Get The Real Insights You Deserve
Demo tailored to your business needs
Preview features you're interested in
Smart solutions from our experts
Confidence intervals are a vital statistical tool for estimating population parameters based on sample data. They provide a range of values within which the true population parameter is likely to fall. Here, we will delve into the intricacies of the confidence interval formula, explaining how to calculate confidence intervals and their significance in statistical analysis.
Whether you’re a student, researcher, or professional, understanding confidence intervals is crucial for making accurate inferences from data. So, let’s demystify the world of confidence intervals and explore the formula behind them.
What is a Confidence Interval?
Before we delve into the formula, let’s first clarify what a confidence interval is. A confidence interval is a range of values within which we can reasonably assume the true population parameter lies. It consists of two components: a point estimate and a margin of error.
The Confidence Interval Formula
The confidence interval formula is based on the principles of estimation and the distribution of sample statistics. For a population parameter, such as the mean or proportion, the general form of the confidence interval formula is,
CI = Point Estimate ± Margin of Error
Point Estimate
The point estimate is the best estimate of the population parameter derived from the sample data. For
instance, it could be the sample mean, proportion, difference in means, or any other relevant statistic.
Margin of Error
The margin of error accounts for the variability in the sample data and provides a measure of
uncertainty. It is typically calculated using the standard deviation of the sample, the sample size, and
the desired level of confidence.
Components of the Confidence Interval Formula
To gain a deeper understanding of the confidence interval formula, let’s examine its key components,
Standard Error
The standard error quantifies the variability in the sample statistic due to random sampling. It is
calculated by dividing the standard deviation of the sample by the square root of the sample size.
Z-Score or T-Score
The choice between the z-score or t-score depends on the sample size and the information available about
the population. Moreover, for large samples (typically above 30), the z-score is used, assuming a normal
distribution. Then, for small samples, the t-score is employed, taking into account the degrees of
freedom.
Critical Value
The critical value corresponds to the desired level of confidence. It is obtained from a standard normal
distribution or a t-distribution, depending on the sample size and assumptions made.
Calculating Confidence Intervals
To calculate a confidence interval, you need three key pieces of information: the sample data, the sample size, and the desired confidence level.
- Let’s consider an example where we want to estimate the mean weight of a population based on a sample of 100 individuals. For instance, the sample mean weight is 150 pounds, and the standard deviation is 20 pounds. We choose a 95% confidence level.
Step 1: Determine the critical value. In this case, for a 95% confidence level, the critical value is 1.96. This value is obtainable from statistical tables or using online calculators.
Step 2: Calculate the standard error. The standard error measures the variability of the sample mean and is calculated as the standard deviation divided by the square root of the sample size. In this case, the standard error is 20 / √100 = 2.
Step 3: Apply the formula. Using the formula for confidence intervals, we can subsequently calculate the confidence interval for the mean weight,
Confidence Interval = 150 ± (1.96 × 2) = 150 ± 3.92
- The confidence interval is, therefore (146.08, 153.92). This means that we can be 95% confident that the true population mean weight lies within this range.
- It’s important to note that confidence interval associates with a certain level of confidence. The most commonly used confidence levels are 90%, 95%, and 99%. Certainly, a higher confidence level requires a wider interval to capture the true parameter value with greater certainty.
- Furthermore, it’s worth mentioning that there are other formulas for calculating confidence intervals for different scenarios, such as when dealing with proportions, differences between means, or regression coefficients. These formulas involve specific statistical distributions, such as the t-distribution or the normal distribution, depending on the sample size and assumptions.
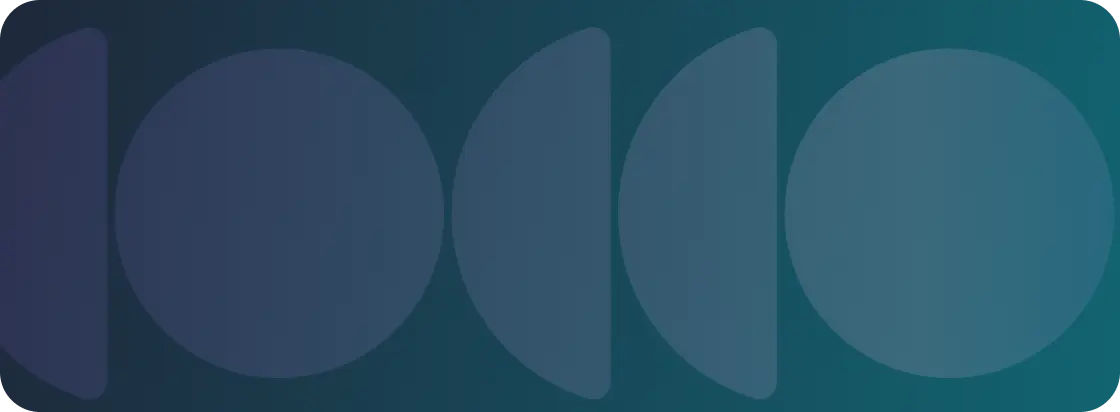
Snooze complex calculations and let SurveySparrow do it for you!
A personalized walkthrough by our experts. No strings attached!
Key Advantages of Calculating Confidence Interval
Quantifies Uncertainty
Confidence intervals provide a measure of uncertainty associated with an estimated parameter. Instead of relying solely on a point estimate, confidence intervals convey the range within which the true population parameter is likely to lie. This helps to account for sampling variability and provides a more comprehensive understanding of the data.
Reliable Inference
Confidence intervals enable researchers and analysts to draw reliable inferences about the population based on sample data. By specifying a confidence level (e.g., 95%), confidence intervals allow you to quantify the level of confidence you have in the interval’s ability to capture the true parameter value. This improves the reliability and robustness of your conclusions.
Comparison and Hypothesis Testing
Confidence intervals play a crucial role in hypothesis testing. They allow you to determine whether a parameter value falls within or outside the interval. If the null hypothesis value lies outside the confidence interval, it provides evidence to reject the null hypothesis in favor of an alternative hypothesis. Thus this aids in making informed comparisons and decisions based on statistical evidence.
Sample Size Determination
Calculating confidence intervals can help in determining the appropriate sample size for future studies or surveys. By considering the desired level of precision and confidence, you can estimate the sample size required to achieve a specific width of the confidence interval. Therefore, this helps optimize data collection efforts and ensure statistically sound results.
Communication of Findings
Confidence intervals provide a concise summary of statistical results that can be easily communicated to stakeholders, decision-makers, or the general audience. Instead of conveying complex statistical measures, confidence intervals offer a clear and intuitive representation of the estimated parameter’s uncertainty. This enhances the understanding and interpretation of statistical findings.
Comparison of Groups or Interventions
Confidence intervals allow for the comparison of different groups or interventions. When calculating confidence intervals for the difference between means or proportions, you can assess whether the intervals overlap or not. Non-overlapping intervals suggest a statistically significant difference, while overlapping intervals indicate a lack of evidence for a significant distinction.
How can SurveySparrow help you calculate the confidence interval formula effectively?
SurveySparrow, as a survey platform, can assist in collecting data that helps in calculating confidence intervals. Here’s how SurveySparrow can help,
Data Collection
SurveySparrow provides a user-friendly interface to create and distribute surveys. You can design surveys to collect the necessary data for your research or analysis. By reaching out to your target audience through various distribution methods, such as email invitations or website embedding, you can gather a representative sample of responses.
Data Analysis
Once you have collected the survey responses, SurveySparrow offers analytical features to help you make sense of the data. You can access built-in reporting and analytics tools that provide insights into response patterns, demographic information, and other relevant variables.
Statistical Calculations
Using SurveySparrow’s reporting features, you can easily calculate confidence intervals. For example, you can calculate means, proportions, and standard deviations using the collected data. In fact, these calculations serve as the basis for estimating population parameters and constructing confidence intervals.
Exporting Data
SurveySparrow allows you to export survey data in various formats, such as Excel or CSV files. Thus this flexibility enables you to analyze the data using external statistical software or tools that may provide more advanced options for calculating confidence intervals.
Integrations
SurveySparrow integrates with popular data analysis and visualization platforms, such as Google Sheets and Power BI. By integrating with these tools, you can seamlessly transfer survey data for further analysis and visualization, including the calculation of confidence intervals.
Frequently Asked Questions (FAQs)
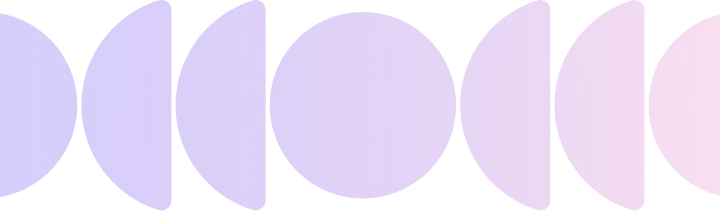