Knowledge
Correlation Analysis: Definition, Types, Examples
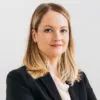
Article written by Kate Williams
Product Marketing Manager at SurveySparrow
10 min read
21 September 2024
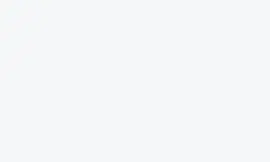
You’ve run your survey. You have collected thousands of responses. Now, it’s time to make sense of the data. To uncover the relationship between data points and highlight the big picture!
Meet Correlation Analysis!
You might remember the term from your school days; it’s a data analysis strategy essential for insights analysts.
In this blog, we will go beyond the definition and discuss how businesses in varying industries can benefit from correlation analysis with examples.
What is Correlation Analysis?
A statistical research technique, correlation analysis, is used to study how one variable affects another and how much they affect each other.
It can reveal a common thread, an underlying cause, that might seem unrelated on a surface level. You can apply correlation analysis to various kinds of data sets. Researchers use it to analyze quantitative data gathered via surveys or polls.
Sometimes, you may be able to predict how things will relate, like ice cream sales increasing in the summertime, while in others, the unsurfaced relationship will come as a complete surprise!
Well, correlation analysis of your survey data can help you understand which metrics/variables have close relationships. And how the behavior of one variable can influence one or more variables in a similar or opposite way.
Does employee engagement improve customer satisfaction? Will raising your product pricing increase your revenue?
Confused? Let me explain with...
An Example
Say you design a survey so you can know how satisfied customers are with the various aspects of your product or service. You can ask about the ease of using the product, customer support, and so on, and collect data on purchase frequency.
Through the responses you collect, you will be able to know if the levels of satisfaction have a positive correlation to the frequency at which customers make purchases with you and influence their decisions making process.
Or you can conduct a survey on social media usage awareness. Ask questions about the platforms they use and follow up with questions about their brand awareness with your company.
This data analysis can help you determine whether there is any correlation between social media usage and brand perception.
In such cases, a conversational survey is the best solution. You can create one easily with SurveySparrow's drag-and-drop builder. That's not it, send them via multiple channels and analyze the data with advanced analytics tools. All in one platform! You can try it for free!
Here's a sample template you can use! It's fully customizable so feel free to make it your own.
Customer Feedback Survey Template
Use This TemplatePopular Statistical Methods Used in Correlation Analysis
The two most widely used analytical formulae for correlation is using Pearson correlation analysis & Spearman method. Let’s look at both types and how they’re used.
Pearson correlation analysis is used to measure the strength of the linear relationship between two variables. But for it to be viable, each variable must be continuous, and the relationship must be linear. For example, analyzing the relationship between height and weight in a group of people.
Whereas the Spearman method is used when the variables are ordinal and the relationship between the variables is monotonic, which means they’re either always increasing or always decreasing. For example, Analyzing the relationship between the ranking of students in a class and their hours of study.
How to Choose The Right Type of Correlation Analysis Method
Let's take the example of a customer satisfaction survey. You've asked the participants to rate their satisfaction with your product on a scale of 1-5. Say that you have also recorded their age.
If we want to assume that the relationship between satisfaction and age is linear and both variables are normally distributed, we could use Pearson correlation.
However, if we suspect that the relationship is non-linear or that the satisfaction ratings are not normally distributed, we would use Spearman correlation.
Types of Correlation
Now that you’re familiar with the types of correlation analysis methods, let's discuss the three types of correlation that you will find:
- Positive Correlation: The variables have a positive linear relationship as the two variables increase or decrease in the same direction.
For example, Study hours and grades. Students who tend to study long hours generally score more marks. - Negative Correlation: A negative correlation is when the relationship has a negative slope, and the variables change in opposite directions
For example, study hours and errors in exams. Students who tend to study long hours make fewer errors during exams. - No Correlation: Here, the variables have no linear relationship and they behave very differently.
For example, Study hours and hair color. A person’s hair color has no influence over the study hours they put in.
Why is Correlation Analysis Important?
While marketers can use correlation analysis to study the effectiveness of ads in their marketing campaigns, technical support teams can use it to reduce the number of alerts by grouping related alerts into a single alert and filtering out the rest. The use cases are plenty. But if I am to list the top 5 benefits of correlation analysis of survey data, they would be:
Validate Trends
Verify if the trends you’ve observed are just random fluctuations or if they’re statistically significant for your business.
For example, you can use correlation analysis to compare customer satisfaction scores with other variables, such as product quality ratings, employee satisfaction, or changes in pricing, to determine whether the decline in customer satisfaction is simply a flux.
Gain Context
Correlation analysis provides a much broader perspective, and you can easily understand how survey data is related to other data you have collected.
For example, let’s take your survey data on customer satisfaction and sales numbers. The correlation analysis would help you understand the relationship between customer satisfaction and your increased revenue and uncover actionable insights on your marketing & sales strategies.
Identify Key Factors
Let go of assumptions and discover the exact factors that have the most influence on your business.
For example, imagine you have survey data on customer satisfaction, pricing, and product features. A correlation analysis might tell you which variable is strongly related to customer satisfaction, and you can start improving that influential variable to increase customer satisfaction.
Prioritize Research
From identifying the key factors that drive your business success, get insights on where to focus your research efforts more.
For example, run a correlation analysis on customer satisfaction and product features to understand where the customers are unsatisfied and where you must focus to improve.
Drive Change
Using the insights from the survey, you can make smart, data-driven changes to your product or business.
For example, if you have survey data on employee satisfaction and churn rates, with correlation analysis, you can uncover which variable is negatively correlated to churn, implement changes, and retain your employees.
Techniques for Correlation Analysis
There are different techniques you can adopt based on objective and data type, let’s talk about the three most commonly used ones here.
Correlation Coefficients
Remember Pearson’s method and Spearman’s method that we discussed earlier? The correlation coefficient can be explained as the unit of measurement used to calculate the strength & direction of the relationship between the two variables using these analysis methods and is always between -1 and 1.
Cross-Tabulation
A popular statistical tool for categorizing data, cross-tabulation involves data that are mutually exclusive from each other. It will help you make smart decisions from raw data by identifying patterns, trends, and a correlation between parameters. Get deeper insights on why you use cross-tabulation in survey analysis here!
Well, if you need a tool to make sense of your survey data, you know where to go! You can create, share, collect, and analyze data all in one platform!

Regression Analysis
When you want to study the relationship between one variable that is dependent and a series of other variables, regression analysis would be your best way forward.
How to Make Sense of Survey Data with Correlational Analysis
Key performance indicators like customer satisfaction and NPS® play a vital role in the success of your business. And, correlational analysis of your survey data will let you zero in on the exact key drivers that influence and lead these metrics for you.
From the right product placement in your retail store shelf to evaluating the success of your marketing efforts, correlation analysis will uncover hidden patterns, and unify actionable insights from your scattered survey data.
For example, a hotel chain runs a customer satisfaction survey to understand the factors influencing guest satisfaction rates.
Using correlation analysis, you might find strong positive correlations between guest satisfaction and room cleanliness and amenities. This suggests that these factors are important contributors to increase your overall customer satisfaction.
You can also create a cross-tabulation table to examine how the guest type and the length of their stay are related to overall guest satisfaction. The completed cross-tabulation analysis would help you identify & categorize guest segments that are more likely to be satisfied with their stay with you.
Regression analysis will predict customer satisfaction scores by identifying factors that affect the guest experience and help you prioritize your improvement efforts.
Examples of Correlation Analysis in Surveys Across Industries
The variables involved in correlation analysis differ based on your objective. Let’s see how you can run correlation analysis in different industries.
In E-commerce
If you take the variables 'website design' and 'conversion rates,' you can analyze the relationship between website design elements such as layout or navigation and conversion rates. Using the insights, you may optimize the website design to ensure maximum conversions.
You can also explore the correlation between customer experience factors such as delivery speed or returns policy and repeat purchase behavior. This insight can help you identify critical areas for improvement to increase customer loyalty.
In Retail
Here, you can take the variables like your store's layout and sales numbers. You can effectively analyze the correlation between your aisle design and the number of sales you have closed to uncover suggestions to optimize your store's design & increase sales.
In SaaS
Applying correlation analysis techniques will help you understand the relationship between your product features and customer churn. It would tell you if it's the performance of a critical feature that is causing your customers to leave you.
In Market Research
One of the most popular statistical methods in market research, correlation analysis, can be used to learn the relationship between market trends and consumer behavior. You can also use the same method to improve survey engagement by correlating it with another variable, like survey questions.
In Healthcare
With correlation analysis, explore if there is a relationship between patient satisfaction and healthcare outcomes to identify key factors that can contribute to positive outcomes. You can also consider variables like doctor satisfaction and patient satisfaction ratings to uncover factors that deliver positive patient experiences.
The Conclusion
Correlation analysis helps businesses generate actionable insights on customer preferences, employee satisfaction, product performance, overall business health, and more. You can either calculate and run these analyses using Excel or a calculator.
However, the most convenient, easy-to-generate-insights way would be choosing a survey analytics tool like SurveySparrow to do all the grunt work for you. And bother you only with business insights that can make decision-making easier, improve customer experiences, increase employee engagement, and drive business growth.
Start 14 Days free trial
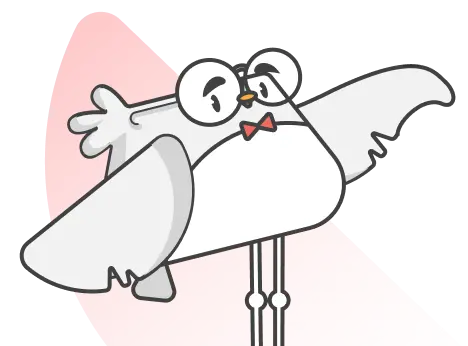

Kate Williams
Excels in empowering visionary companies through storytelling and strategic go-to-market planning. With extensive experience in product marketing and customer experience management, she is an accomplished author, podcast host, and mentor, sharing her expertise across diverse platforms and audiences.
Related Articles
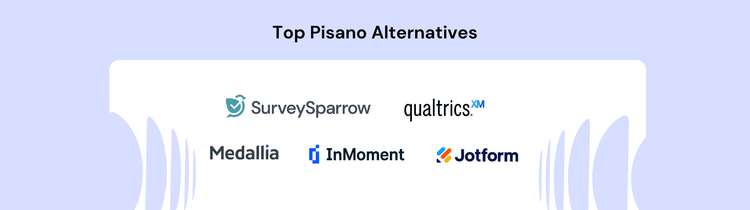
Alternative
Top Pisano Competitors and Alternatives in 2024
7 MINUTES
16 September 2024
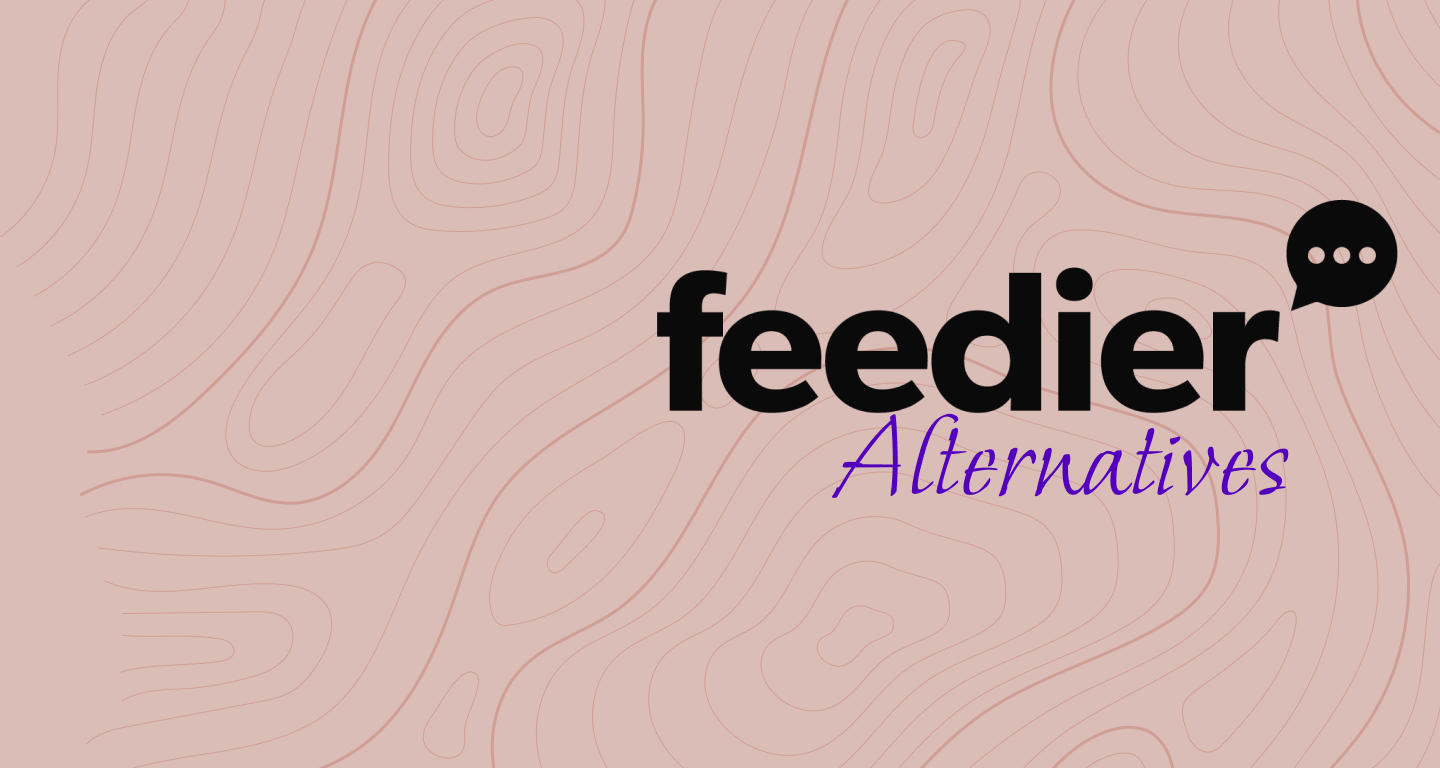
Alternative
8 Feedier Alternatives for Managing Customers Effectively
13 MINUTES
30 May 2023
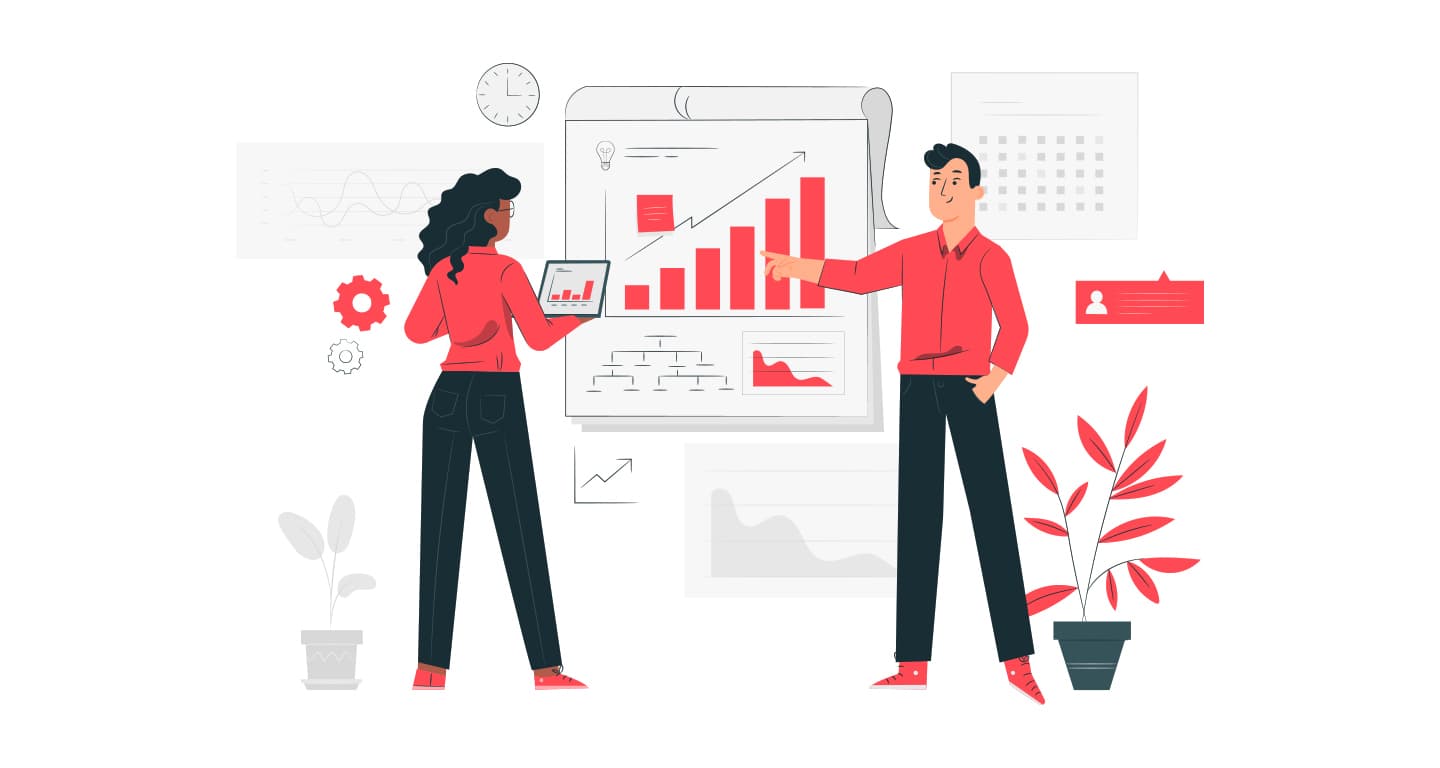
Best Of
6 Metrics Chief Customer Officers Care About & How to Impact Them
15 MINUTES
17 March 2021
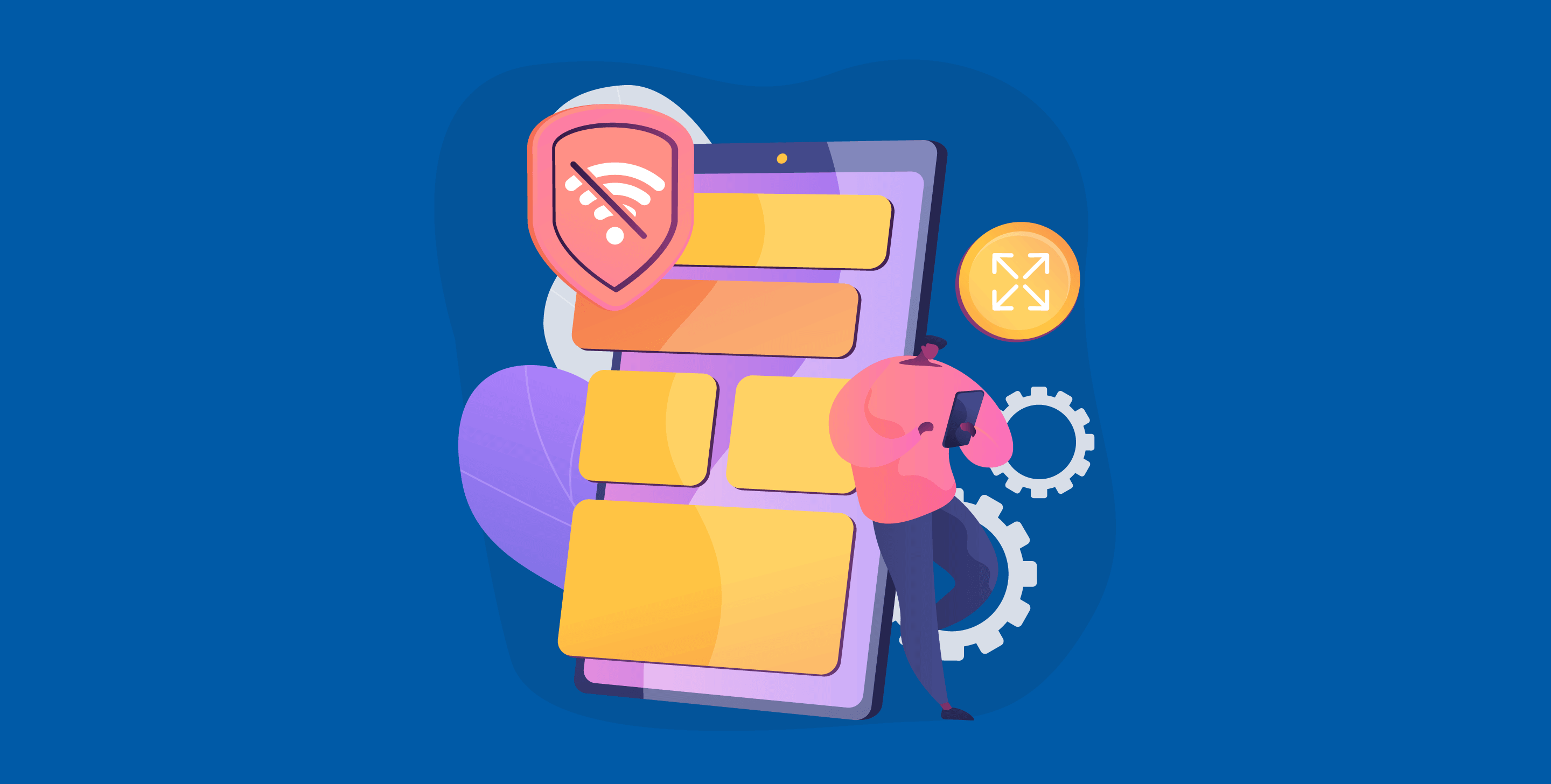
Survey Tips
Offline Form is the New Talk of the Town, and Rightly So!
9 MINUTES
27 September 2018