Best Of
Bivariate Analysis: What is it, Types + Examples

Article written by Aysha Muhammed
aysha
6 min read
2 March 2025
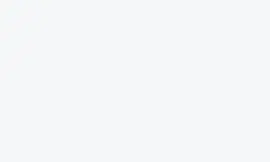
Numbers don’t lie, but they sure can be misleading—until you know how to interpret them.
Say your website traffic has doubled this month. Fantastic, right? But hold on. Are those extra visitors actually buying anything? Or are they just window shopping and bouncing off?
Or maybe your sales have skyrocketed. Great news! But… is it because of your latest email campaign? A price drop? Or just a seasonal trend?
This is where bivariate analysis comes in. It helps you move past surface-level stats and dig deeper into the real relationships between two variables. Because raw data is just numbers. But bivariate analysis? That’s insight.
In this blog, we’ll break down what bivariate analysis is, the different types, real-world examples, and, of course, how SurveySparrow makes it ridiculously easy to gather and analyze data that actually makes sense.
What is Bivariate Analysis?
Bivariate analysis is a statistical method that examines the relationship between two variables (hence, "bi-" meaning two). Unlike univariate analysis (which looks at a single variable), bivariate analysis helps determine whether there’s an association, correlation, or dependency between two factors.
For example, a business might want to know:
- Does increasing digital ad spend lead to higher sales?
- Is there a link between customer service response time and satisfaction scores?
- Do employees who work longer hours perform better, or does productivity decline past a certain point?
By analyzing these relationships, businesses, researchers, and analysts can move beyond guesswork and make data-driven decisions.
Why is Bivariate Analysis Important?
Understanding the relationship between two variables is about making sense of data to drive real-world decisions. Without it, you might be relying on intuition rather than evidence, which can be a costly mistake.
Bivariate analysis helps you:
- Identify trends and correlations that aren’t immediately obvious – Spotting patterns in data helps businesses and researchers anticipate behavior, market shifts, or operational efficiencies.
- Predict future outcomes based on past relationships – If there’s a historical link between two factors, bivariate analysis can help forecast what might happen next.
- Make strategic business decisions with greater confidence – Knowing which variables influence each other allows for data-driven decision-making rather than guesswork.
For example, if an eCommerce company notices that offering discounts leads to a spike in sales, they can incorporate strategic promotions into their pricing strategy. But they may also want to explore whether those discounts bring in new, long-term customers or just one-time buyers. A follow-up bivariate analysis comparing discounted vs. non-discounted repeat purchase rates would give them the insights they need.
In a SaaS business, understanding the relationship between customer engagement and retention is critical. If data shows that users who complete a product tutorial are significantly more likely to renew their subscriptions, then focusing on onboarding experiences and customer education can drive long-term growth.
Types of Bivariate Analysis (With Examples)
Bivariate analysis methods depend on the type of data being studied. The three main types are:
- Numerical vs. Numerical – When both variables are continuous numbers (e.g., revenue and ad spend).
- Categorical vs. Categorical – When both variables represent categories (e.g., customer satisfaction level and subscription type).
- Numerical vs. Categorical – When one variable is numerical and the other is categorical (e.g., salary levels across different job roles).
1. Numerical vs. Numerical (Correlation & Regression Analysis)
When both variables are numerical, the goal is to measure the strength and direction of their relationship. The two most common techniques used here are correlation analysis and regression analysis.
Correlation Analysis
Correlation analysis measures how closely two numerical variables move together. The correlation coefficient ranges from -1 to +1:
- A positive correlation means that as one variable increases, the other also increases (e.g., more social media engagement leads to higher sales).
- A negative correlation means that as one variable increases, the other decreases (e.g., higher workload might lead to lower job satisfaction).
- A zero correlation suggests no relationship between the two variables.
Regression Analysis
Regression goes a step further. While correlation tells you whether two variables are related, regression analysis helps predict outcomes.
For example, a real estate company may analyze the relationship between house prices and square footage. If they find that larger homes consistently sell for higher prices, they can create a regression model to predict the price of a home based on its size.
2. Categorical vs. Categorical (Chi-Square Test & Cross Tabulation)
When dealing with two categorical variables, bivariate analysis helps determine whether there is a significant association between them.
Chi-Square Test
The chi-square test is used to assess whether two categorical variables are independent or related.
For instance, a company might want to know if customer satisfaction varies by subscription type. If premium subscribers consistently report higher satisfaction than free-tier users, the business can tailor its offerings accordingly.
Cross-tabulation (or contingency tables) is another useful method in this category. It organizes data into a matrix format, making it easier to spot trends.
3. Numerical vs. Categorical (T-Test & ANOVA)
When one variable is numerical and the other is categorical, statistical tests like T-tests and ANOVA help determine whether there are significant differences between groups.
T-Test
A T-test is used when comparing the means of two groups. Suppose an eCommerce store wants to analyze whether the average order value differs between male and female customers. A T-test can confirm whether any observed difference is statistically significant or just random variation.
ANOVA (Analysis of Variance)
ANOVA is used when comparing three or more groups. For example, a company might analyze how customer satisfaction scores vary across different product categories. If ANOVA indicates a significant difference, the business can investigate further to understand why some categories perform better than others.
How to Perform Bivariate Analysis in SurveySparrow
With SurveySparrow’s advanced analytics, you don’t need to be a data scientist to perform bivariate analysis. Here’s how you can do it in just a few clicks:
- Step 1: Create a survey with numerical & categorical questions.
- Step 2: Use SurveySparrow’s real-time reporting to collect responses.
- Step 3: Apply filters & cross-tabulation to analyze relationships between two variables.
- Step 4: Export your data to Google Sheets or BI tools for deeper insights.
Pro Tip: Want to see trends over time? Use SurveySparrow’s recurring surveys to track changes in data!

Final Thoughts
Bivariate analysis is not just about numbers ; it’s about understanding connections that drive success. Whether you’re in marketing, customer experience, or research, identifying relationships between variables can help you optimize strategies, boost engagement, and drive growth.
And the best part? SurveySparrow makes the entire process seamless. From collecting feedback to analyzing trends, you get the insights you need—all in one place.
Ready to take your data analysis to the next level? Start your free trial with SurveySparrow today!
Start 14 Days free trial
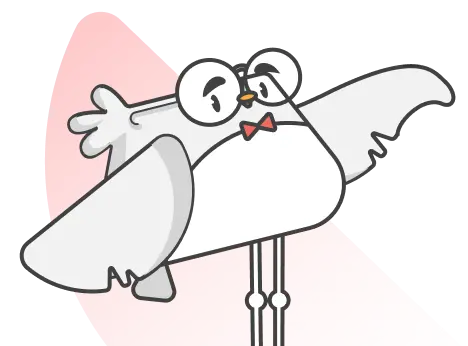

Aysha Muhammed
Part-time hermit and a full-time writer trying to survive the SaaS space, one blog at a time. Bylines in digital but always on deadline.
Related Articles
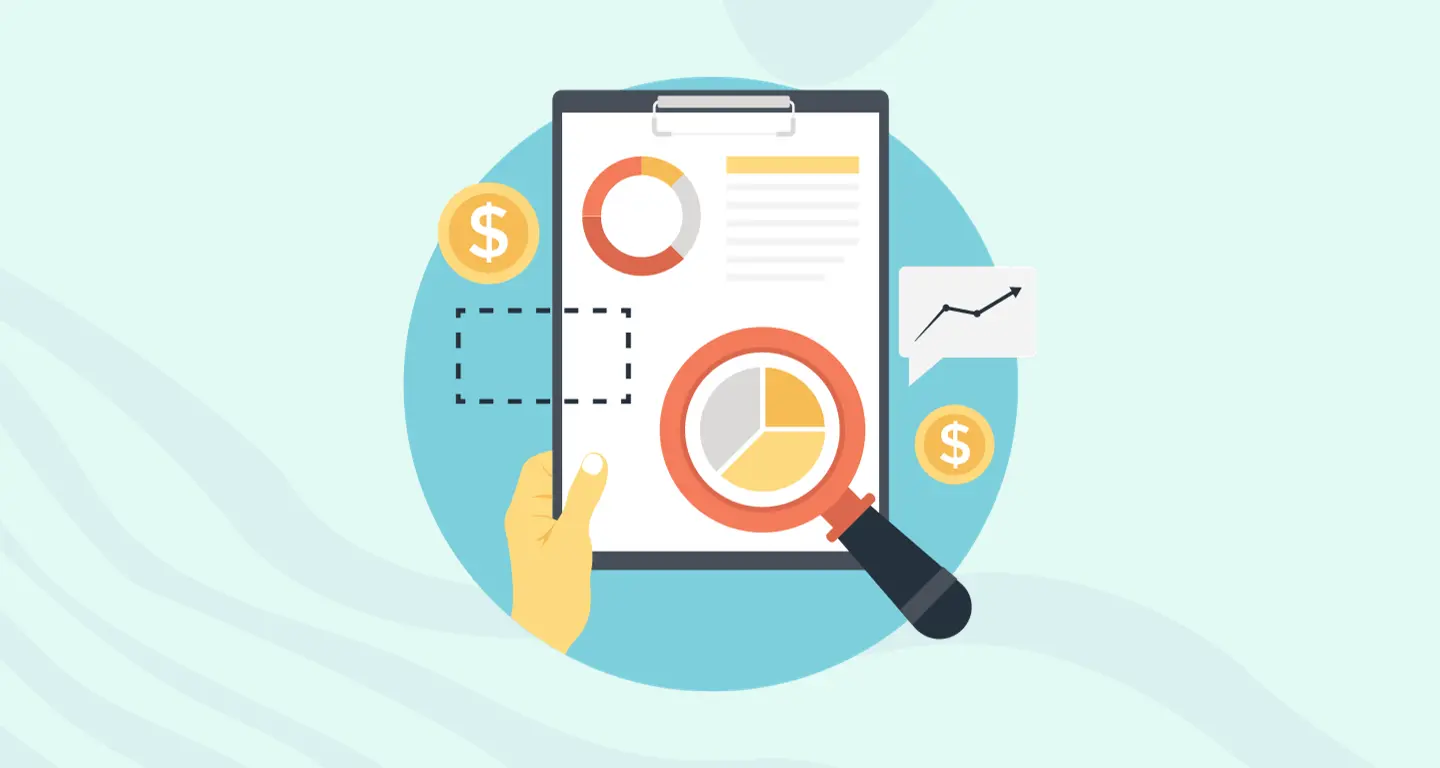
Knowledge
ANOVA: The Comprehensive Guide to Analysis of Variance
10 MINUTES
19 September 2023
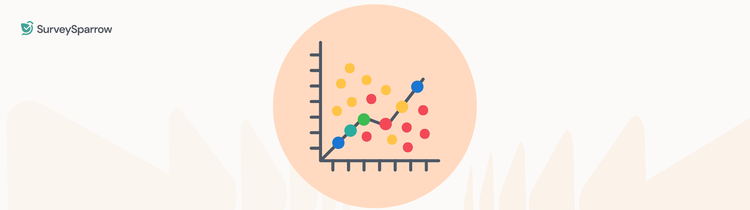
Knowledge
Correlation Analysis: Definition, Types, Examples
10 MINUTES
21 September 2024
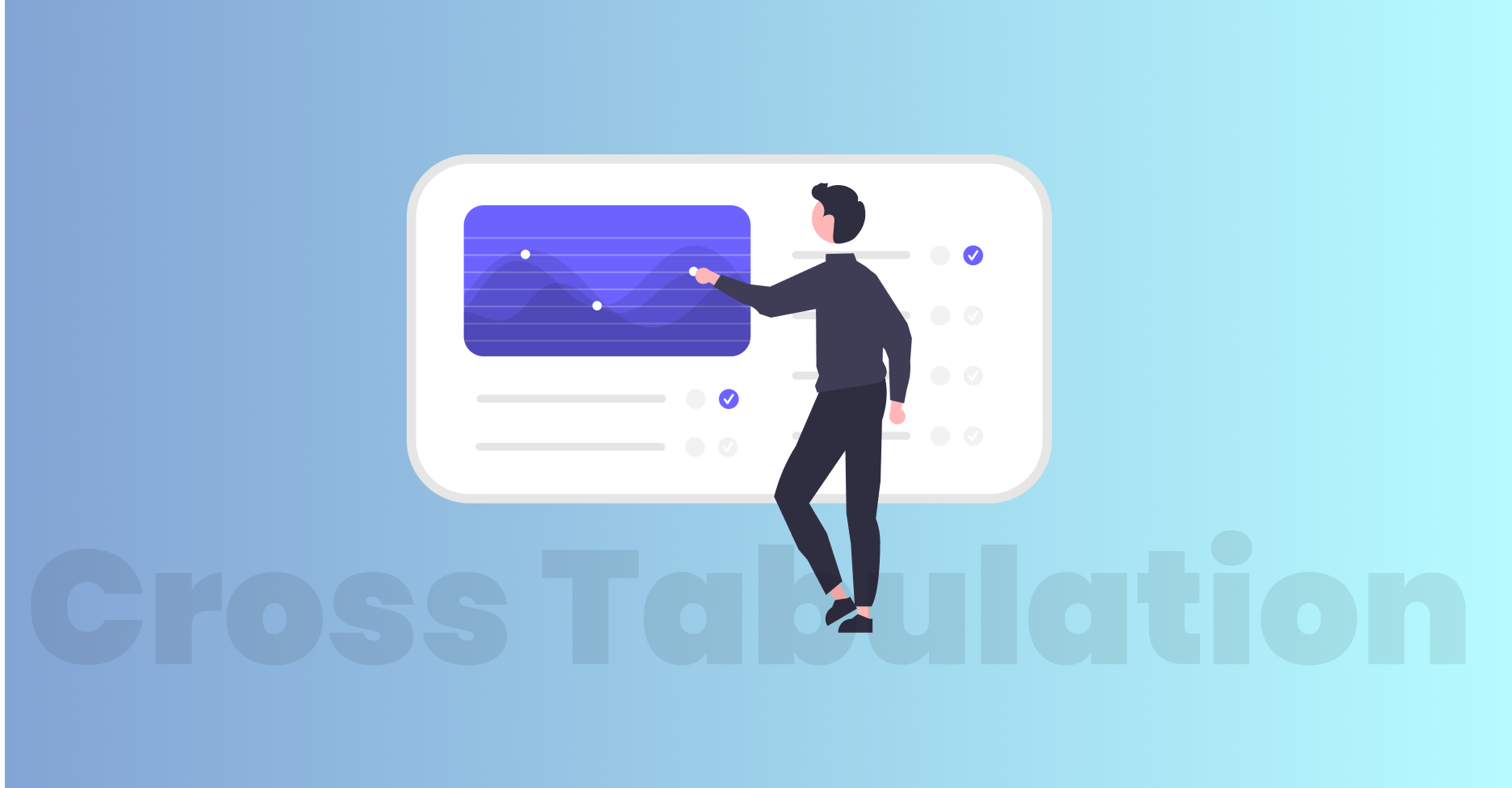
Best Of
Cross-Tabulation in Data Analysis : A Simplified Guide
13 MINUTES
29 June 2022
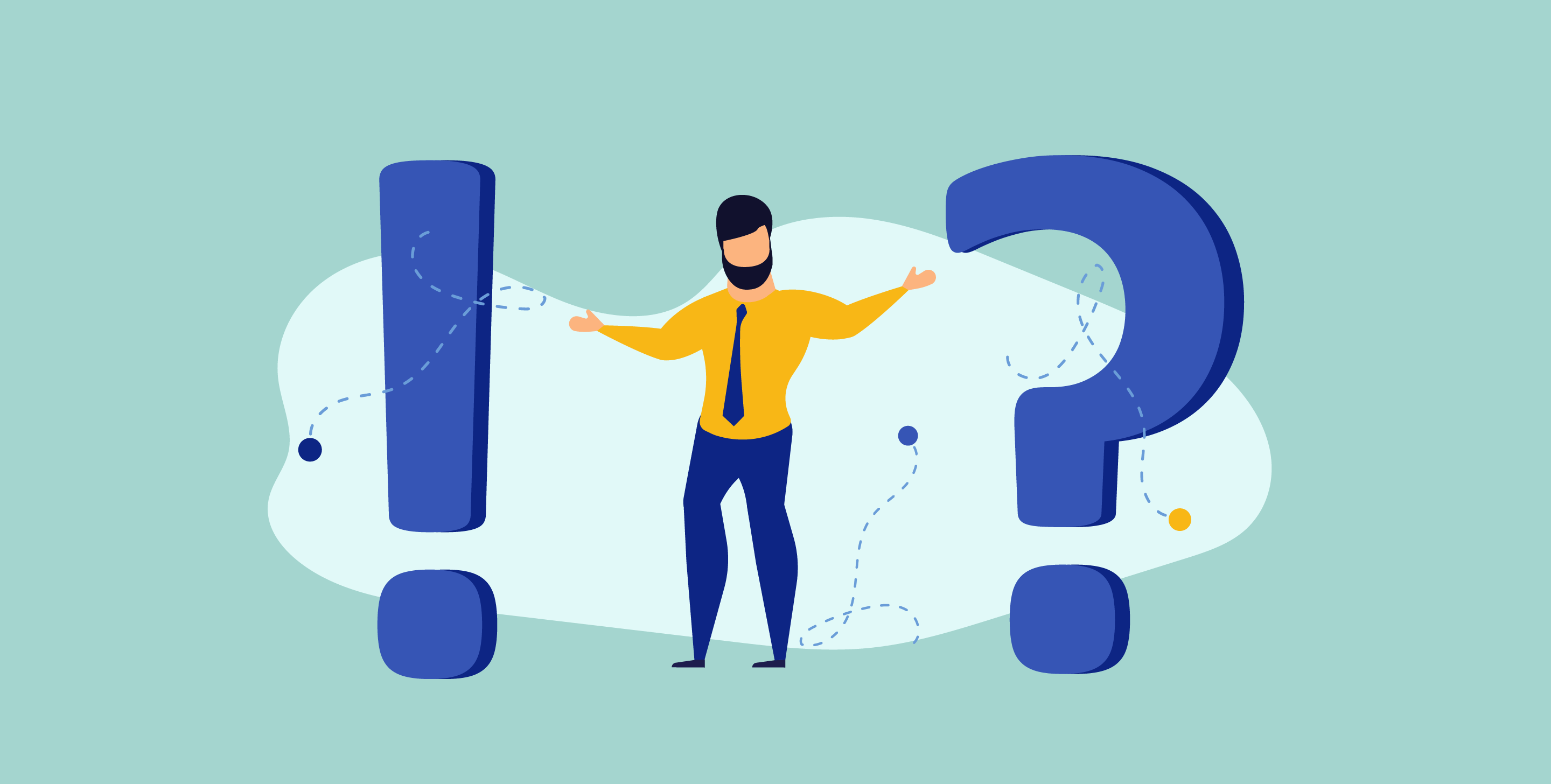
Best Of
12 Must Ask Questions for Your Customer Support Surveys
14 MINUTES
20 October 2020