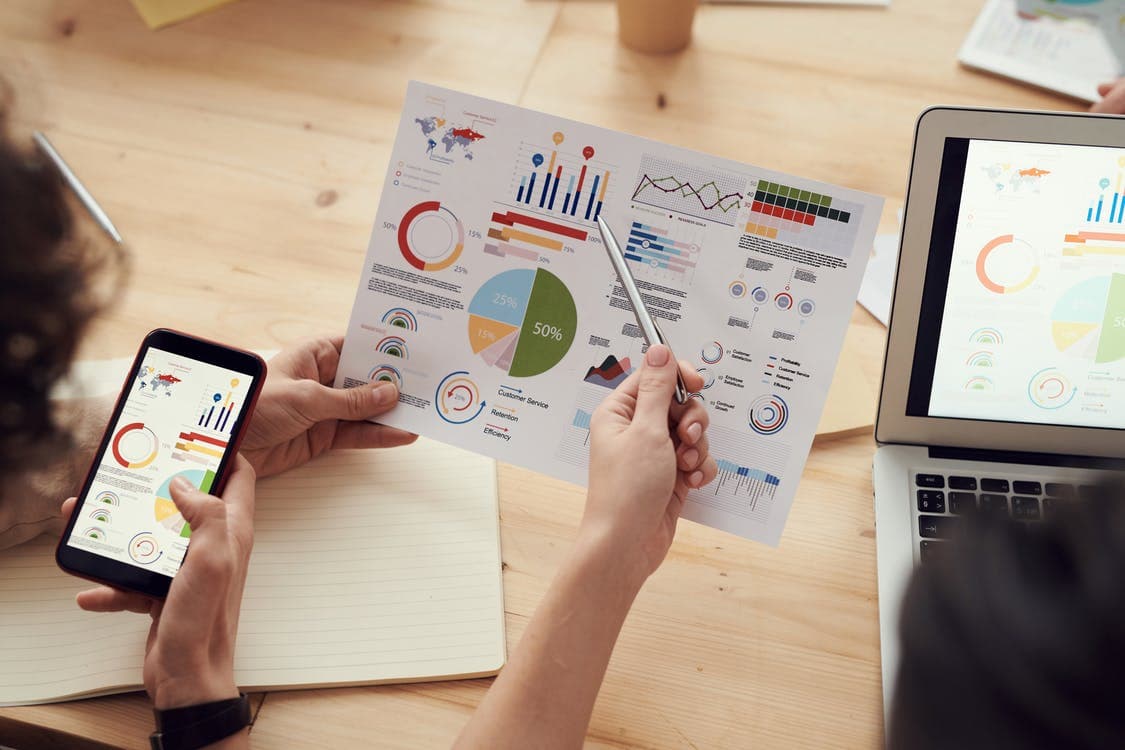
Best Of
Sampling Errors: Avoid These 7 Sampling Errors At Any Cost!
15 MINUTES
10 April 2022