Best Of
Cluster Sampling: A 4-Step Guide with Examples

Article written by Pragadeesh Natarajan
pragadeesh
8 min read
11 September 2024
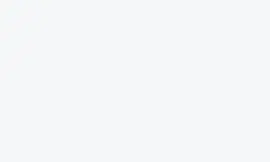
Cluster sampling in statistics allows you to collect the right representative data from a large target audience. Collecting accurate data from a large population is hard, and this is where cluster sampling comes in to ease things up.
Here’s what we’ll cover about the topic. Feel free to jump to a relevant section:
- What is cluster sampling?
- How to conduct cluster sampling
- Types of cluster sampling
- Applications of cluster sampling
- Advantages and disadvantages of cluster sampling
- FAQs
What is Cluster Sampling?
Cluster sampling divides a large target group into multiple smaller groups or clusters for research purposes. Researchers then form a sample by randomly selecting these clusters.
The random selection gives every group in that target population an equal chance to be a part of the sample group. However, only a few relevant groups were selected, and the rest were eliminated.
We use a cluster sample to study large populations. Typically, clusters are obtained from pre-existing groups such as schools or cities.
Then, we group the samples together by certain shared characteristics or attributes. Samples include multiple attributes such as demographics, goals, habits, backgrounds, etc.
Providing more attributes helps you accurately target the right group that could give you accurate feedback. Instead of selecting the entire population, researchers pick a smaller, more productive group within that population to research and collect data.
Cluster Sampling Example
If you’re looking to conduct a survey on the performance of smartphones in the United States, you can divide America’s population into certain popular cities such as New York or Los Angeles.
You can then target those individuals who own smartphones in the respective cities and who use a certain kind of mobile OS. All of the above are valid attributes that would help you target the right group of people to research.
How to Conduct Cluster Sampling in 4 Simple Steps
Here’s how to conduct single-stage cluster sampling and find the correct representative sample:
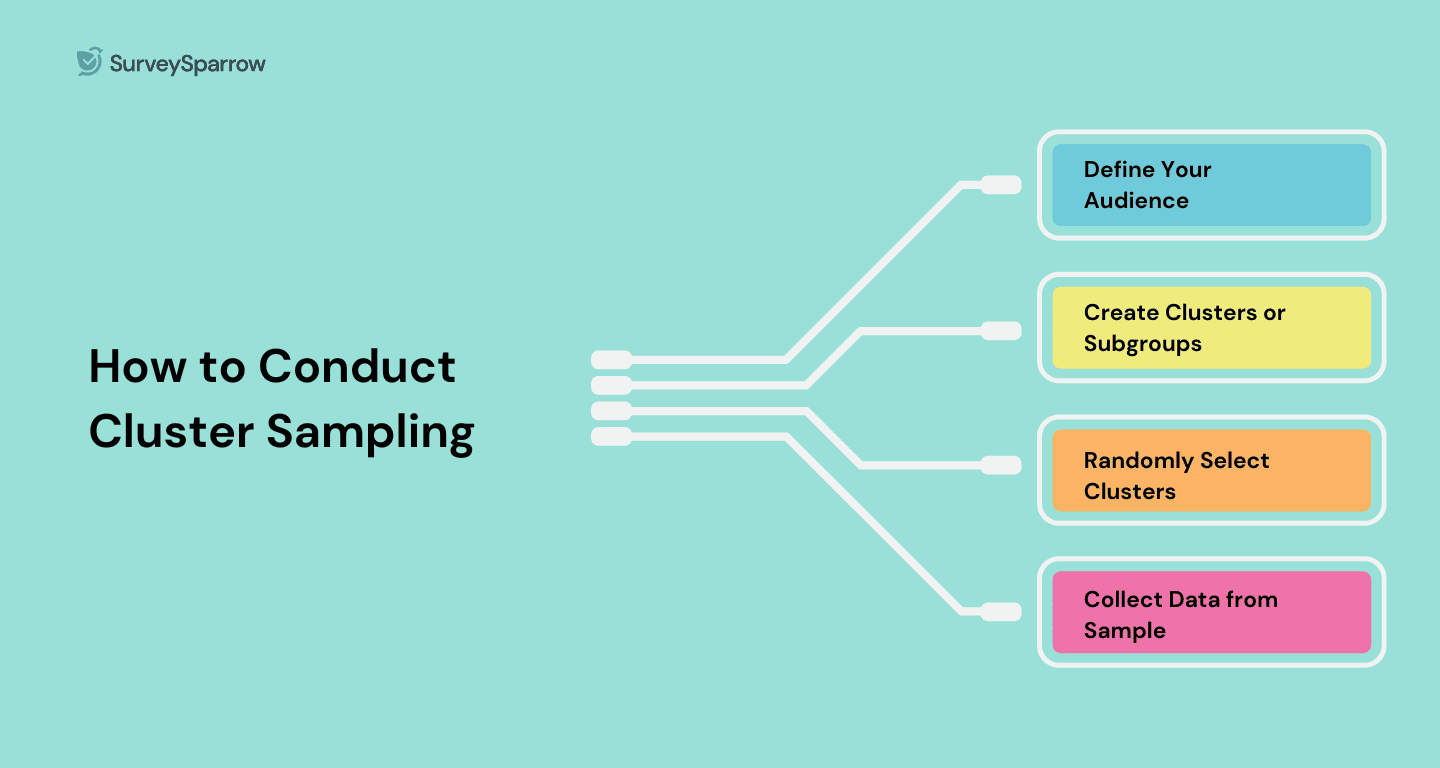
Step 1: Define Your Audience
Decide on your target population and desired sample size.
Step 2: Create Clusters or Subgroups
Now, divide your target population into smaller subgroups or clusters based on specific criteria. Getting this step right is crucial as it affects the quality of your segment or cluster and how well it represents your target population.
Step 3: Randomly Select Your Clusters
Pick a cluster or group that closely resembles the audience that you’re looking to research. You can pick a cluster based on a method of random selection. Make sure to keep the sample size in mind while you select one.
Step 4: Collect Data from the Sample
Finally, conduct your research and collect data from your selected clusters.
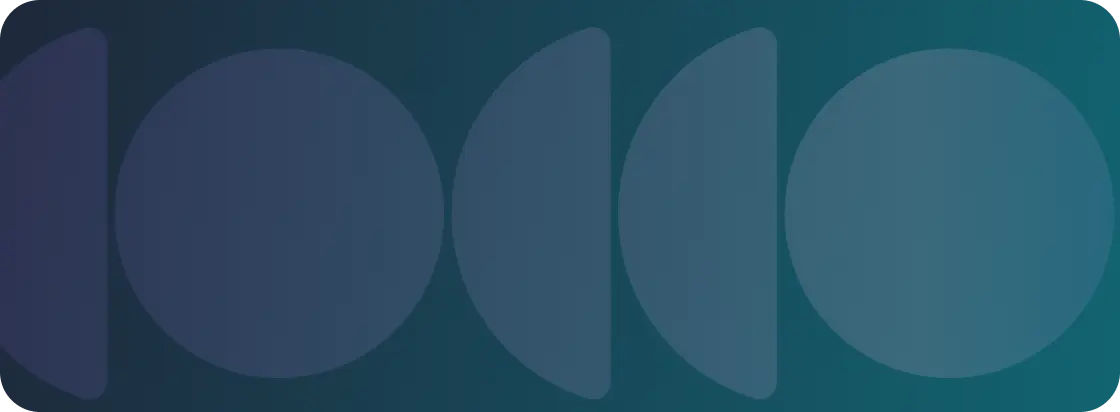
Maximize Your Survey Data with AI!
A personalized walkthrough by our experts. No strings attached!
3 Types of Cluster Sampling
There are mainly 3 types of cluster sampling you should know about. They are as follows.
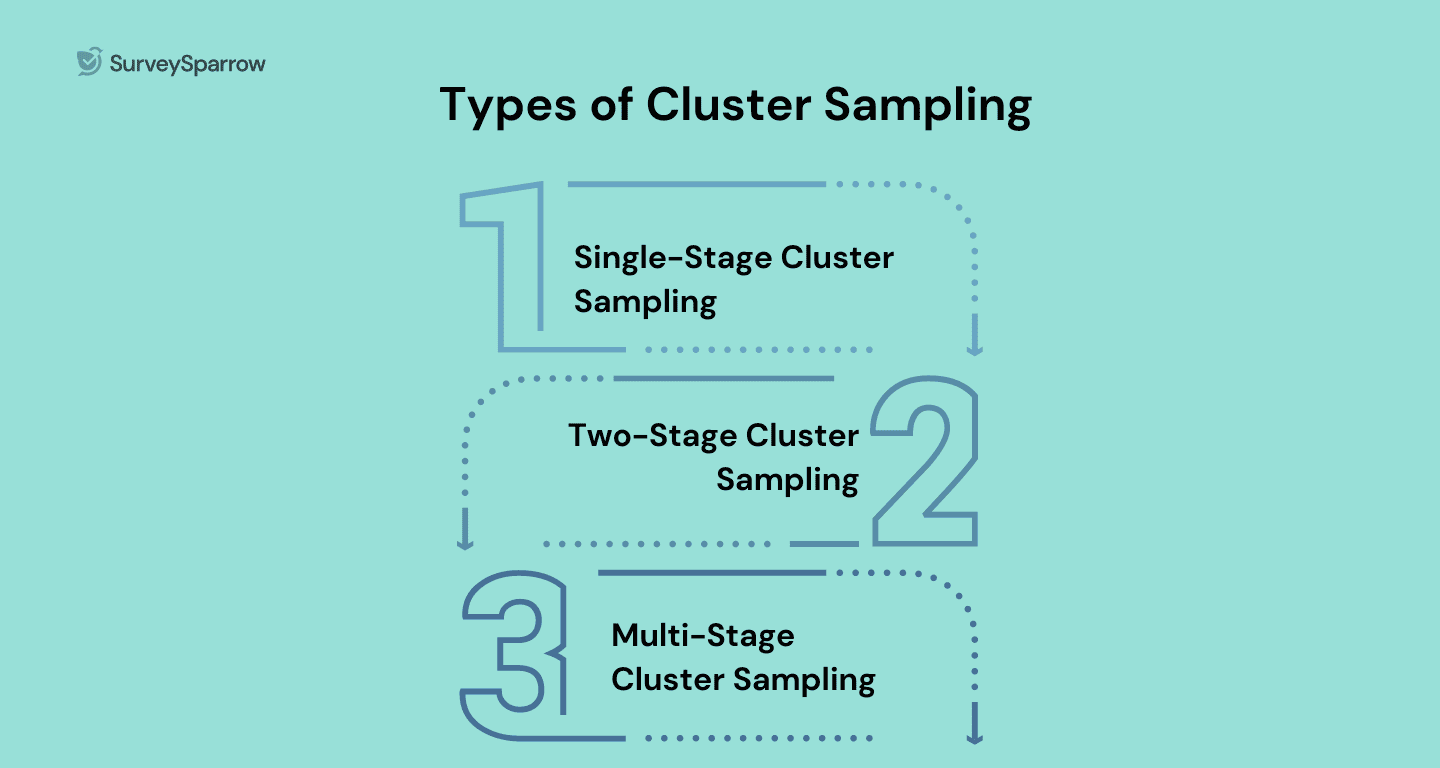
1. Single-Stage Cluster Sampling
In single-stage cluster sampling, entire clusters are randomly selected. And for the data, it's collected from every member within those selected clusters. This method is efficient when the population is geographically dispersed.
For example, suppose a researcher wants to study a population across several states. In this case, they might randomly select a few states and survey all individuals within those states.
2. Two-Stage Cluster Sampling
Two-stage cluster sampling involves two levels of selection. Firstly, the researchers randomly select clusters, and then they randomly select individuals within them. This method allows for a more manageable sample size while still providing a representative sample.
For instance, a study might first choose several cities, and then randomly select households within those cities to survey.
3. Multi-Stage Cluster Sampling
As the name indicates, it allows for multiple levels of clustering. Researchers may first select large clusters, then smaller sub-clusters with those. This process continues until it reaches a manageable sample size.
Multi-stage cluster sampling is particularly useful for populations with a hierarchical structure. For example, a study randomly selected countries, then the states, and then the villages within the countries.
6 Major Applications of Cluster Sampling
Here are some common applications of cluster sampling.
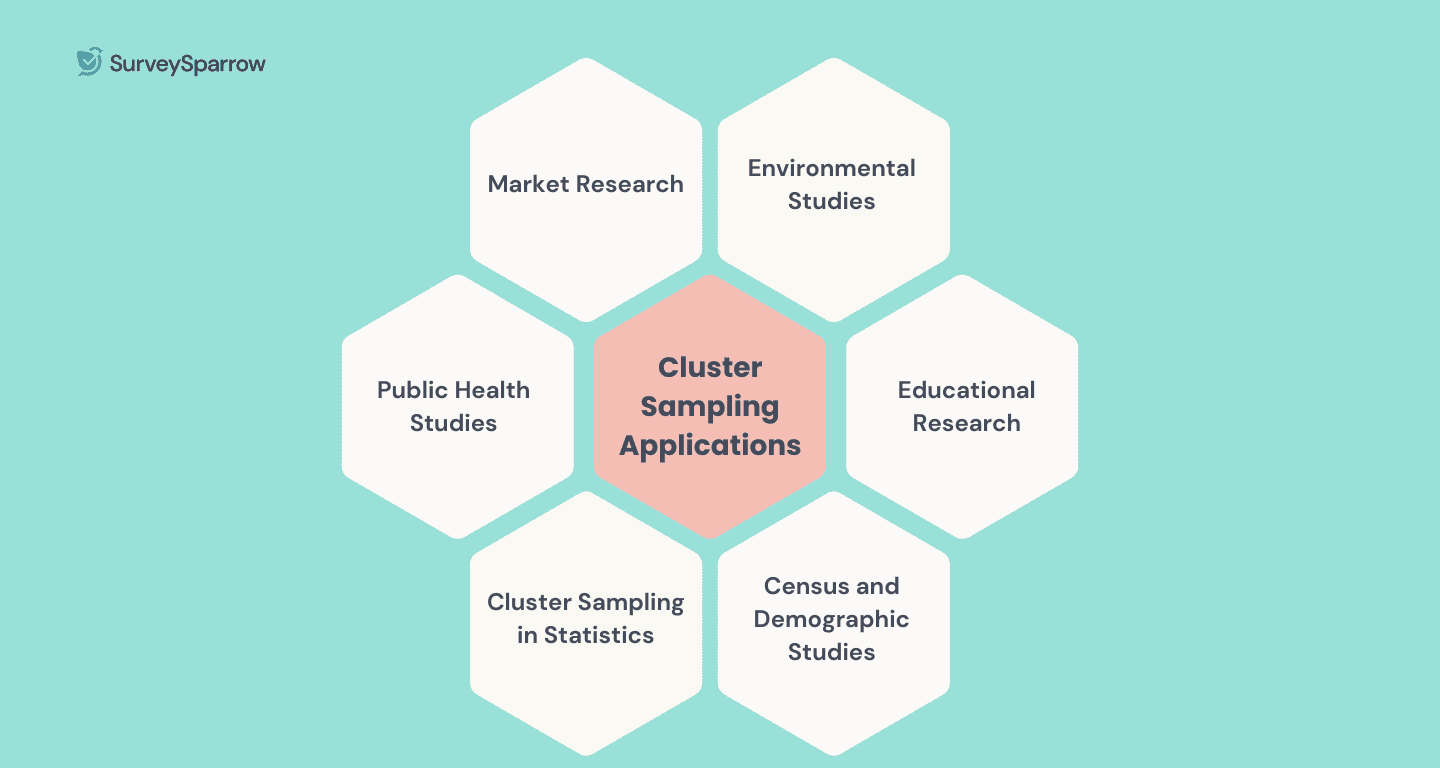
1. Market Research
Cluster sampling is frequently employed in market research. It's used to collect customer insights from specific geographical regions. By focusing on selected clusters, businesses can efficiently assess customer preferences, satisfaction, and more. All of this is possible without the need to survey the entire market.
2. Public Health Studies
In public health, this sampling method is used for rapid assessments of community health behaviors and needs.
For example, researchers might divide a city first into neighborhoods (clusters). Afterward, they randomly select some to survey about health practices, vaccination rates, or disease prevalence. This is highly helpful in emergencies or resource-limited settings where complete data collection is impossible.
3. Educational Research
Educational studies often utilize cluster sampling to evaluate interventions or programs within schools.
The clusters here are the schools. So, researchers will start by selecting the schools and then survey all students. This way, they can gather insights into educational outcomes. A tailored one can collect the effectiveness of teaching methods across different demographics.
4. Environmental Studies
Cluster sampling is used here to assess ecological data across large areas. Here, the clusters are specific regions or habitats. The researchers can gather data on biodiversity, pollution levels, or resource usage without the need to survey the entire ecosystem.
5. Census and Demographic Studies
Another area where cluster sampling is often applied is in demographic surveys and censuses. By selecting clusters of households or individuals, statisticians can estimate population characteristics and trends.
6. Cluster Sampling in Statistics
Statisticians use clusters as a practical sampling method for research. For instance, you use it during a natural disaster as it is totally impractical to collect data from every single person affected by the disaster.
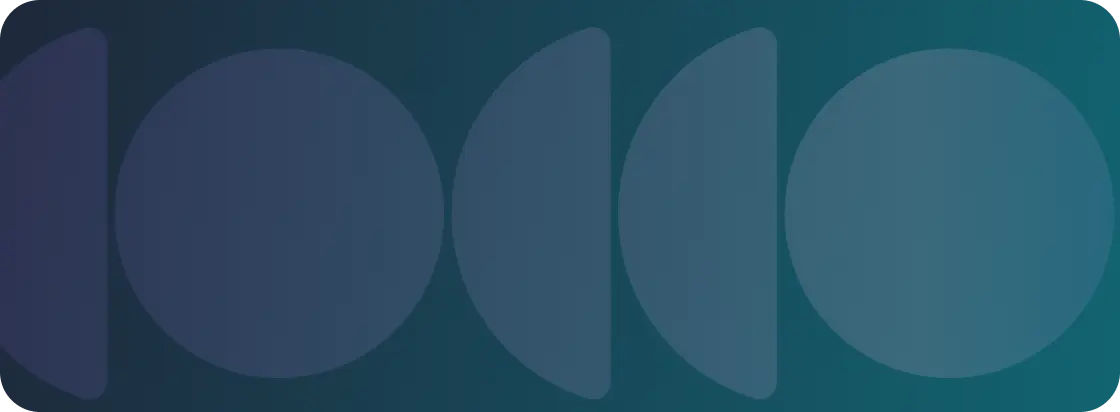
Maximize Your Survey Data with AI!
A personalized walkthrough by our experts. No strings attached!
Advantages and Disadvantages of Cluster Sampling
Like every other sampling method, cluster sampling also has its advantages and disadvantages. Let's have a look at them.
Advantages of Cluster Sampling
1. Time and cost-efficient:
Collecting data from a smaller number of people is more time and cost-efficient than collecting data from the target population as a whole.
2. Easy to implement
Compared to other probability sampling methods, a cluster sample is relatively easy to implement in practical situations.
3. High reliability
It can get you reliable, valid results when your target population is clustered properly.
Disadvantages of Cluster Sampling
1. Imprecise results
When you fail to put together groups that closely resemble your desired population, you’ll get results that aren’t accurate.
2. Difficult to analyze
It can become quite difficult to analyze when you’re dealing with different segments of people or clusters and their characteristics or attributes.
3. High sampling error
Cluster samples can be prone to high sampling error when not done right.
Final thoughts
In order to conduct an effective one, you need to come up with characteristics or attributes that accurately represent your target audience. Once you’ve nailed this part, it gets a lot easier to collect data that’s accurate and resembles the opinions of your target population.
Are you looking to use surveys to collect data from your selected clusters? SurveySparrow provides you with everything you need to create and conduct conversational surveys that your audience will love answering!
Conversational surveys typically tend to boost survey responses and create pleasant experiences that your customers will appreciate.
Most of your customers will complete your survey as they would be pleased to take surveys that are conversational in nature and are quite different from the long, boring forms you’ve seen on the internet.
Also, SurveySparrow provides you with a simple drag-and-drop interface and premade templates that anyone can use to create conversational surveys in minutes without having to start from scratch.
If you’re looking to boost your survey responses and create pleasant experiences, take the conversational way and try SurveySparrow today!
Have you got any questions on cluster analysis? Any interesting tips or hacks for conducting effective cluster analysis? Let us know in the comment section below.
If you’re wondering whether SurveySparrow is the right fit for you and would rather have someone walk you through our platform, reach out to us for a free, personalized demo!
Or sign up and try it out for free!
A personalized walkthrough by our experts. No strings attached!
Frequently Asked Questions (FAQs) about Cluster Sampling
What is cluster sampling?
It is a research approach that splits the target audience into multiple smaller groups or clusters to better target and collect accurate data.
What are the different types of cluster sampling?
There are three distinct types: single-stage, two-stage, and multi-stage. You can collect data from every unit (single-stage) or a random sample of units (multi-stage) within the selected clusters.
What are some advantages and disadvantages of cluster sampling?
It is time and cost-efficient, especially when targeting large samples. However, it provides average results as it is difficult to ensure that your clusters closely represent your target population as a whole.

Pragadeesh Natarajan
Related Articles
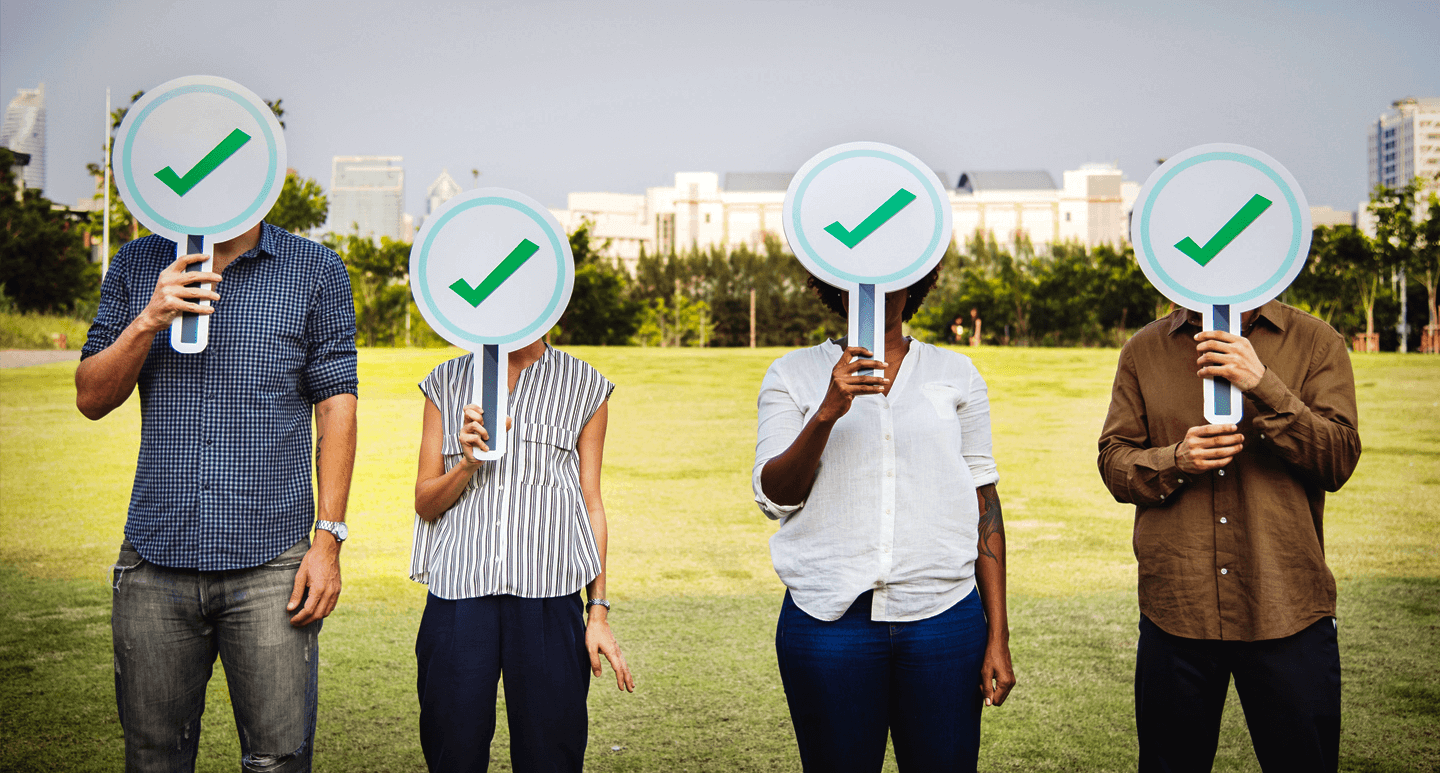
Survey Tips
All The Best 12 Exit Interview Questions You Should be Asking!
13 MINUTES
24 September 2018
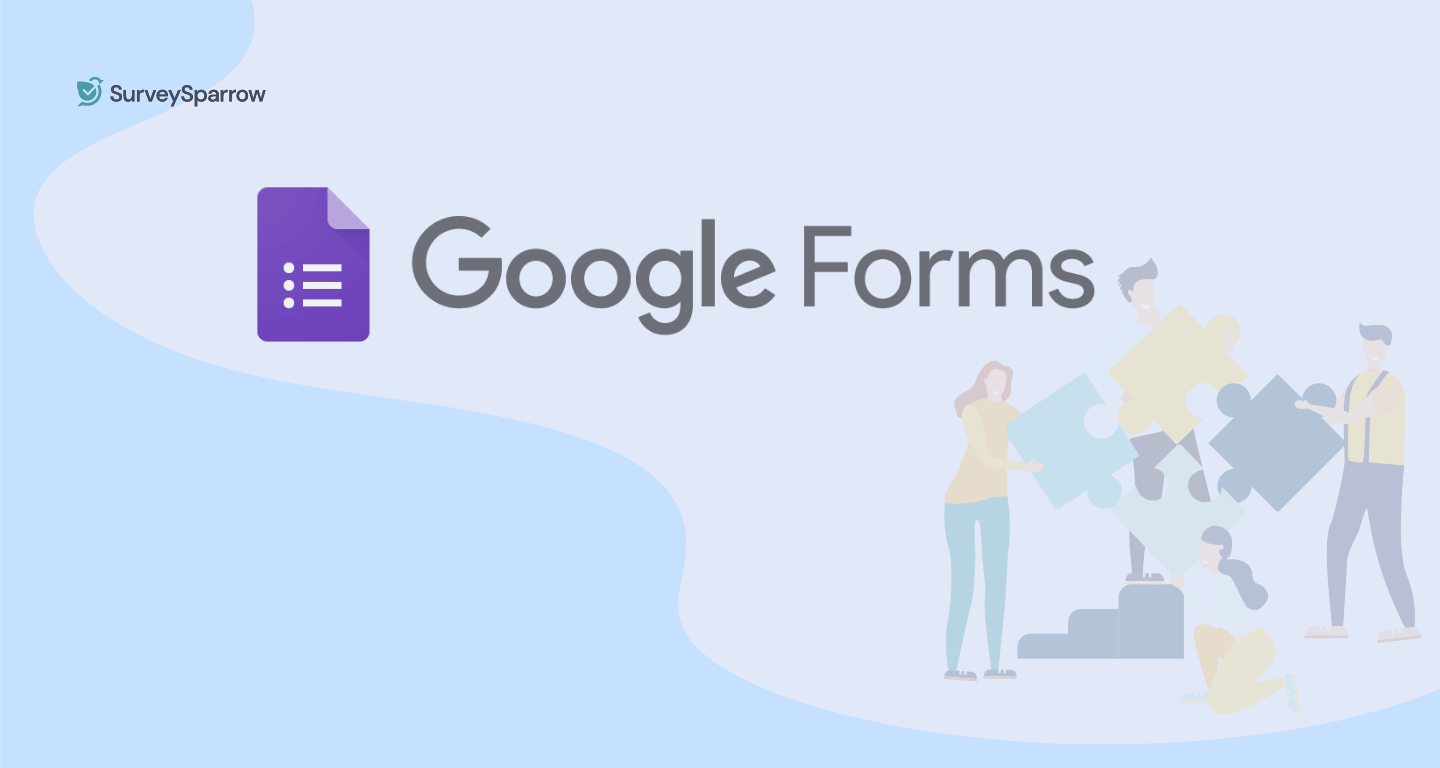
Knowledge
How to Make an Escape Room on Google Forms in Just 4 Steps
10 MINUTES
25 March 2024
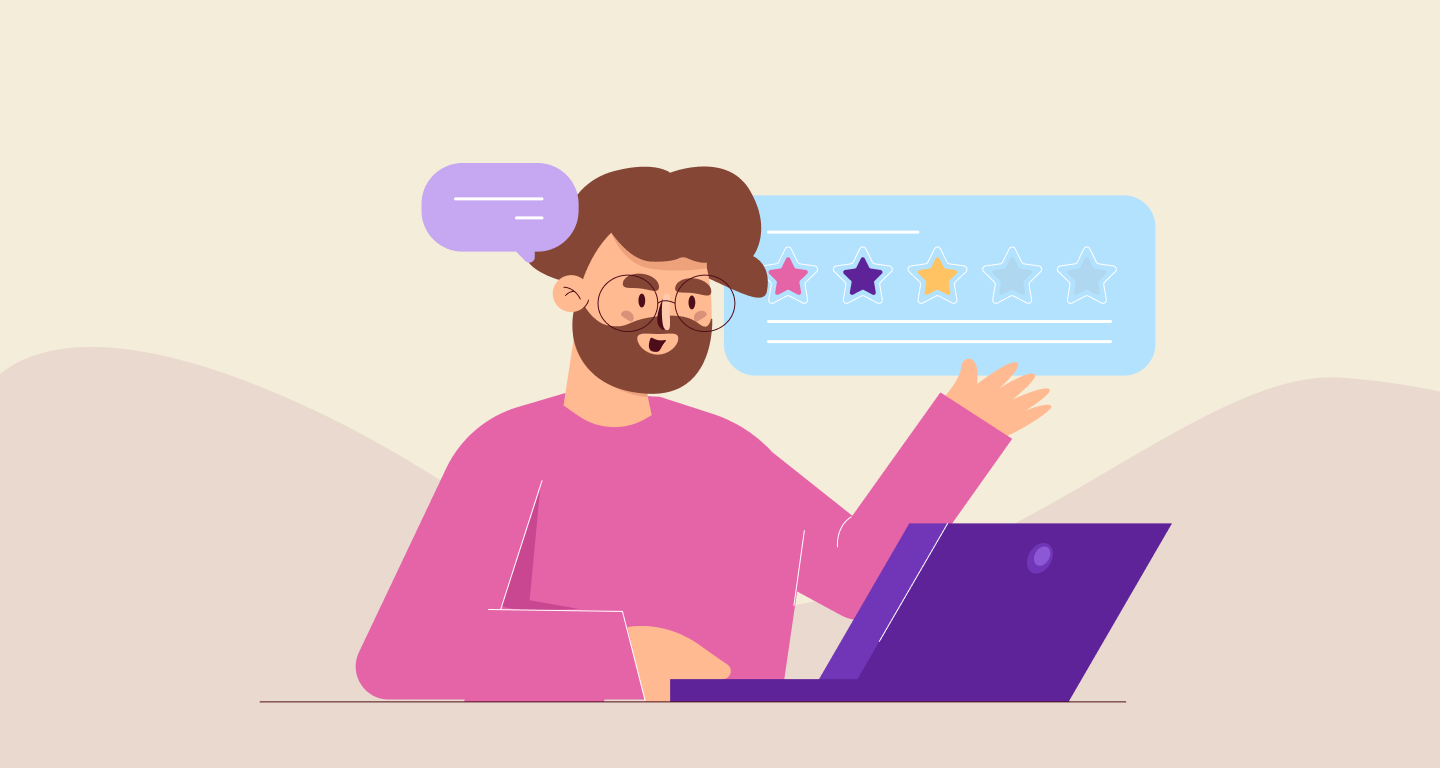
Customer Experience
The Role of a Customer Experience Manager: Exploring the Power of CX
11 MINUTES
30 June 2023
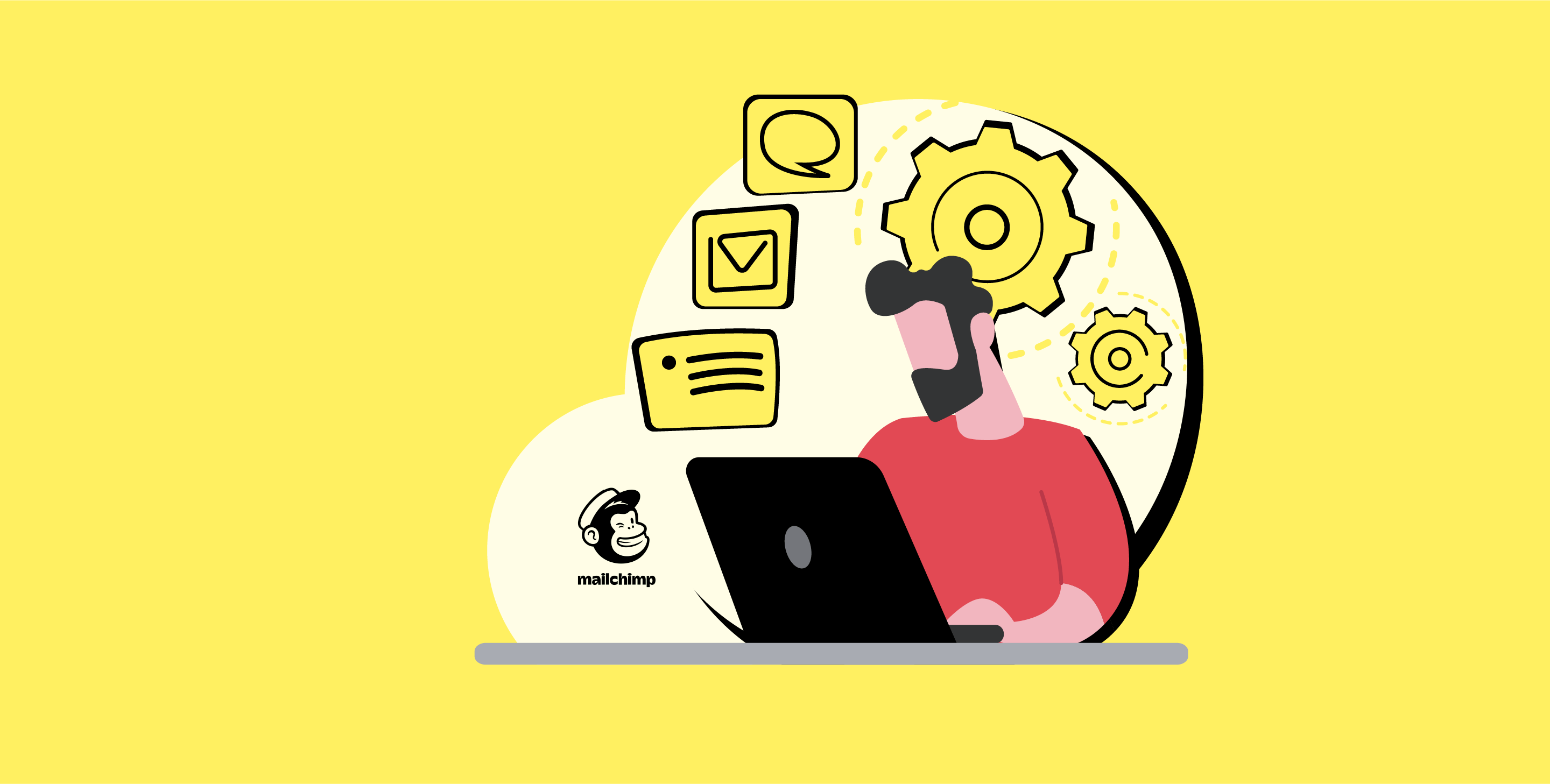
Compare
11 Must-Have Mailchimp Integrations for Marketers
9 MINUTES
21 November 2021